Self-supervised Contrastive Learning of Radio Data for Source Detection, Classification and Peculiar Object Discovery
Publications of the Astronomical Society of Australia(2024)
摘要
New advancements in radio data post-processing are underway within the SKAprecursor community, aiming to facilitate the extraction of scientific resultsfrom survey images through a semi-automated approach. Several of thesedevelopments leverage deep learning (DL) methodologies for diverse tasks,including source detection, object or morphology classification, and anomalydetection. Despite substantial progress, the full potential of these methodsoften remains untapped due to challenges associated with training largesupervised models, particularly in the presence of small and class-unbalancedlabelled datasets. Self-supervised learning has recently established itself asa powerful methodology to deal with some of the aforementioned challenges, bydirectly learning a lower-dimensional representation from large samples ofunlabelled data. The resulting model and data representation can then be usedfor data inspection and various downstream tasks if a small subset of labelleddata is available. In this work, we explored contrastive learning methods tolearn suitable radio data representation from unlabelled images taken from theASKAP EMU and SARAO MeerKAT GPS surveys. We evaluated trained models and theobtained data representation over smaller labelled datasets, also taken fromdifferent radio surveys, in selected analysis tasks: source detection andclassification, and search for objects with peculiar morphology. For allexplored downstream tasks, we reported and discussed the benefits brought byself-supervised foundational models built on radio data.
更多查看译文
AI 理解论文
溯源树
样例
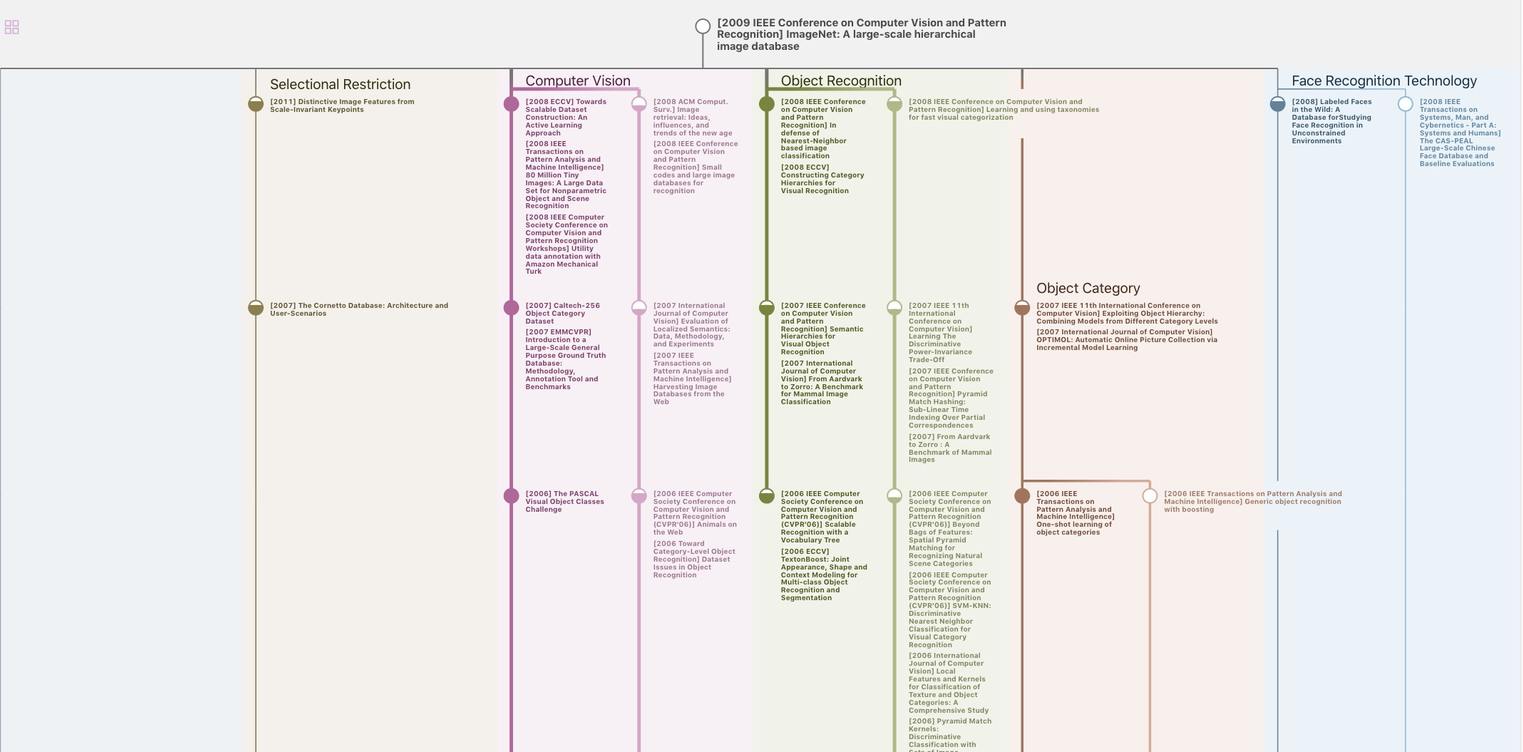
生成溯源树,研究论文发展脉络
Chat Paper
正在生成论文摘要