HGG-41. STRUCTURAL VARIANT DRIVERS IN PEDIATRIC HIGH-GRADE GLIOMA
Neuro-oncology(2020)
摘要
Abstract BACKGROUND Driver single nucleotide variants (SNV) and somatic copy number aberrations (SCNA) of pediatric high-grade glioma (pHGGs), including Diffuse Midline Gliomas (DMGs) are characterized. However, structural variants (SVs) in pHGGs and the mechanisms through which they contribute to glioma formation have not been systematically analyzed genome-wide. METHODS Using SvABA for SVs as well as the latest pipelines for SCNAs and SNVs we analyzed whole-genome sequencing from 174 patients. This includes 60 previously unpublished samples, 43 of which are DMGs. Signature analysis allowed us to define pHGG groups with shared SV characteristics. Significantly recurring SV breakpoints and juxtapositions were identified with algorithms we recently developed and the findings were correlated with RNAseq and H3K27ac ChIPseq. RESULTS The SV characteristics in pHGG showed three groups defined by either complex, intermediate or simple signature activities. These associated with distinct combinations of known driver oncogenes. Our statistical analysis revealed recurring SVs in the topologically associating domains of MYCN, MYC, EGFR, PDGFRA & MET. These correlated with increased mRNA expression and amplification of H3K27ac peaks. Complex recurring amplifications showed characteristics of extrachromosomal amplicons and were enriched in coding SVs splitting protein regulatory from effector domains. Integrative analysis of all SCNAs, SNVs & SVs revealed patterns of characteristic combinations between potential drivers and signatures. This included two distinct groups of H3K27M DMGs with either complex or simple signatures and different combinations of associated variants. CONCLUSION Recurrent SVs associate with signatures shaped by an underlying process, which can lead to distinct mechanisms to activate the same oncogene.
更多查看译文
AI 理解论文
溯源树
样例
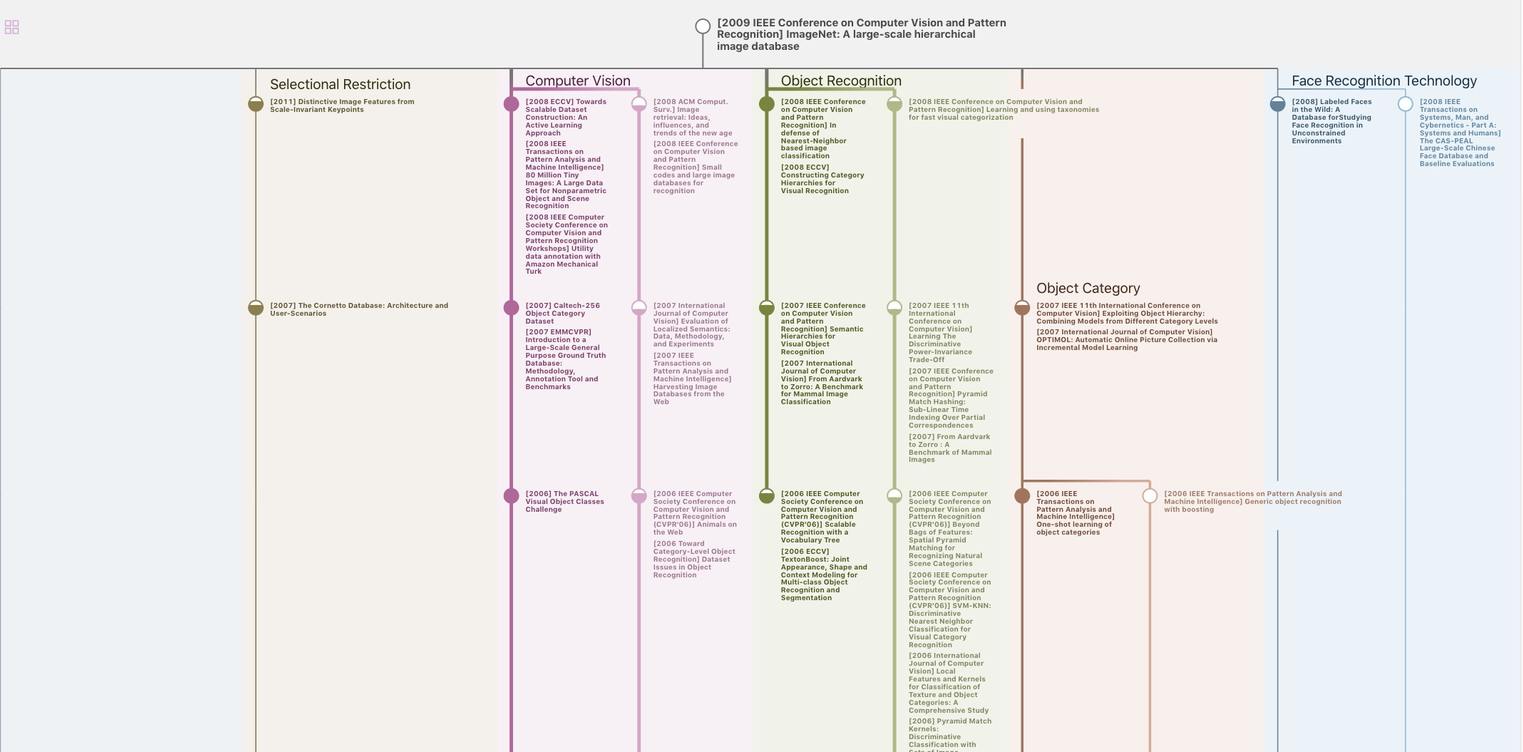
生成溯源树,研究论文发展脉络
Chat Paper
正在生成论文摘要