Ensemble Uncertainty Guided Road Scene Anomaly Detection: A Simple Meta-Learning Approach
IEEE Transactions on Intelligent Transportation Systems(2024)
摘要
A simple method is presented for road scene anomaly detection, which is particularly important in autonomous driving. Without the prior knowledge about the appearance and size, anomaly detection is formulated as an OoD (Out-of-Distribution) detection task. Epistemic uncertainty indicates that when a model is trained with finite training data, it will fail to yield reliable prediction if the testing data is far from the training data distribution (OoD). Deviating from previous works, instead of modelling the uncertainty of a single model explicitly, this work casts the individual uncertainty into an ensemble uncertainty metric by measuring the discrepancy between the different models. The benefits are: 1) Following a meta-learning framework, outputs from the off-the-shelf models can be used directly, which results in a very small training cost; 2) With the ensemble approach, the model is especially robust to dataset shift. Experimental results show that the performance of the proposed method is competitive: 1) Its APs (Average precisions) are 3.5%, 3.1%, and 5.2% higher than the best SOTAs (State-of-the-arts) on LaF (Lost-and-Found), RA (Road Anomaly), and RO (Road Obstacles) respectively; 2) For all datasets with different road conditions, it obtains consistent performance. (Code is available at https://github.com/yazhouliu/EUG).
更多查看译文
关键词
Uncertainty,Roads,Anomaly detection,Training data,Data models,Training,Task analysis,semantic segmentation,ensemble uncertainty,meta learning
AI 理解论文
溯源树
样例
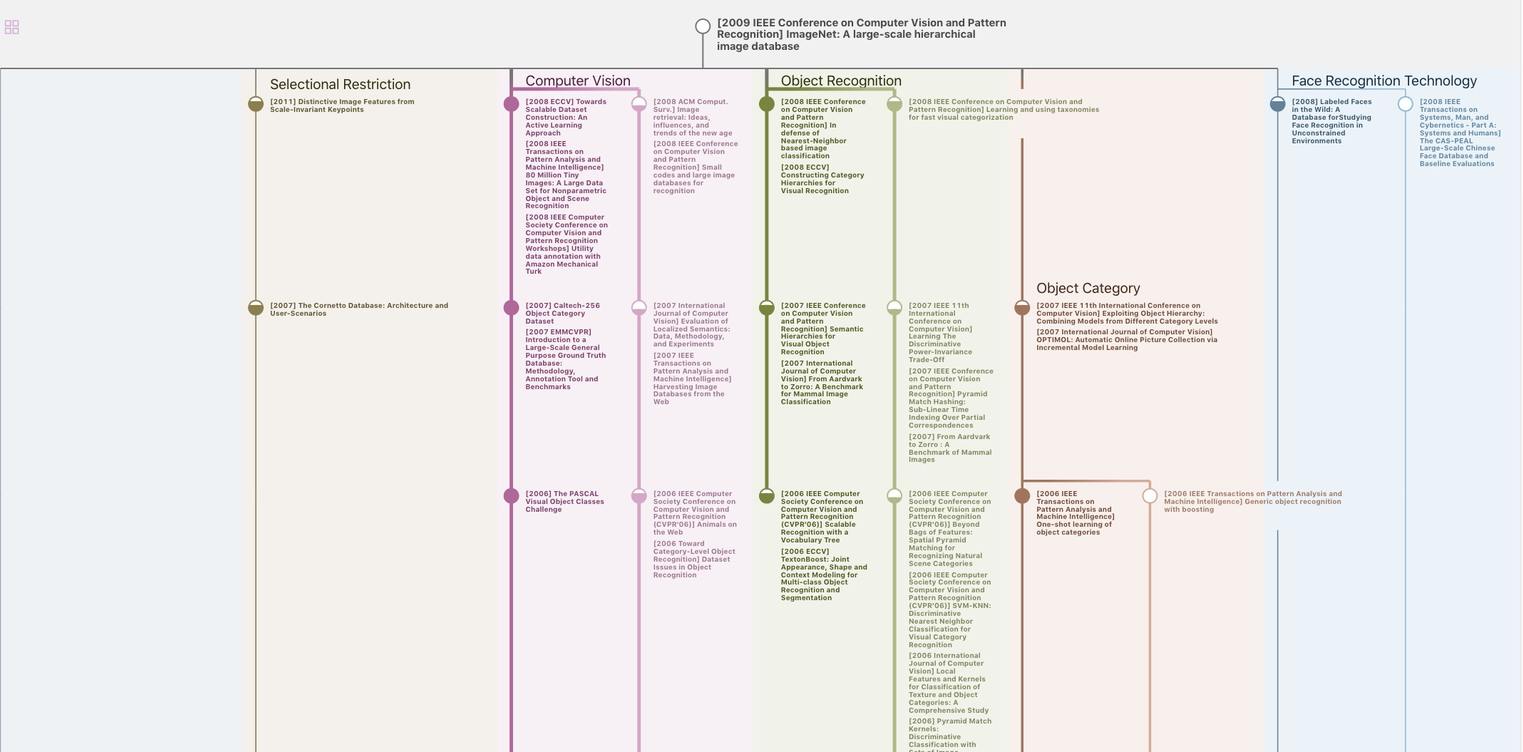
生成溯源树,研究论文发展脉络
Chat Paper
正在生成论文摘要