Genetic algorithm based wrapper feature selection on hybrid prediction model for analysis of high dimensional data
Industrial and Information Systems(2014)
关键词
data analysis,data mining,diseases,feature selection,genetic algorithms,medical computing,pattern classification,pattern clustering,gwhpm,hpm,classifier model,data mining concept,disease prediction,genetic algorithm based wrapper feature selection,high dimensional data analysis,hybrid prediction model,k-means clustering technique,medical field,classifier,clustering,feature selection (fs),genetic algorithm based wrapper feature selection hybrid prediction model (gwhpm),hybrid prediction model (hpm),diabetes,support vector machines,predictive models,accuracy
AI 理解论文
溯源树
样例
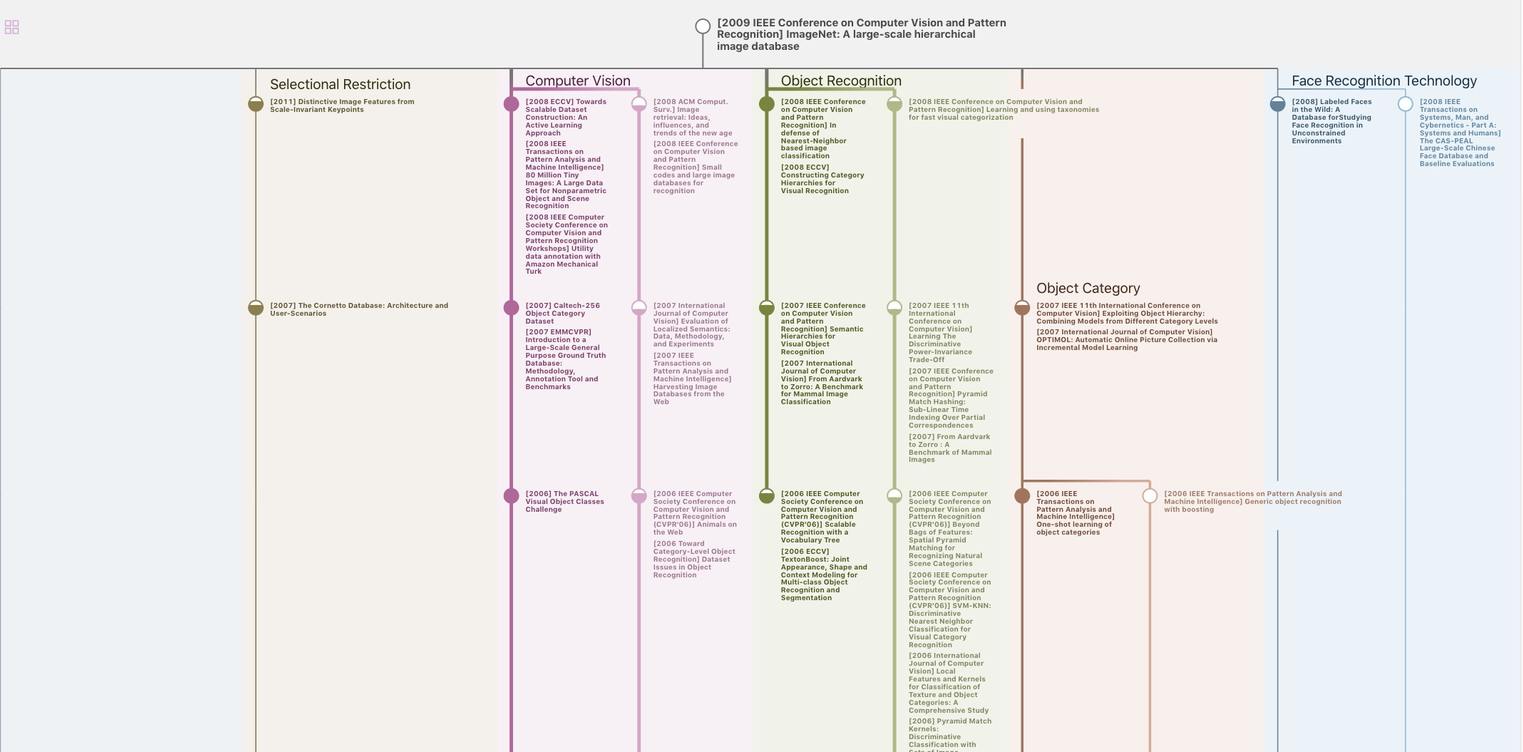
生成溯源树,研究论文发展脉络
Chat Paper
正在生成论文摘要