Gradient-Mask Tuning Elevates the Upper Limits of LLM Performance
CoRR(2024)
Abstract
Large language models (LLMs) have revolutionized lots of fields of research.
Although it is well-known that fine-tuning is essential for enhancing the
capabilities of LLMs, existing research suggests that there is potential
redundancy in the fine-tuning process and therefore proposes to update only a
subset of parameters. However, these methods fail to leverage the task-specific
information to identify important parameters during training. Based on the
insight that gradients inherently contain information on task-specific data, we
propose Gradient-Mask Tuning (GMT), a method that selectively updates
parameters during training based on their gradient information. Specifically,
we compute the absolute values of the gradients and apply masking to those with
relatively smaller magnitudes. Our empirical results across various tasks
demonstrate that GMT not only outperforms traditional fine-tuning methods but
also elevates the upper limits of LLM performance. Further analysis indicates
that GMT exhibits insensitivity to mask ratio and possesses computational
efficiency comparable to vanilla SFT.
MoreTranslated text
AI Read Science
Must-Reading Tree
Example
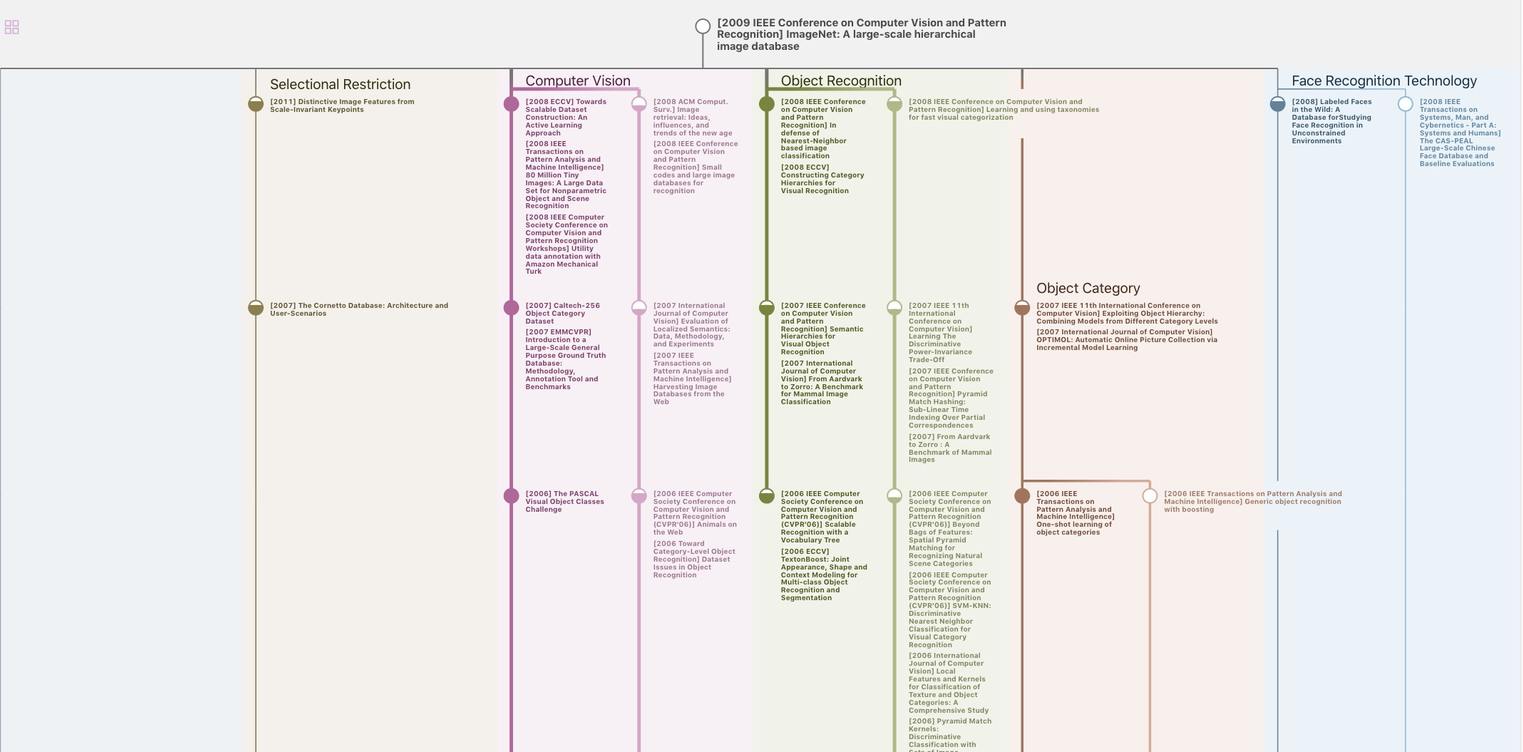
Generate MRT to find the research sequence of this paper
Chat Paper
Summary is being generated by the instructions you defined