Extracting and Characterizing I/O Behavior of HPC Workloads.
2022 IEEE International Conference on Cluster Computing (CLUSTER)(2022)
摘要
System administrators set default storage-system configuration parameters with the goal of providing high per-formance for their system's I/O workloads. However, this gener-alized configuration can lead to suboptimal I/O performance for individual workloads. Users can provide parameter settings to the storage system to obtain better performance for individual applications, but it can be very challenging to determine which parameters to set and to what values. This problem is further ex-acerbated by the increased complexity of modern storage systems. In this work, we move towards solving this problem by providing a systematic categorization of workload-related information that users or middleware libraries can pass to the storage system to optimize I/O performance for specific workloads. We study applications and workflows from different scientific domains to cover a broad range of HPC use cases. Through our categorization, we find that a) workload features differ based on the hardware, software, and data components involved in the execution of workloads and b) multiple workload features together drive I/O optimizations. The methodology proposed in this work optimizes complex scientific workloads by 2.2 x−8 x, using workload-aware I/Ooptimizations. Using the proposed methodology, users can pragmatically characterize their workload, and this characterization can assist the storage system in configuring itself to optimize I/Operformance for individual workloads in HPC systems.
更多查看译文
关键词
I/O characterization,workflow I/O,Montage,Simulation,LBANN,Workload-aware,Pegasus
AI 理解论文
溯源树
样例
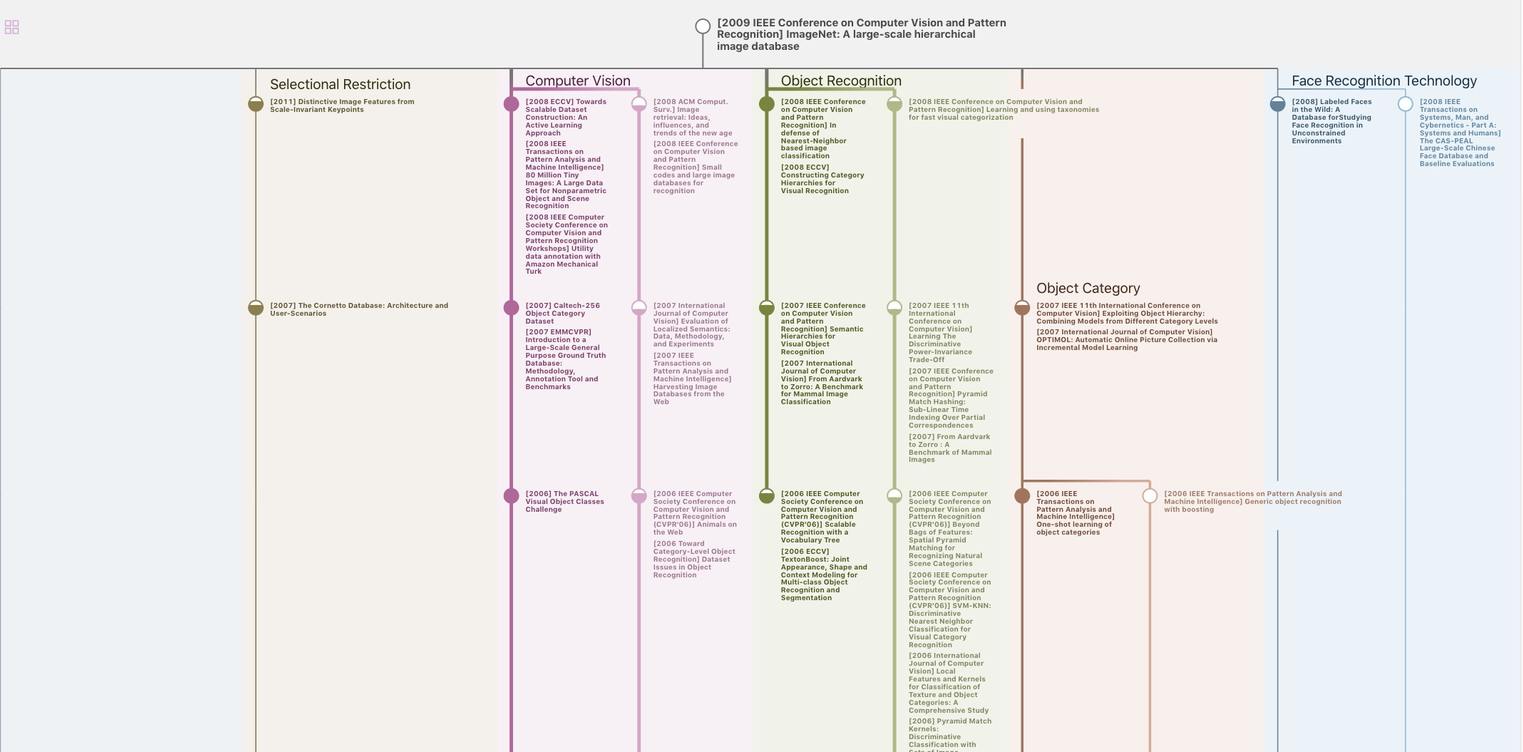
生成溯源树,研究论文发展脉络
Chat Paper
正在生成论文摘要