Modeling New Nature of Extraction and State Identification of Vibration Shock Signals from Hydroelectric Generating Units Using LCGSA Optimized RBF Combined with CEEMDAN Sample Entropy
IEEE ACCESS(2024)
摘要
The feature extraction and state recognition of vibration signals of hydroelectric generating units are of great significance for effectively ensuring the safety and lifespan of unit operation. This article proposes a fault diagnosis method for hydroelectr ic units using wavelet threshold algorithm preprocessing and improved (Gravitational Search Algorithm) GSA optimizer optimized (Radial Basis Function) RBF neural network. Using the (Wavelet Transform) WT algorithm for denoising preprocessing of the origina l signal, the processed signal is decomposed using (Complete Ensemble Empirical Mode Decomposition With Adaptive Noise) CEEMDAN, and IMF components are selected using the correlation coefficient method for sample entropy calculation. Finally, a GSA RBF fault diagnosis model for hydroelectric generating units is established. To improve convergence speed and accuracy, Lévy flight and chaotic sequence optimization GSA are introduced to reestablish the LCGSA RBF diagnosis model. The experimental results show th at the accuracy of this method reaches 100%, which is superior to other comparative models. Compared with CEEMDAN RBF, the accuracy of this method has been improved by 12.5%, and the diagnostic results have the highest consistency with the actual results. This study is of great significance for promoting the safe and reliable operation of hydroelectric units.
更多查看译文
关键词
Vibrations,Entropy,Feature extraction,Fault diagnosis,Electric shock,Renewable energy sources,Optimization,Hydroelectric power generation,CEEMDAN,sample entropy,LCGSA-RBF,vibration shock signals,hydroelectric generating units
AI 理解论文
溯源树
样例
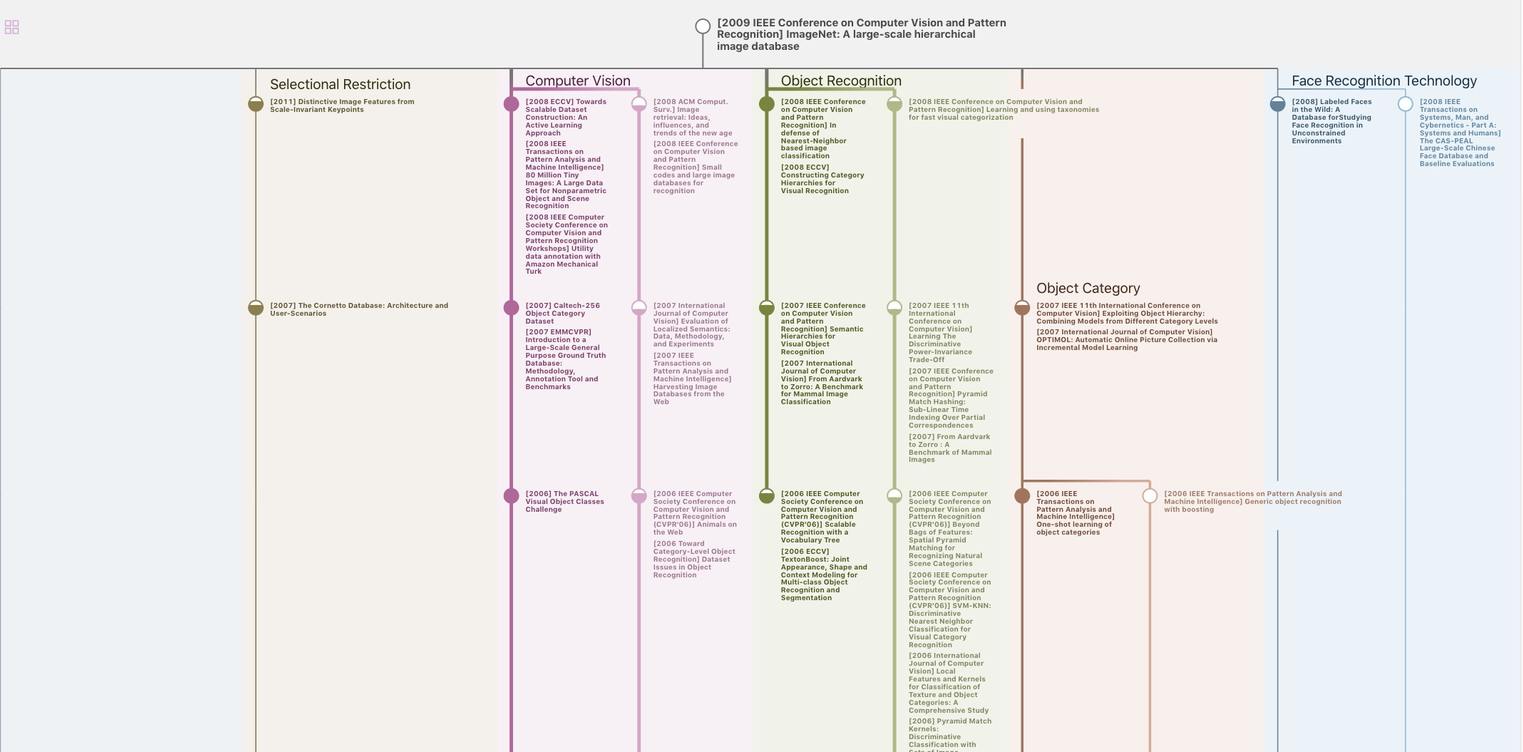
生成溯源树,研究论文发展脉络
Chat Paper
正在生成论文摘要