A Machine Learning Approach to Identify the Air Shower Cores for the GRAPES-3 Experiment
Proceedings of The 6th International Workshop on Deep Learning in Computational Physics — PoS(DLCP2022)(2022)
摘要
The GRAPES-3 experiment located in Ooty consists of a dense array of 400 plastic scintillator detectors spread over an area of 25,000 $m^2$ and a large area (560 $m^2$) tracking muon telescope. Everyday, the array records about 3 million showers in the energy range of 1 TeV - 10 PeV induced by the interaction of primary cosmic rays in the atmosphere. These showers are reconstructed in order to find several shower parameters such as shower core, size, and age. High-energy showers landing far away from the array often trigger the array and are found to have their reconstructed cores within the array even though their true cores lie outside, due to reconstruction of partial information. These showers contaminate and lead to an inaccurate measurement of energy spectrum and composition. Such showers are removed by applying quality cuts on various shower parameters, manually as well as with machine learning approach. This work describes the improvements achieved in removal of such contaminated showers with the help of machine learning.
更多查看译文
AI 理解论文
溯源树
样例
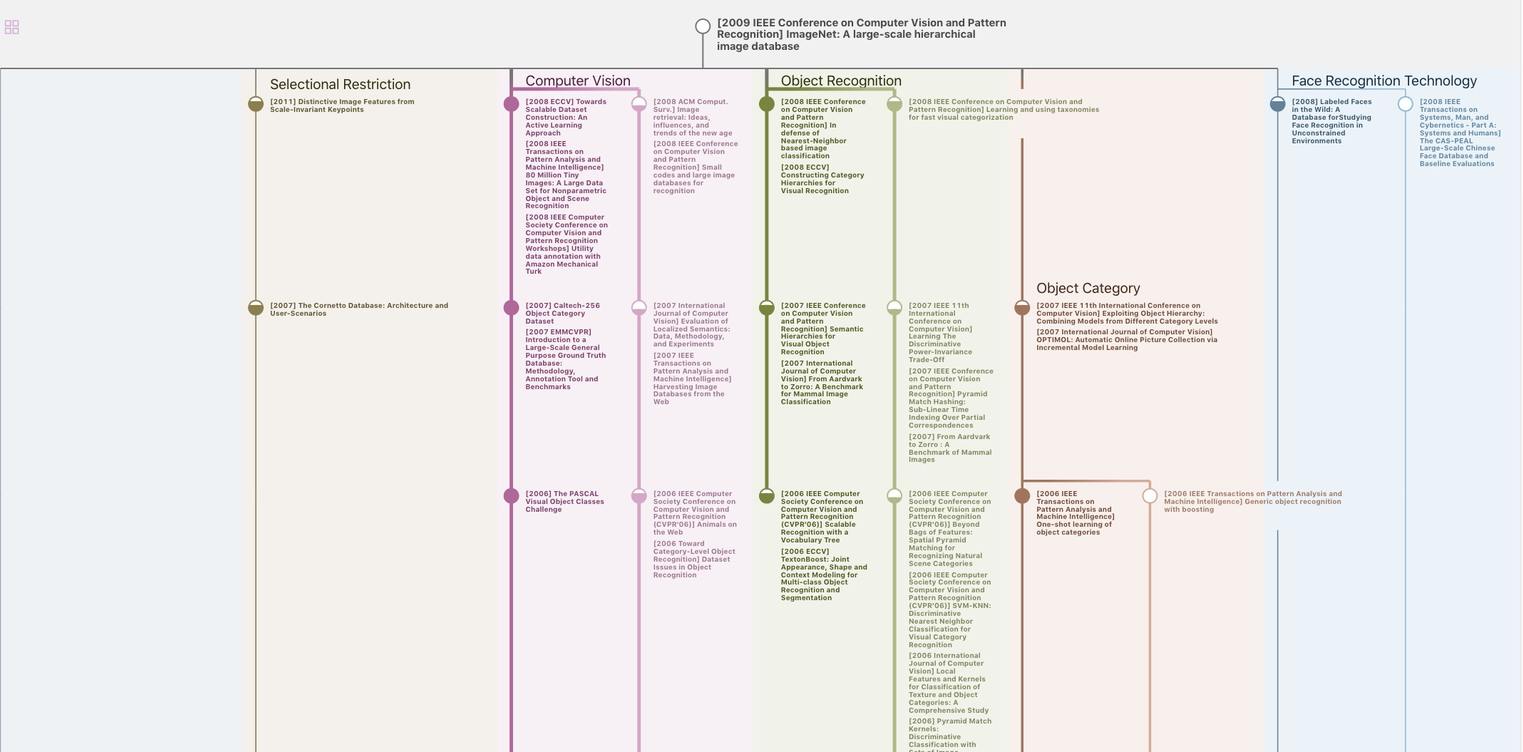
生成溯源树,研究论文发展脉络
Chat Paper
正在生成论文摘要