ION: Navigating the HPC I/O Optimization Journey Using Large Language Models
PROCEEDINGS OF THE 2024 16TH ACM WORKSHOP ON HOT TOPICS IN STORAGE AND FILE SYSTEMS, HOTSTORAGE 2024(2024)
摘要
Effectively leveraging the complex software and hardware I/O stacks of HPC systems to deliver needed I/O performance has been a challenging task for domain scientists. To identify and address I/O issues in their applications, scientists largely rely on I/O experts to analyze the recorded I/O traces of their applications and provide insights into the potential issues. However, due to the limited number of I/O experts and the growing demand for data-intensive applications across the wide spectrum of sciences, inaccessibility has become a major bottleneck hindering scientists from maximizing their productivity. Inspired by the recent rapid progress of large language models (LLMs), in this work we propose IO Navigator (ION), an LLM-based framework that takes a recorded I/O trace of an application as input and leverages the in-context learning, chain-of-thought, and code generation capabilities of LLMs to comprehensively analyze the I/O trace and provide diagnosis of potential I/O issues. Similar to an I/O expert, ION provides detailed justifications for the diagnosis and an interactive interface for scientists to ask detailed questions about the diagnosis. We illustrate ION's applicability by assessing it on a set of controlled I/O traces generated with different I/O issues. We also demonstrate that ION can match state-of-the-art I/O optimization tools and provide more insightful and adaptive diagnoses for real applications. We believe ION, with its full capabilities, has the potential to become a powerful tool for scientists to navigate through complex I/O subsystems in the future.
更多查看译文
AI 理解论文
溯源树
样例
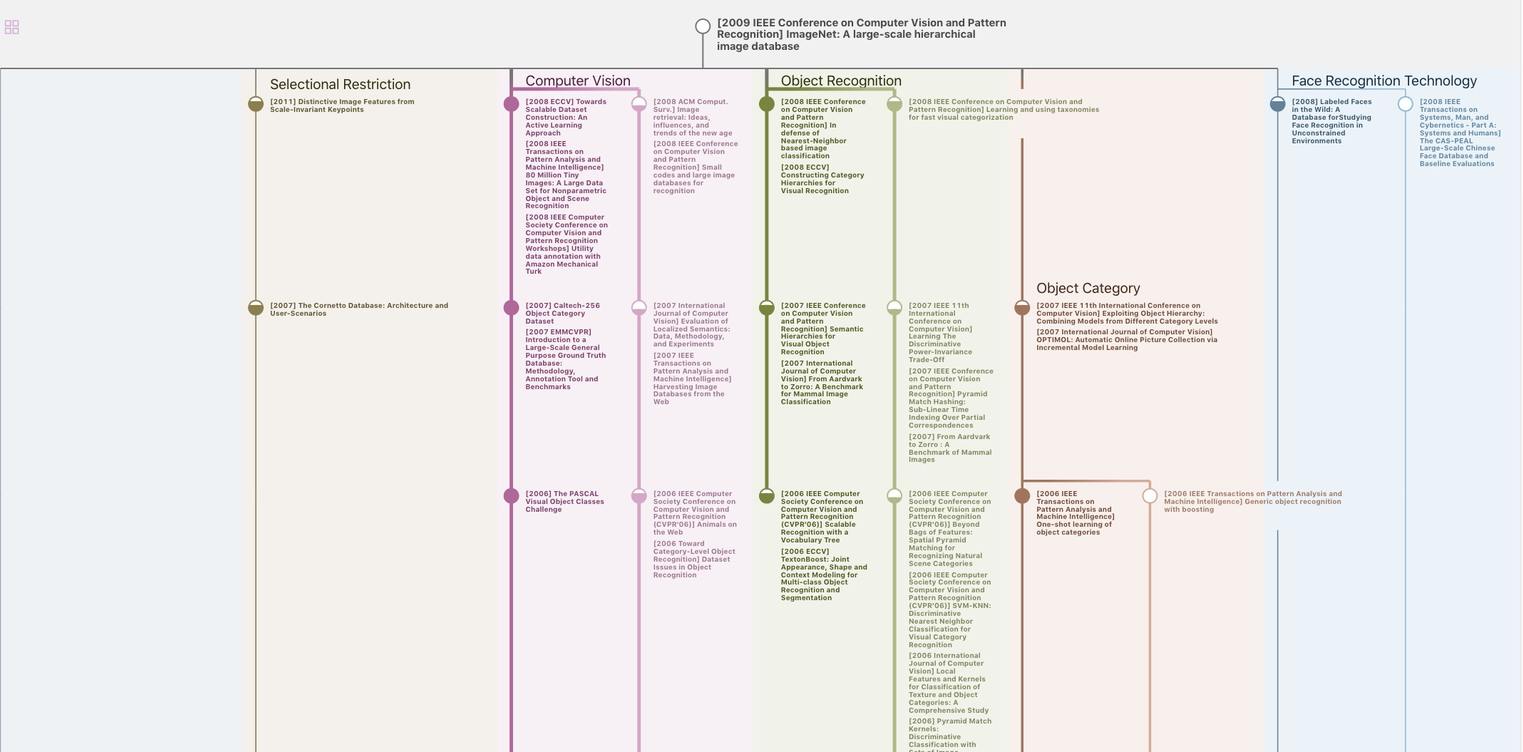
生成溯源树,研究论文发展脉络
Chat Paper
正在生成论文摘要