Improving Zero-Shot Semantic Segmentation Using Dynamic Kernels
International Conference on Digital Image Computing Techniques and Applications(2023)
摘要
Zero-shot Semantic Segmentation (ZS3) is a challenging task that segments objects belonging to classes that are completely unseen during training. An established and intuitive approach is to formulate ZS3 as a combination of two subtasks where, at first, mask proposals are generated and then each pixel in those regions is assigned a class label. Most of the existing works struggle to generate masks with high generalization capability, which results in significant underperformance in unseen classes. In this connection, we propose the use of ‘Dynamic Kernels’ to help a ZS3 model better ‘understand’ the objects in the training phase by taking advantage of their inherent inductive biases to generate better mask proposals. They act as specialized agents that are updated based on their corresponding contents from the seen classes and then utilize that knowledge to understand unseen objects. The proposed pipeline also leverages the Contrastive Language-Image Pre-Training (CLIP) architecture to perform segment classification which further improves the generalization performance by exploiting its cross-modal training. Dynamic kernels go hand-in-hand with CLIP since it is able to process the granularity of CLIP from image level to pixel level resulting in performance improvement for both the seen and unseen classes. Our method, ‘Zero-Shot dynamic Kernel Network’ (ZSK-Net), outperforms the previous works by achieving +6.4h IoU on the Pascal VOC dataset. It also achieves state-of-the-art result on the COCO-Stuff dataset by +0.9h IoU on a single prompt setting.
更多查看译文
关键词
Semantic Segmentation,Zero-Shot Learning,Vision-Language Pre-Training,CLIP
AI 理解论文
溯源树
样例
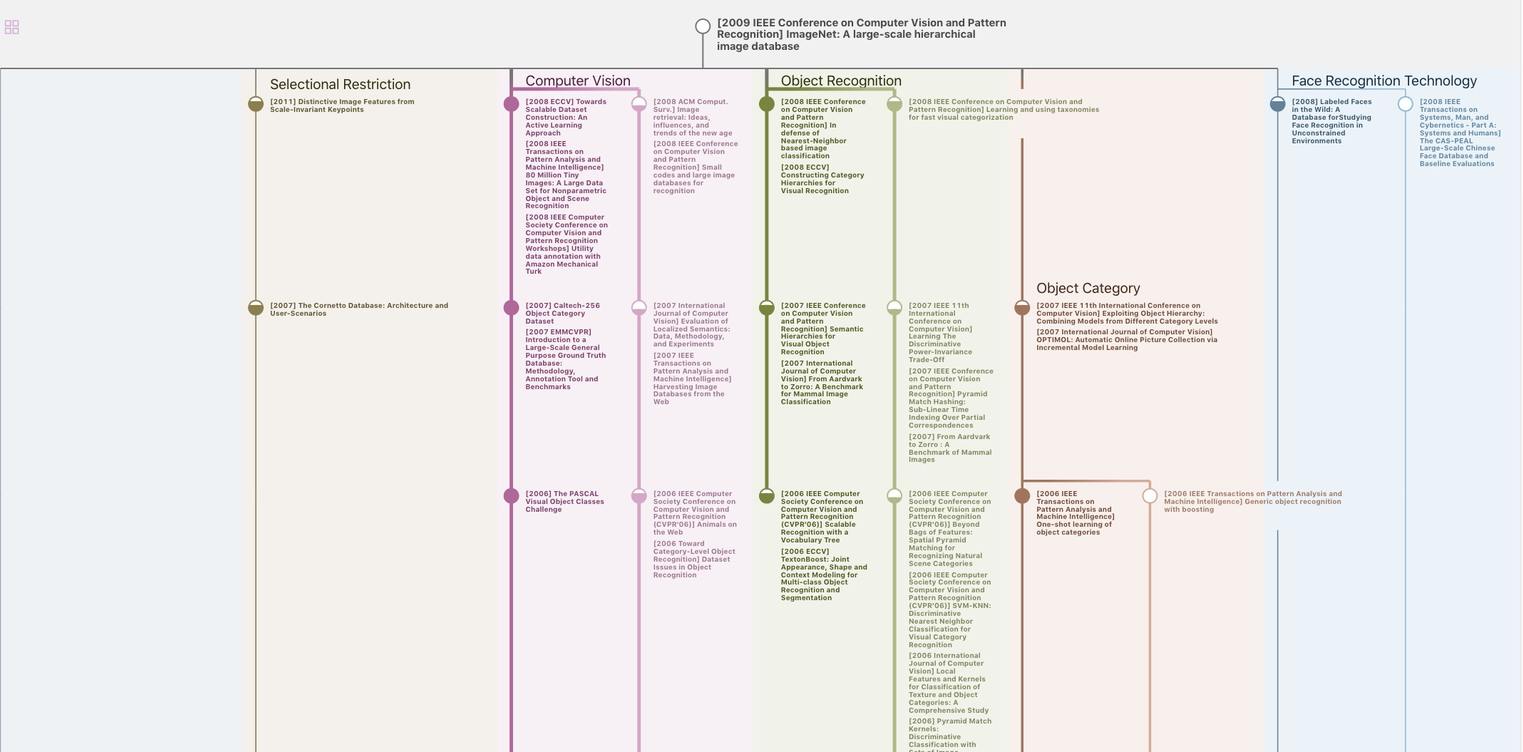
生成溯源树,研究论文发展脉络
Chat Paper
正在生成论文摘要