EXFOR-based Simultaneous Evaluation for Neutron-Induced Fission Cross Section of Plutonium-242
Journal of Nuclear Science and Technology(2023)
关键词
Plutonium-242,fission,simultaneous evaluation,JENDL,EXFOR
AI 理解论文
溯源树
样例
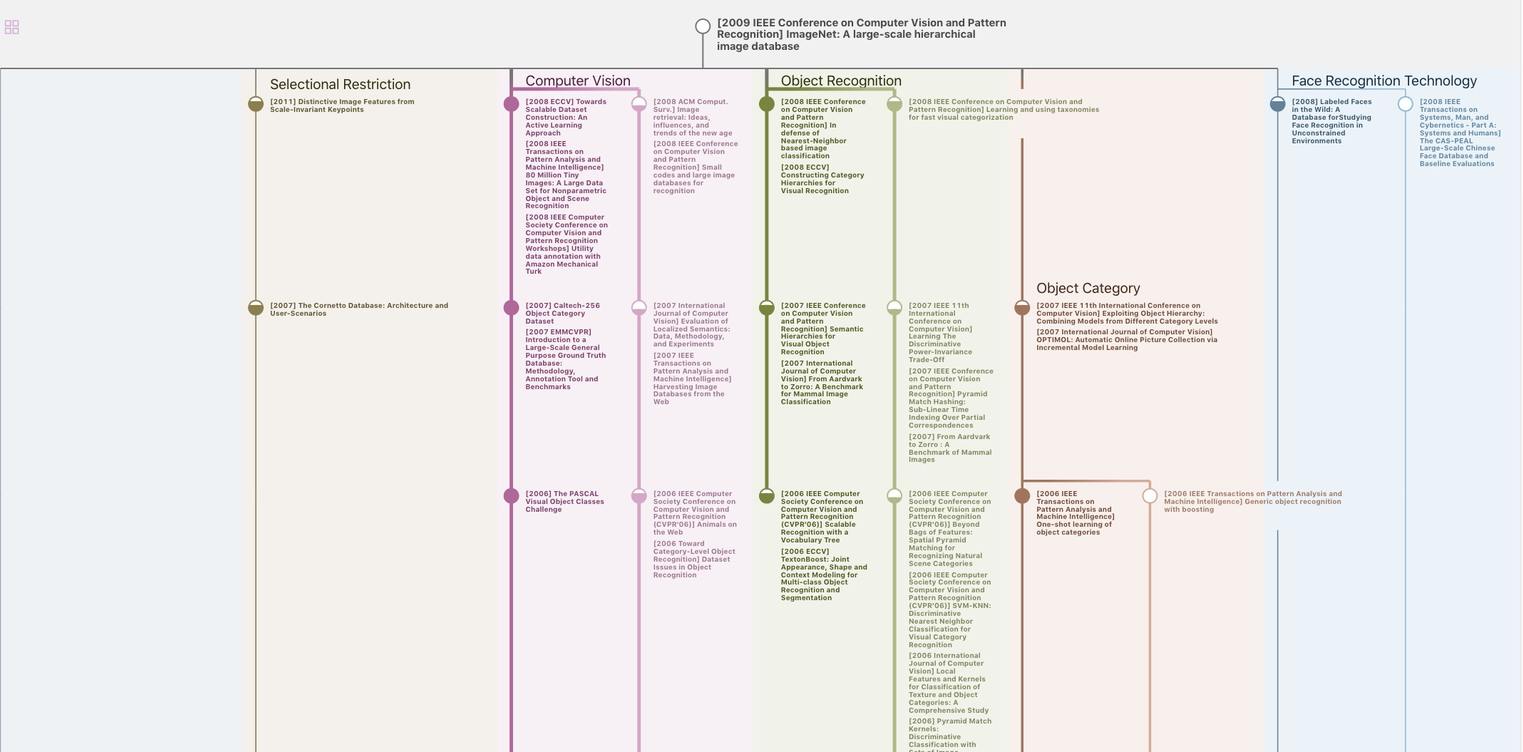
生成溯源树,研究论文发展脉络
Chat Paper
正在生成论文摘要