Representation Deficiency in Masked Language Modeling.
arXiv (Cornell University)(2023)
摘要
Masked Language Modeling (MLM) has been one of the most prominent approaches for pretraining bidirectional text encoders due to its simplicity and effectiveness. One notable concern about MLM is that the special $\texttt{[MASK]}$ symbol causes a discrepancy between pretraining data and downstream data as it is present only in pretraining but not in fine-tuning. In this work, we offer a new perspective on the consequence of such a discrepancy: We demonstrate empirically and theoretically that MLM pretraining allocates some model dimensions exclusively for representing $\texttt{[MASK]}$ tokens, resulting in a representation deficiency for real tokens and limiting the pretrained model's expressiveness when it is adapted to downstream data without $\texttt{[MASK]}$ tokens. Motivated by the identified issue, we propose MAE-LM, which pretrains the Masked Autoencoder architecture with MLM where $\texttt{[MASK]}$ tokens are excluded from the encoder. Empirically, we show that MAE-LM improves the utilization of model dimensions for real token representations, and MAE-LM consistently outperforms MLM-pretrained models on the GLUE and SQuAD benchmarks.
更多查看译文
关键词
Masked Language Modeling
AI 理解论文
溯源树
样例
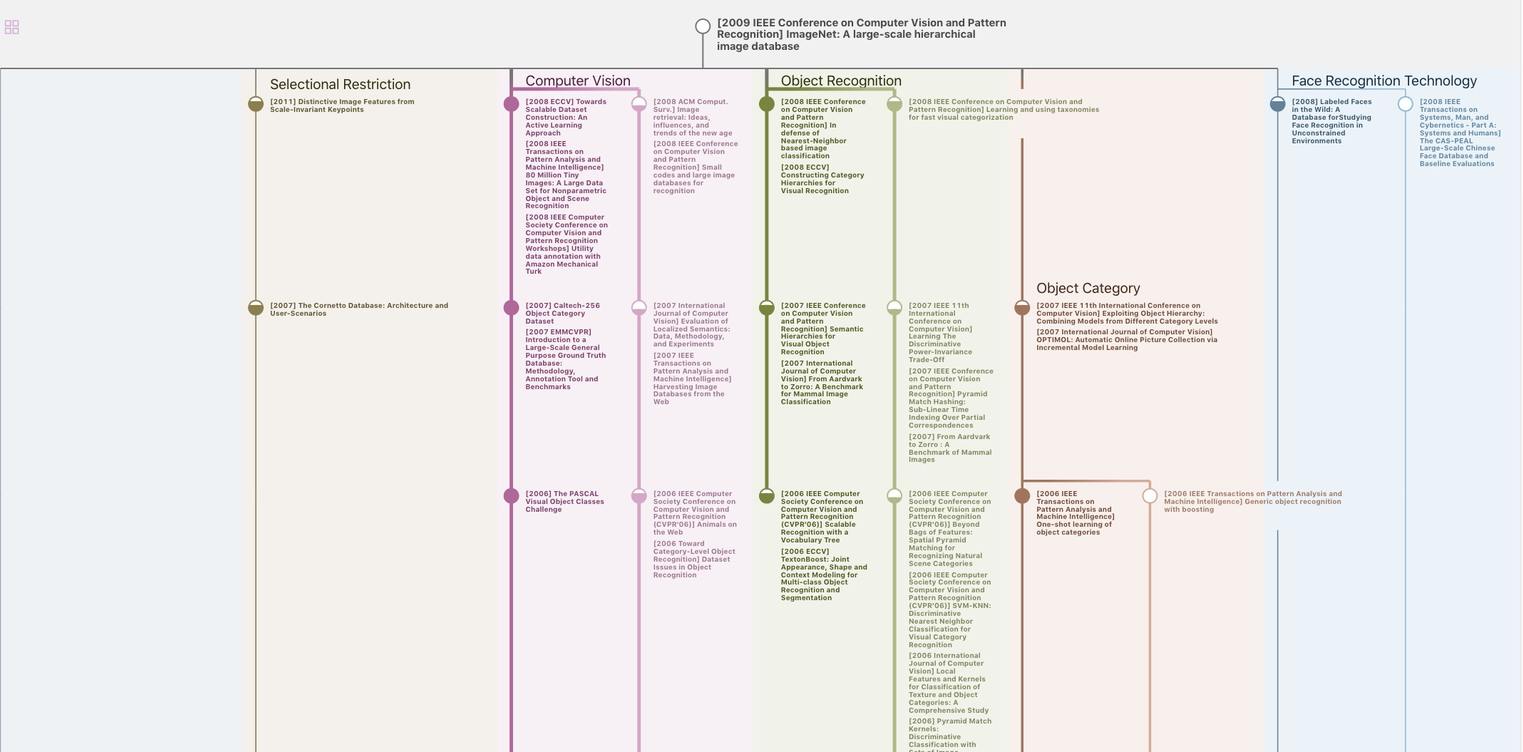
生成溯源树,研究论文发展脉络
Chat Paper
正在生成论文摘要