RadImageGAN -- A Multi-modal Dataset-Scale Generative AI for Medical Imaging
CoRR(2023)
摘要
Deep learning in medical imaging often requires large-scale, high-quality
data or initiation with suitably pre-trained weights. However, medical datasets
are limited by data availability, domain-specific knowledge, and privacy
concerns, and the creation of large and diverse radiologic databases like
RadImageNet is highly resource-intensive. To address these limitations, we
introduce RadImageGAN, the first multi-modal radiologic data generator, which
was developed by training StyleGAN-XL on the real RadImageNet dataset of
102,774 patients. RadImageGAN can generate high-resolution synthetic medical
imaging datasets across 12 anatomical regions and 130 pathological classes in 3
modalities. Furthermore, we demonstrate that RadImageGAN generators can be
utilized with BigDatasetGAN to generate multi-class pixel-wise annotated paired
synthetic images and masks for diverse downstream segmentation tasks with
minimal manual annotation. We showed that using synthetic auto-labeled data
from RadImageGAN can significantly improve performance on four diverse
downstream segmentation datasets by augmenting real training data and/or
developing pre-trained weights for fine-tuning. This shows that RadImageGAN
combined with BigDatasetGAN can improve model performance and address data
scarcity while reducing the resources needed for annotations for segmentation
tasks.
更多查看译文
AI 理解论文
溯源树
样例
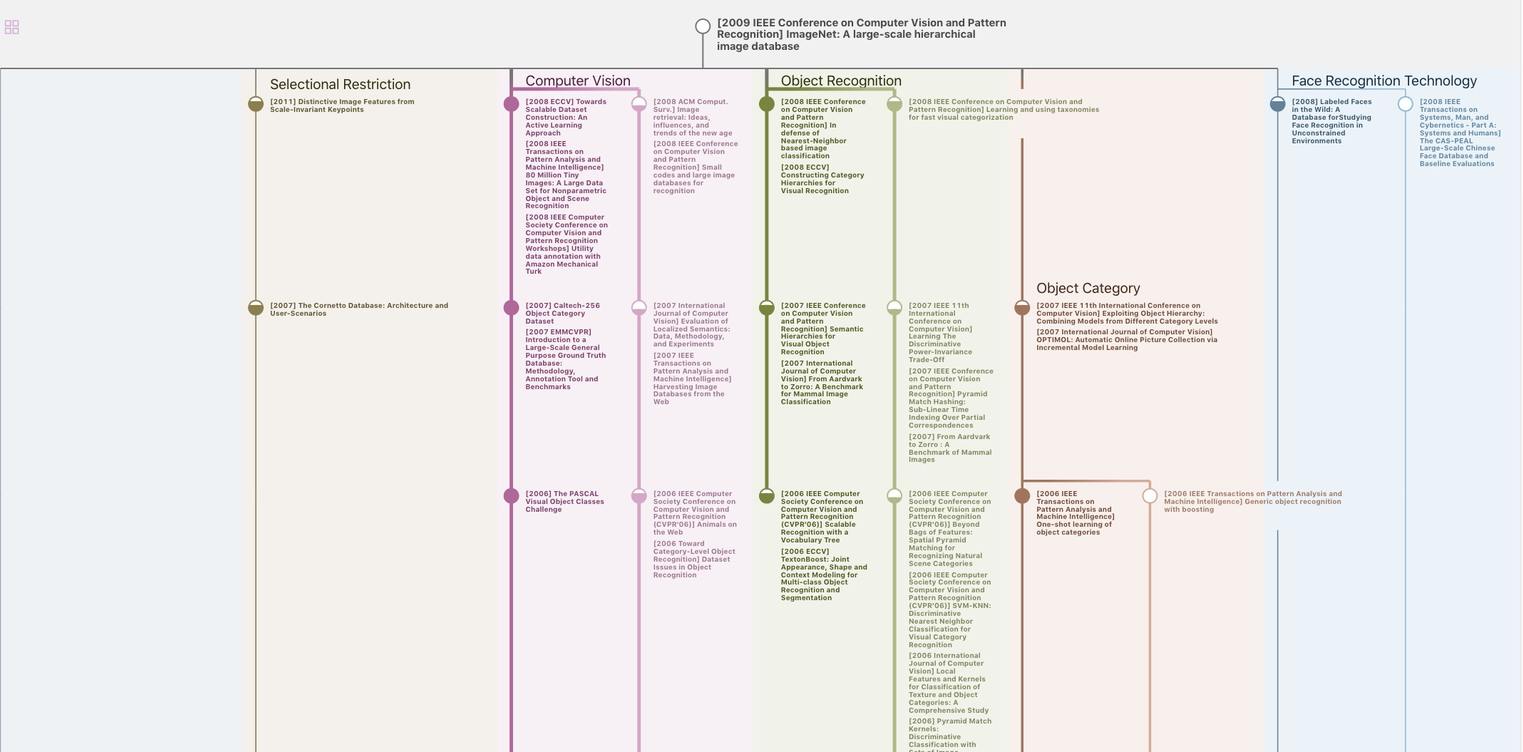
生成溯源树,研究论文发展脉络
Chat Paper
正在生成论文摘要