Adapting activity recognition to a person with Multi-Classifier Adaptive Training.
JAISE(2015)
摘要
Activity-recognition classifiers, which label an activity based on sensor data, have decreased classification accuracy when used in the real world with a particular person. To improve the classifier, a Multi-Classifier Adaptive-Training algorithm MCAT is proposed. The MCAT adapts activity recognition classifier to a particular person by using four classifiers to utilise unlabelled data. The general classifier is trained on the labelled data available before deployment and retrieved in the controlled environment. The specific classifier is trained on a limited amount of labelled data belonging to the new person in the new environment. A domain-independent meta-classifier decides whether to classify a new instance with the general or specific classifier. The final, second meta-classifier decides whether to include the new instance into the training set of the general classifier. The general classifier is periodically retrained, gradually adapting to the new person in the new environment. The adaptation results were evaluated for statistical significance. Results showed that the MCAT outperforms competing approaches and significantly increases the initial activity-recognition classifier classification accuracy.
更多查看译文
关键词
activity recognition,adaptation,semi supervised learning
AI 理解论文
溯源树
样例
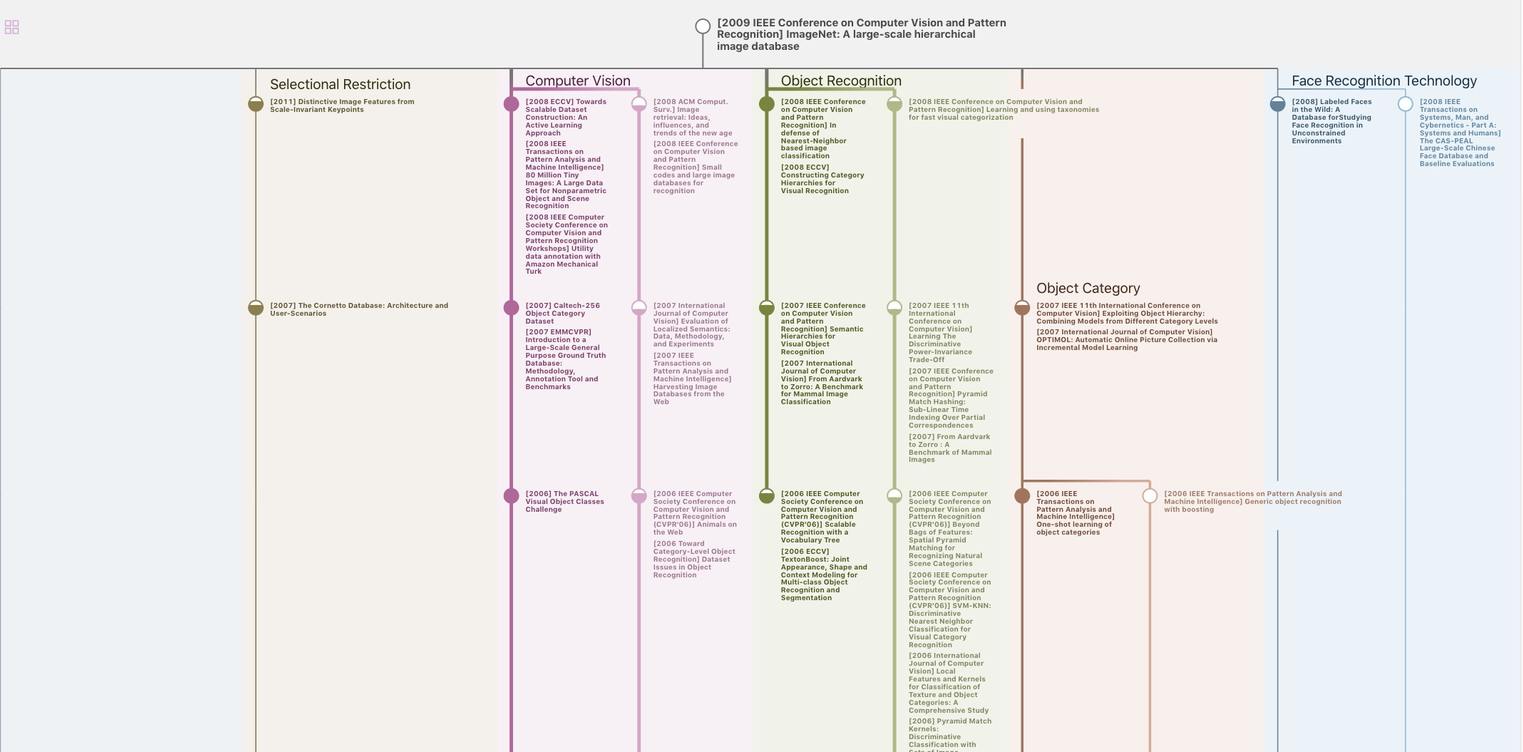
生成溯源树,研究论文发展脉络
Chat Paper
正在生成论文摘要