Load forecasting under data corruption based on anomaly detection and combined robust regression
INTERNATIONAL TRANSACTIONS ON ELECTRICAL ENERGY SYSTEMS(2020)
摘要
With the expansion of information technology, cybersecurity vulnerability issues caused by data integrity attacks are becoming a major threat for the modern power industries. Electricity load forecasting under data integrity attacks has attracted great concern for power industries in recent years. However, most conventional load forecasting models lack the capacity to produce accurate results against data corruption. A comprehensive framework for robust load forecasting in the presence of imperfect datasets has not been discussed in the existing literature. This paper introduces a robust load forecasting framework to obtain higher prediction accuracy given different levels of data corruption. Firstly, the corrupted data is corrected by the anomaly detection method based on denoising variational autoencoder. Then, the combined robust forecasting model is established to integrate the independent advantages of different forecasters. The numerical results of case studies on the dataset of Global Energy Forecasting Competition 2014 demonstrate the outstanding effectiveness of the proposed method over other competing methods. Under different degrees of data corruption, the average mean absolute percentage error (MAPE) of the proposed model is 2.37 and the average root mean square error (RMSE) of the proposed model is 113.35, which represents the best performance among all competing models.
更多查看译文
关键词
anomaly detection,data integrity attack,forecast combination,load forecasting,robust regression
AI 理解论文
溯源树
样例
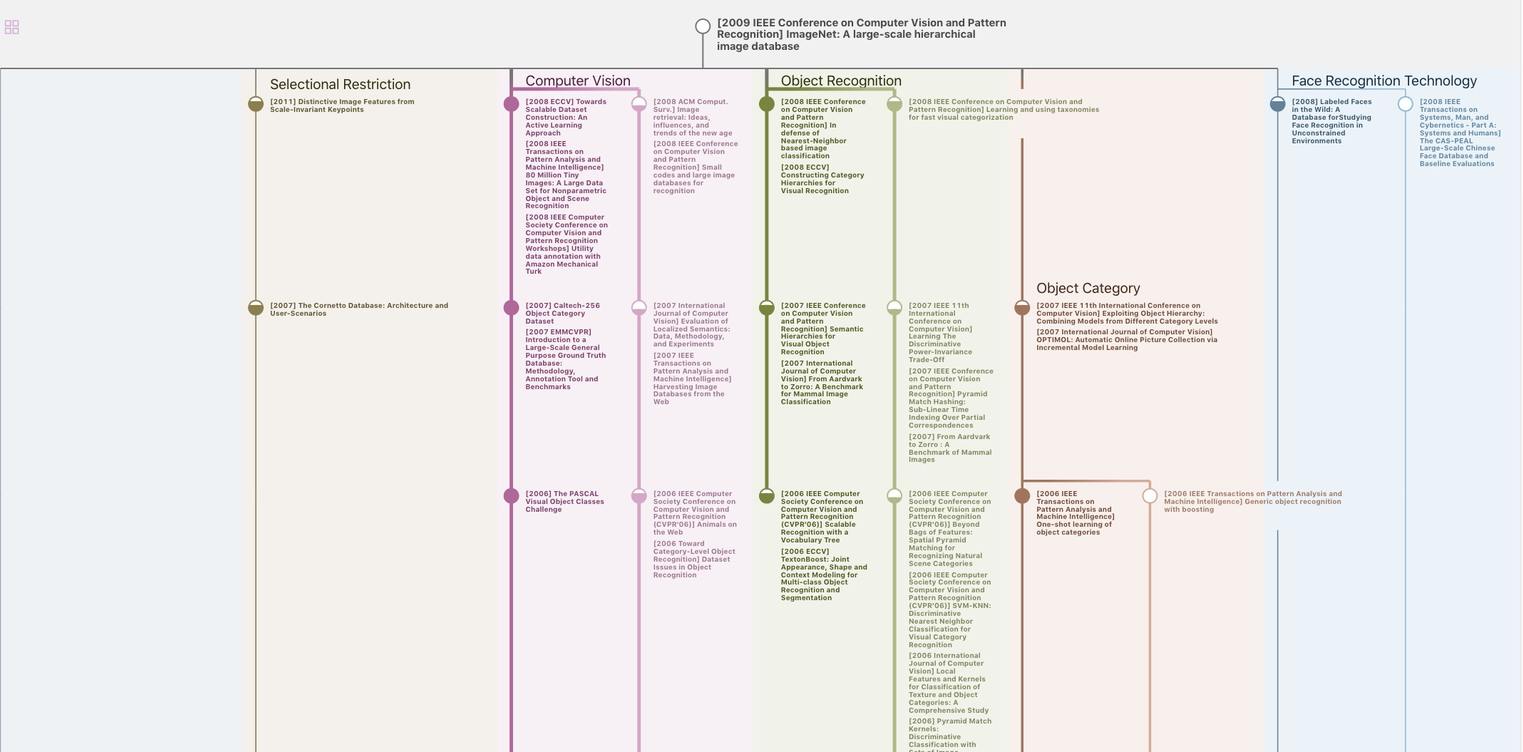
生成溯源树,研究论文发展脉络
Chat Paper
正在生成论文摘要