READ: Improving Relation Extraction from an ADversarial Perspective
NAACL-HLT (Findings)(2024)
摘要
Recent works in relation extraction (RE) have achieved promising benchmarkaccuracy; however, our adversarial attack experiments show that these worksexcessively rely on entities, making their generalization capabilityquestionable. To address this issue, we propose an adversarial training methodspecifically designed for RE. Our approach introduces both sequence- andtoken-level perturbations to the sample and uses a separate perturbationvocabulary to improve the search for entity and context perturbations.Furthermore, we introduce a probabilistic strategy for leaving clean tokens inthe context during adversarial training. This strategy enables a larger attackbudget for entities and coaxes the model to leverage relational patternsembedded in the context. Extensive experiments show that compared to variousadversarial training methods, our method significantly improves both theaccuracy and robustness of the model. Additionally, experiments on differentdata availability settings highlight the effectiveness of our method inlow-resource scenarios. We also perform in-depth analyses of our proposedmethod and provide further hints. We will release our code athttps://github.com/David-Li0406/READ.
更多查看译文
AI 理解论文
溯源树
样例
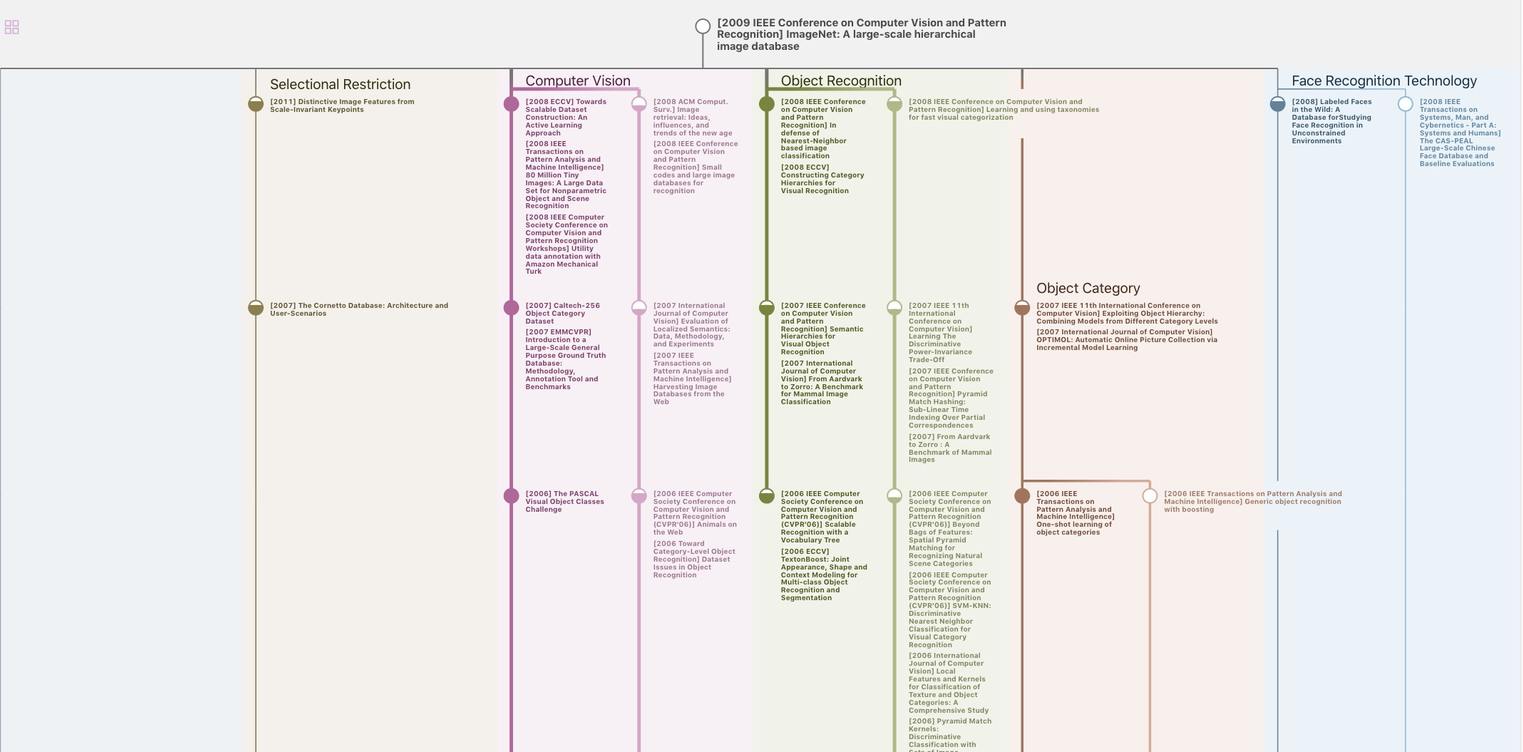
生成溯源树,研究论文发展脉络
Chat Paper
正在生成论文摘要