Unsupervised Sign Language Translation and Generation
Findings of the Association for Computational Linguistics ACL 2024(2024)
摘要
Motivated by the success of unsupervised neural machine translation (UNMT),we introduce an unsupervised sign language translation and generation network(USLNet), which learns from abundant single-modality (text and video) datawithout parallel sign language data. USLNet comprises two main components:single-modality reconstruction modules (text and video) that rebuild the inputfrom its noisy version in the same modality and cross-modality back-translationmodules (text-video-text and video-text-video) that reconstruct the input fromits noisy version in the different modality using back-translationprocedure.Unlike the single-modality back-translation procedure in text-basedUNMT, USLNet faces the cross-modality discrepancy in feature representation, inwhich the length and the feature dimension mismatch between text and videosequences. We propose a sliding window method to address the issues of aligningvariable-length text with video sequences. To our knowledge, USLNet is thefirst unsupervised sign language translation and generation model capable ofgenerating both natural language text and sign language video in a unifiedmanner. Experimental results on the BBC-Oxford Sign Language dataset (BOBSL)and Open-Domain American Sign Language dataset (OpenASL) reveal that USLNetachieves competitive results compared to supervised baseline models, indicatingits effectiveness in sign language translation and generation.
更多查看译文
AI 理解论文
溯源树
样例
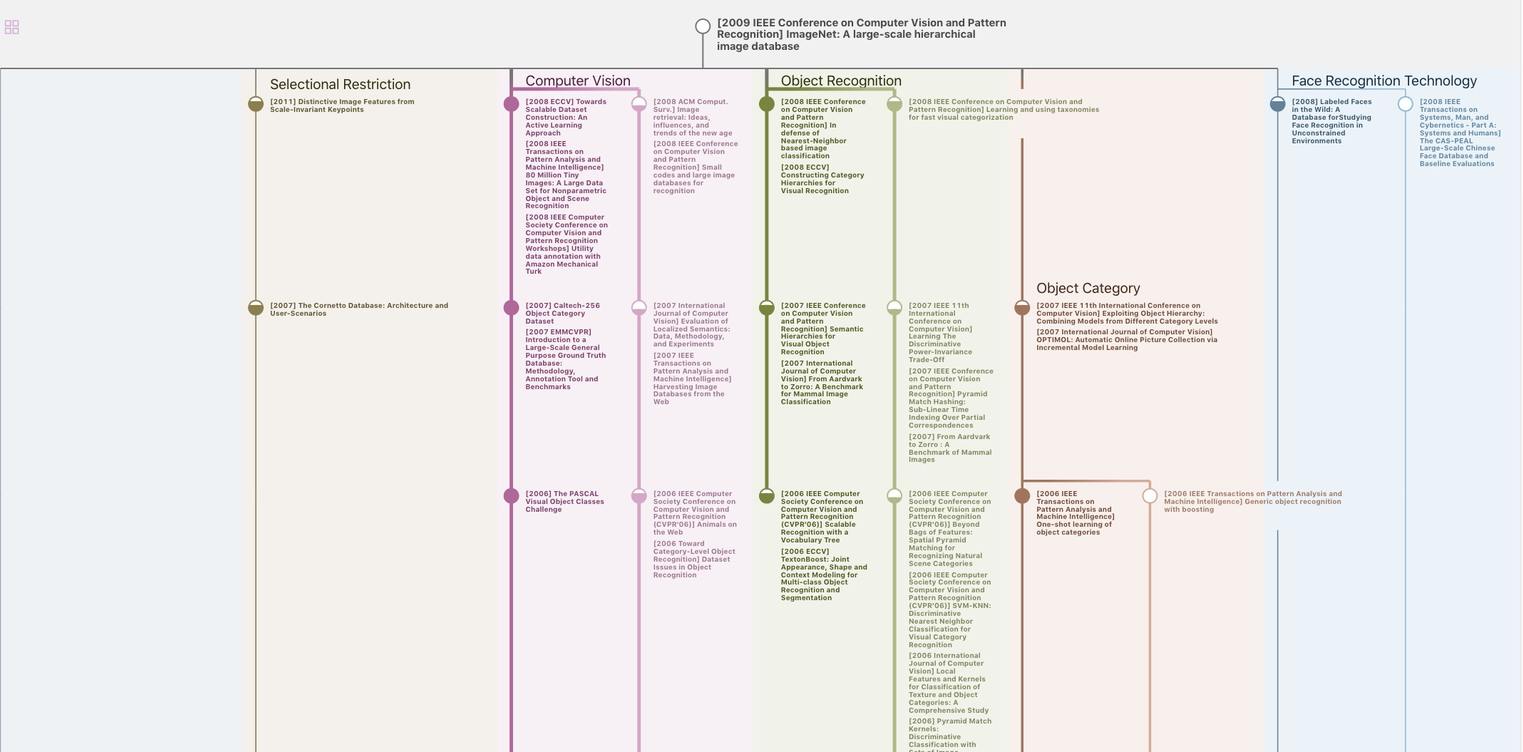
生成溯源树,研究论文发展脉络
Chat Paper
正在生成论文摘要