Pavement Crack Detection Algorithm Based on Reinforcement Learning and Traditional Supervised Learning
Fifteenth International Conference on Graphics and Image Processing (ICGIP 2023)(2024)
摘要
Traditional supervised learning has achieved high accuracy in crack detection tasks. However, due to the complex pavement situation, it often requires millions of data to train model in industry. It is difficult to process large-scale data to make it suitable for training. The very diverse data also result in the learning bottleneck of traditional supervised learning. In addition, the redundancy of network units in the actual forward inference of the model will waste lots of computing resources and slow the speed, which is also limiting overall detection effect. To solve these problems, we propose an automatic pavement crack detection algorithm based on reinforcement learning to optimize traditional supervised training. Firstly, based automatic pruning strategy in reinforcement learning, the detection efficiency of traditional supervised learning model can be improved with little even no loss of accuracy. Secondly, after the training of traditional supervised learning model, selecting and optimizing data with poor detection effect can break the learning bottleneck. The experimental results show that the proposed algorithm can significantly improve the accuracy and inference efficiency of crack detection under the actual complex pavement situation, and has achieved remarkable detection effect on the laboratory engineering project data set CiCS500 and several other public data sets.
更多查看译文
AI 理解论文
溯源树
样例
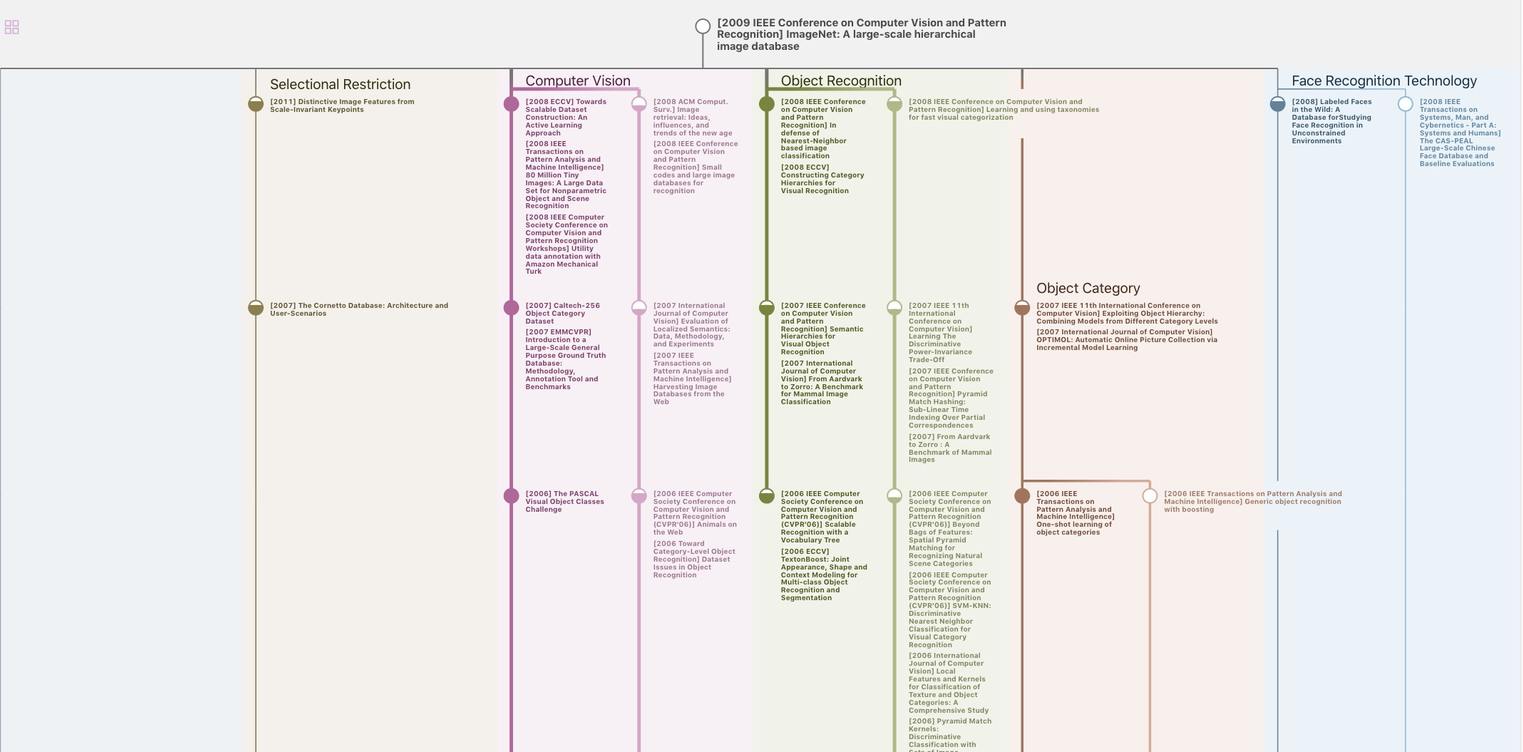
生成溯源树,研究论文发展脉络
Chat Paper
正在生成论文摘要