Unintended Consequences of Trying to Help: Augmented Target Recognition Cues Bias Perception
Journal of vision(2022)
摘要
Rapid advances in computer vision mean that artificial intelligence-aided systems may be able to provide helpful suggestions for a variety of complex visual tasks. One example of this approach is Augmented Target Recognition (ATR) where Soldiers in the field may be aided in a threat detection task by a system that indicates potential threats. It is currently unclear how ATR systems may bias performance in instances where the system is incorrect. This has important implications for the eventual adoption of such systems. In this study, participants were tasked to rapidly identify 2-5 armed individuals in 100 generated images. Participants completed the task with aid from a Liberal system (i.e., more false alarms, fewer misses), a Conservative system (i.e., fewer false alarms, more misses), or no additional information. We compared target detection performance, operationalized as d’, for both ATR conditions relative to the no ATR condition. Both ATR systems improved the speed of threat detection, but d’ improvement was negligible. The system induced sizable bias which varied depending on the criterion of the ATR system. Participants with Liberal ATR were much more likely to miss targets that were missed by the ATR. Meanwhile, participants with the Conservative ATR were much more likely to identify incorrectly marked, unarmed people (false-alarms) as threats. These results suggest that ATR cues induce automation bias, which may be due to attentional capture upon first viewing the scene with ATR markings. In a second experiment, we created an ‘interactive’ ATR (iATR) where the classification of target was provided to the user after they queried a target. This approach greatly reduced the evidence of bias induced by the ATR markings in Experiment 1, but did not result in a net benefit in terms of target detection.
更多查看译文
AI 理解论文
溯源树
样例
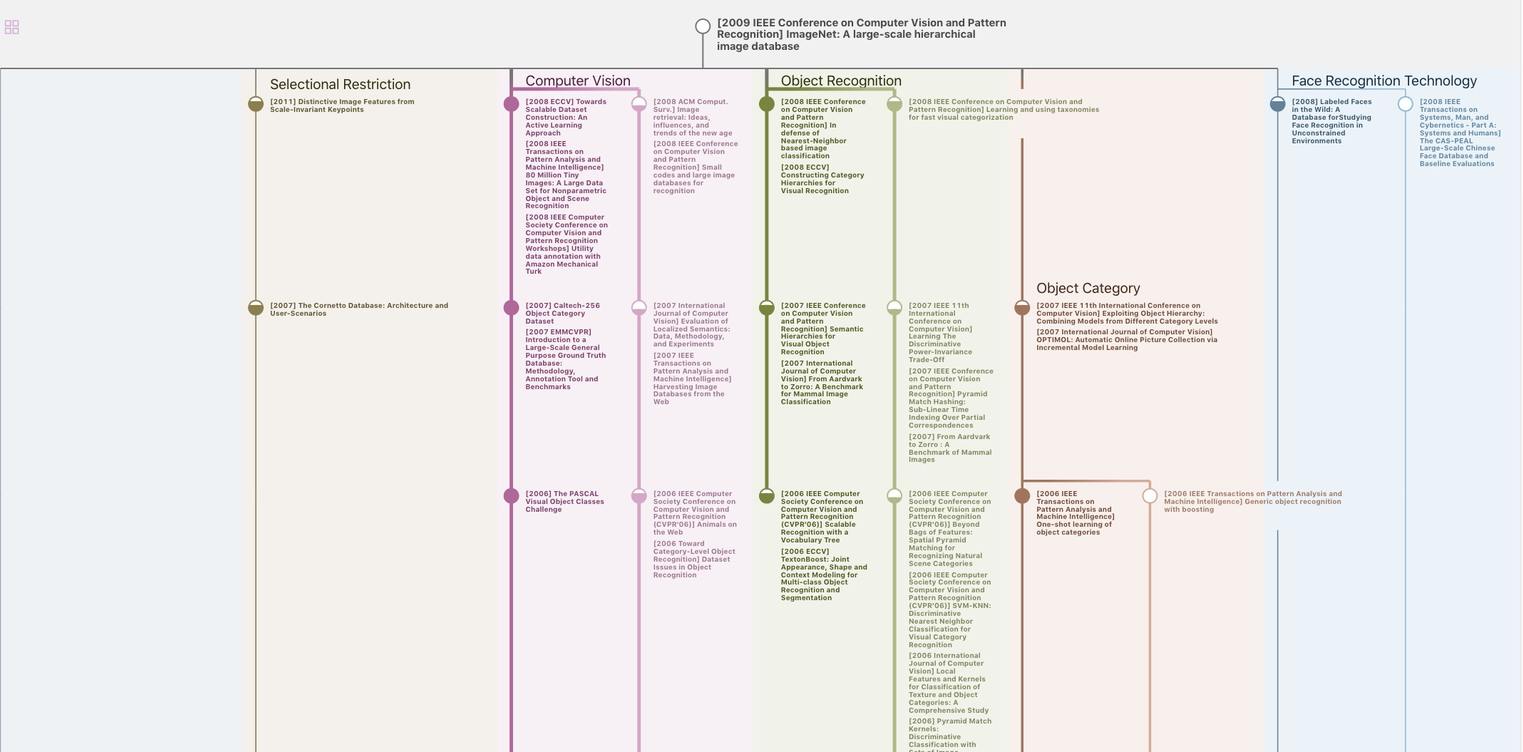
生成溯源树,研究论文发展脉络
Chat Paper
正在生成论文摘要