Generalized Preference Optimization: A Unified Approach to Offline Alignment
ICML(2024)
摘要
Offline preference optimization allows fine-tuning large models directly fromoffline data, and has proved effective in recent alignment practices. Wepropose generalized preference optimization (GPO), a family of offline lossesparameterized by a general class of convex functions. GPO enables a unifiedview over preference optimization, encompassing existing algorithms such asDPO, IPO and SLiC as special cases, while naturally introducing new variants.The GPO framework also sheds light on how offline algorithms enforceregularization, through the design of the convex function that defines theloss. Our analysis and experiments reveal the connections and subtledifferences between the offline regularization and the KL divergenceregularization intended by the canonical RLHF formulation. In all, our resultspresent new algorithmic toolkits and empirical insights to alignmentpractitioners.
更多查看译文
关键词
Constraint Optimization,Algorithm Selection,Distributed Algorithms,Group Decision Making
AI 理解论文
溯源树
样例
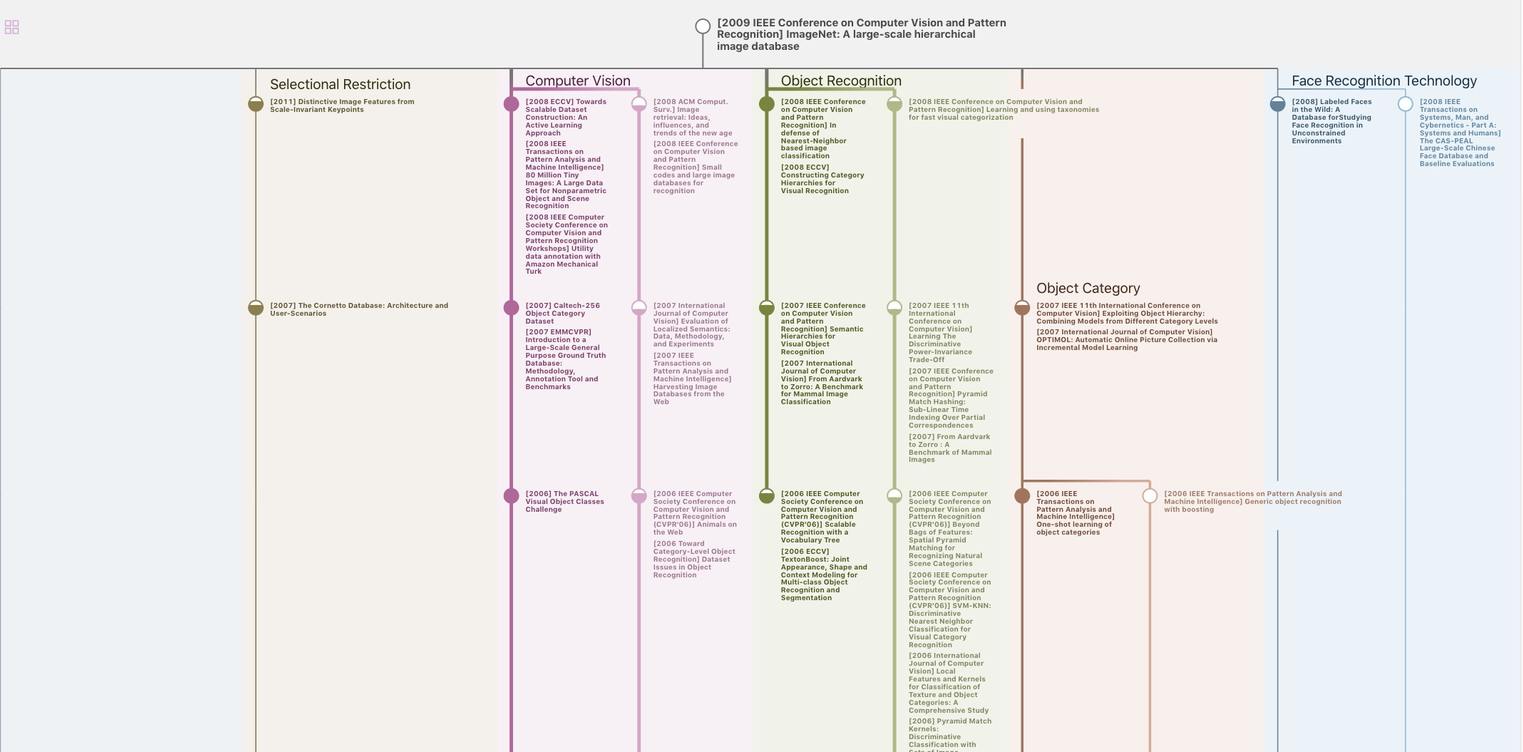
生成溯源树,研究论文发展脉络
Chat Paper
正在生成论文摘要