ADDER: Service-Specific Adaptive Data-Driven Radio Resource Control for Cellular-IoT
PROCEEDINGS 2024 IEEE 25TH INTERNATIONAL SYMPOSIUM ON A WORLD OF WIRELESS, MOBILE AND MULTIMEDIA NETWORKS, WOWMOM 2024(2024)
Abstract
Energy-saving methods like Discontinuous Reception (DRX) and Power Save Mode (PSM) are commonly used in Internet of Thing (IoT) applications, allowing for sleep and awake cycle adjustments to save energy. However, understanding and configuring these parameters on devices, especially actuator-type devices, is challenging for IoT service providers. Unlike sensor types, these devices must complete their sleep cycle before responding to infrequent downlink commands, making efficient parameter selection and traffic prediction vital for energy efficiency and command reception. To address this, we present ADDER, a network-side solution leveraging a context-aware traffic predictor. This predictor forecasts downlink arrival probabilities, guiding a deep deterministic policy gradient (DDPG) policymaker that selects energy-saving parameters based on thresholds defined by the IoT service providers. ADDER, leverages contextual information like day of week, hour, weather, holidays, and events, shifting the focus from individual device histories (often erratic) to analyzing broader service traffic patterns. This data-driven strategy enables ADDER to adjust energy-saving settings for the best balance between energy efficiency and latency, customizing to the unique requirements of each service and removing the burden of configuring complex network settings. We observed that ADDER meets latency needs while achieving a 5.9% reduction in energy consumption for services requiring rapid responses. For applications prioritizing energy conservation, such as irrigation systems and city lighting, ADDER achieves a significant 32.7% reduction in energy consumption with a slight increase (9%) in messages might not meet the strictest latency requirements. To evaluate the consequences of prediction inaccuracies from our predictor, we utilized a real-world shared mobility dataset provided by Austin's Transportation Department for a case study.
MoreTranslated text
Key words
NB-IoT,LTE-M,energy saving,context bandit,deep reinforcement learning
AI Read Science
Must-Reading Tree
Example
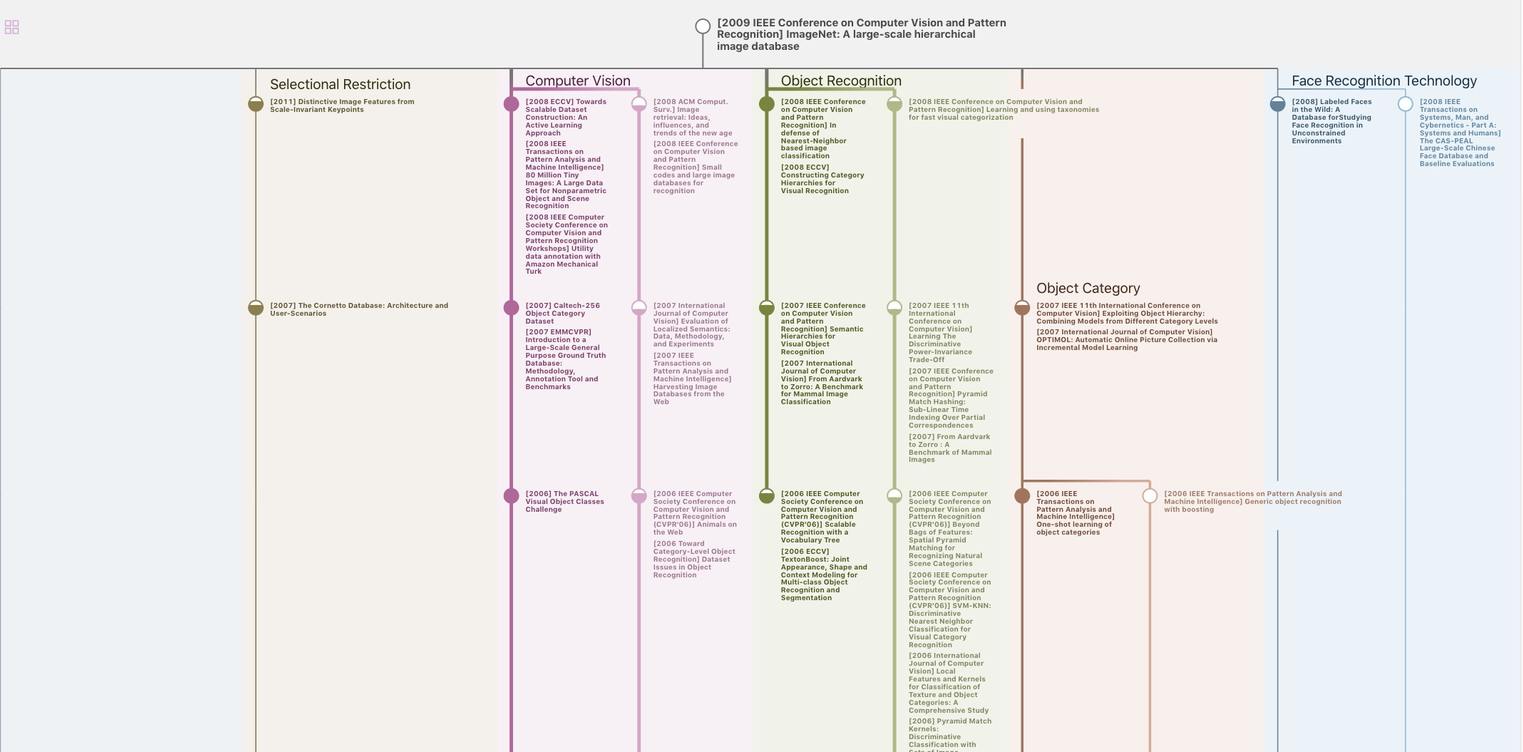
Generate MRT to find the research sequence of this paper
Chat Paper
Summary is being generated by the instructions you defined