MFTCoder: Boosting Code LLMs with Multitask Fine-Tuning
KDD 2024(2024)
摘要
Code LLMs have emerged as a specialized research field, with remarkable studies dedicated to enhancing model's coding capabilities through fine-tuning on pre-trained models. Previous fine-tuning approaches were typically tailored to specific downstream tasks or scenarios, which meant separate fine-tuning for each task, requiring extensive training resources and posing challenges in terms of deployment and maintenance. Furthermore, these approaches failed to leverage the inherent interconnectedness among different code-related tasks. To overcome these limitations, we present a multi-task fine-tuning framework, MFTCoder, that enables simultaneous and parallel fine-tuning on multiple tasks. By incorporating various loss functions, we effectively address common challenges in multi-task learning, such as data imbalance, varying difficulty levels, and inconsistent convergence speeds. Extensive experiments have conclusively demonstrated that our multi-task fine-tuning approach outperforms both individual fine-tuning on single tasks and fine-tuning on a mixed ensemble of tasks. Moreover, MFTCoder offers efficient training capabilities, including efficient data tokenization modes and parameter efficient fine-tuning (PEFT) techniques, resulting in significantly improved speed compared to traditional fine-tuning methods. MFTCoder seamlessly integrates with several mainstream open-source LLMs, such as CodeLLama and Qwen. Our MFTCoder fine-tuned CodeFuse-DeepSeek-33B claimed the top spot on the Big Code Models Leaderboard ranked by WinRate as of January 30, 2024. MFTCoder is open-sourced at https://github.com/codefuse-ai/MFTCOder
更多查看译文
关键词
Multitask Fine-Tuning,Code LLMs,Pre-trained Models,Efficient Training,Parameter Efficient Fine-Tuning
AI 理解论文
溯源树
样例
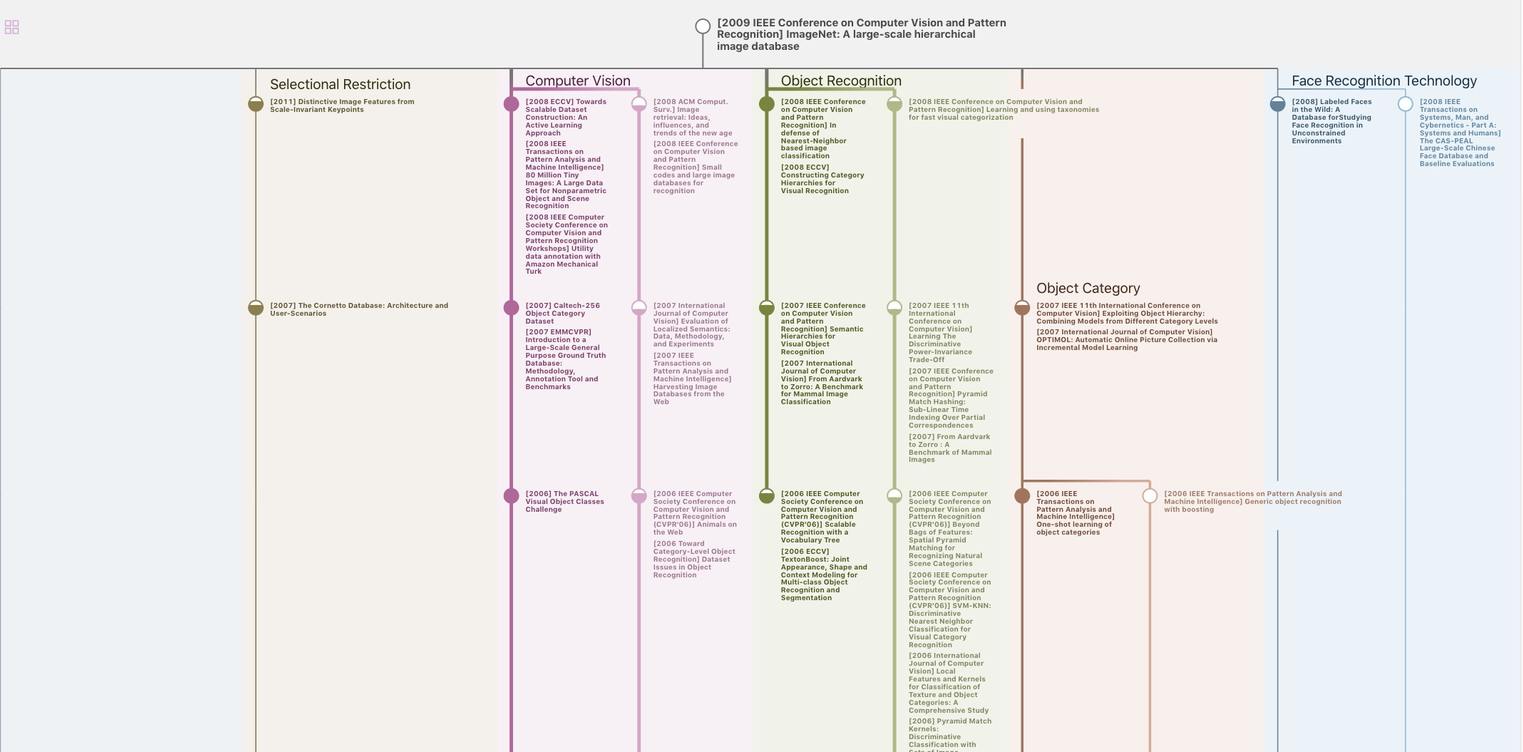
生成溯源树,研究论文发展脉络
Chat Paper
正在生成论文摘要