Amortized Inference for Efficient Grasp Model Adaptation
ICRA 2024(2024)
摘要
In robotic applications such as bin-picking or block-stacking, learned predictive models have been developed for manipulation of objects with varying but known dynamic properties (e.g., mass distributions and friction coefficients). When a robot encounters a new object, these properties are often difficult to observe and must be inferred through interaction, which can be expensive in both inference time and number of interactions. We propose an encoder/decoder action-feasibility model to efficiently adapt to new objects by estimating their unobserved properties through interaction. The encoder predicts a distribution over the unobserved parameters while the decoder predicts action feasibility, which can be used in an uncertainty-aware planner. An explicit representation of uncertainty in the encoder enables information-gathering heuristics to minimize adaptation interactions. The amortized distributions are efficient to compute and perform comparably to particle-based distributions in a grasping domain. Finally, we deploy our method on a Panda robot to grasp heavy objects.
更多查看译文
关键词
Probabilistic Inference,Transfer Learning,Deep Learning in Grasping and Manipulation
AI 理解论文
溯源树
样例
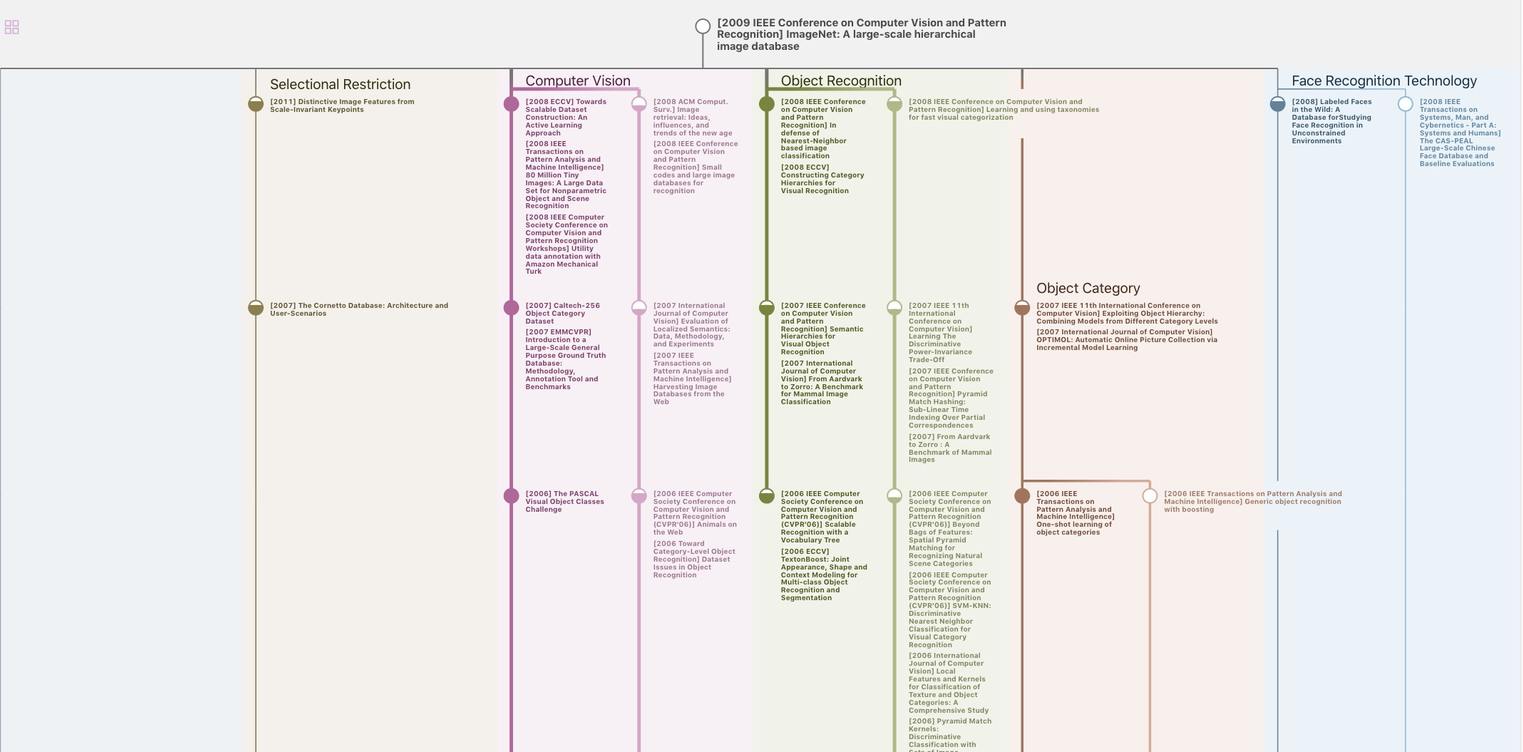
生成溯源树,研究论文发展脉络
Chat Paper
正在生成论文摘要