Airfoil Application of AMPL: an Automated Machine Learning Pipeline
openalex(2024)
摘要
Machine learning (ML) techniques have risen in popularity as ML has shown to be useful in providing an expert level response to predicting values, recognizing patterns, and identifying objects in images. While working through applying ML to estimate airfoil drag coefficients and kinetic energy reduction for terminal ballistics, it was noted that the steps for applying ML were similar enough to where a single workflow could be designed to address both of these problems, and a general pipeline could be used for previously unexplored problem spaces. The steps that were taken for both the rotorcraft airfoil and ballistics problems were feature importance, hyperparameter optimization which is searching for superior model parameters, training the best models returned from hyperparameter optimization, and evaluating the performance of the best models. This work describes the details of each of these steps, and how to use each of the steps individually or as an automated workflow or pipeline. In this work we describe an automated machine-learning pipeline and present the results obtained using the pipeline to predict the lift, drag, and moment coefficients of a rotorcraft airfoil.
更多查看译文
AI 理解论文
溯源树
样例
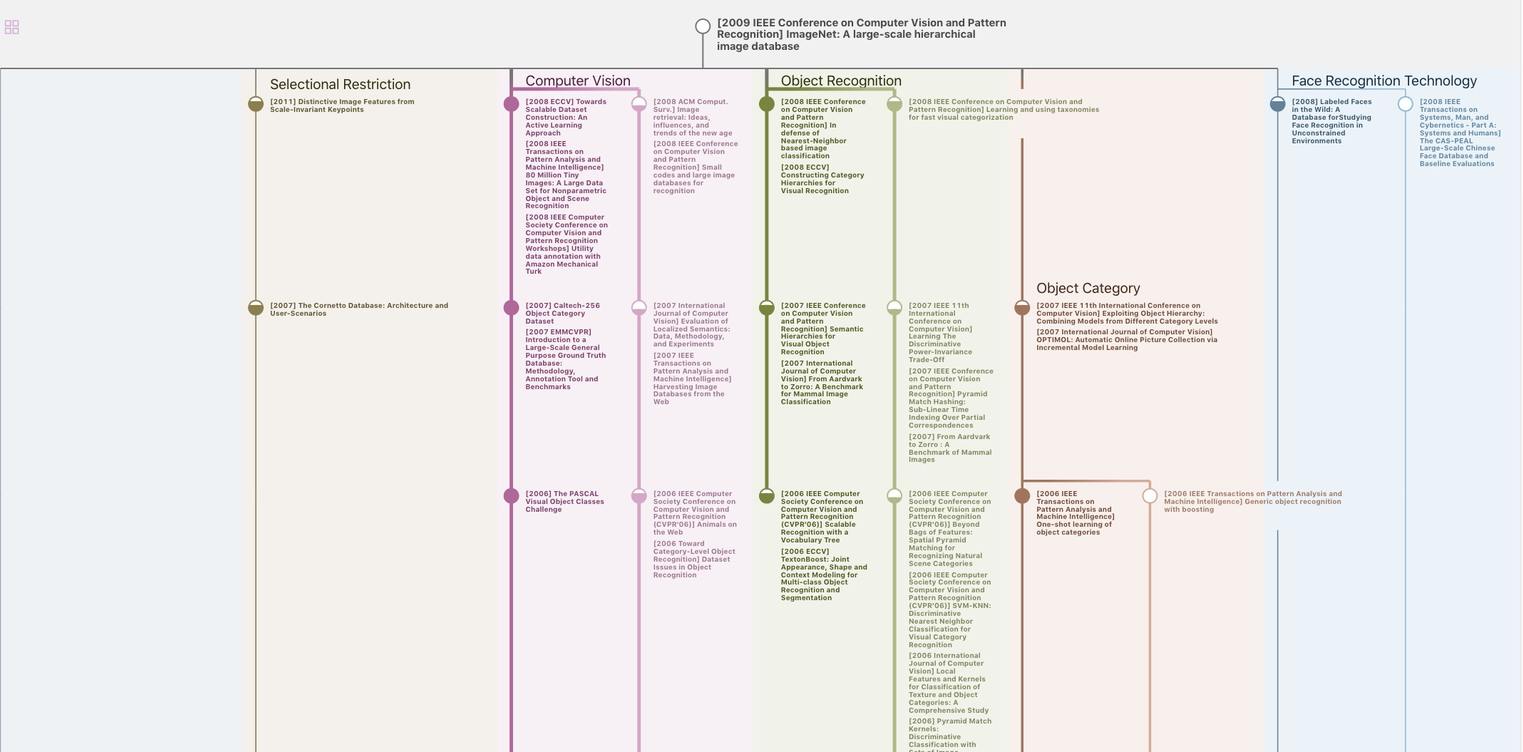
生成溯源树,研究论文发展脉络
Chat Paper
正在生成论文摘要