TableLLM: Enabling Tabular Data Manipulation by LLMs in Real Office Usage Scenarios
arXiv (Cornell University)(2024)
摘要
We introduce TableLLM, a robust large language model (LLM) with 13 billionparameters, purpose-built for proficiently handling tabular data manipulationtasks, whether they are embedded within documents or spreadsheets, catering toreal-world office scenarios. We propose a distant supervision method fortraining, which comprises a reasoning process extension strategy, aiding intraining LLMs to understand reasoning patterns more effectively as well as across-way validation strategy, ensuring the quality of the automaticallygenerated data. To evaluate the performance of TableLLM, we have crafted abenchmark tailored to address both document and spreadsheet formats as well asconstructed a well-organized evaluation pipeline capable of handling bothscenarios. Thorough evaluations underscore the advantages of TableLLM whencompared to various existing general-purpose and tabular data-focused LLMs. Wehave publicly released the model checkpoint, source code, benchmarks, and a webapplication for user interaction.
更多查看译文
AI 理解论文
溯源树
样例
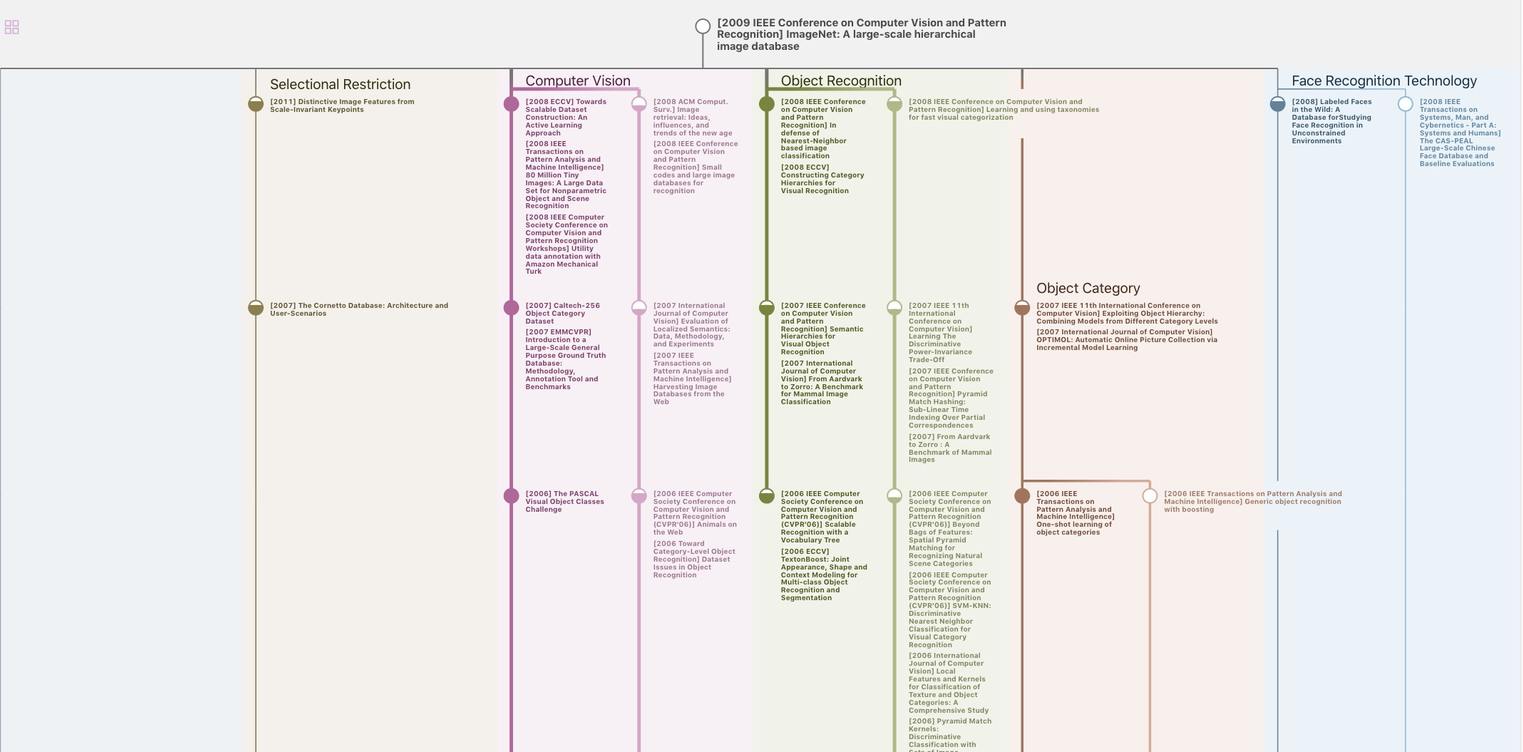
生成溯源树,研究论文发展脉络
Chat Paper
正在生成论文摘要