Nebula: An Edge-Cloud Collaborative Learning Framework for Dynamic Edge Environments.
International Conference on Parallel Processing(2024)
摘要
To bring the great power of modern DNNs into mobile computing and distributed systems, current practices primarily employ one of the two learning paradigms: cloud-based learning or on-device learning. Despite their distinct advantages, neither of these two paradigms could effectively deal with highly dynamic edge environments reflected in quick data distribution shifts and on-device resource fluctuations. In this work, we propose Nebula, an edge-cloud collaborative learning framework to enable rapid model adaptation for changing edge environments. To achieve this, we first propose a new block-level model decomposition scheme to decompose the large cloud model into multiple combinable modules. With this design, we can agilely derive personalized sub-models with compact sizes for edge devices, and quickly aggregate the updated sub-models to integrate new knowledge learned on the edge into the cloud model. We further propose an end-to-end learning framework that incorporates the modular model design into an efficient model adaptation pipeline, including an offline on-cloud model prototyping and training stage, and an online edge-cloud collaborative adaptation stage. Extensive experiments demonstrate that Nebula improves model performance (e.g., 18.89% accuracy increase) and resource efficiency (e.g., 7.12 × communication cost reduction) in adapting models to dynamic edge environments.
更多查看译文
AI 理解论文
溯源树
样例
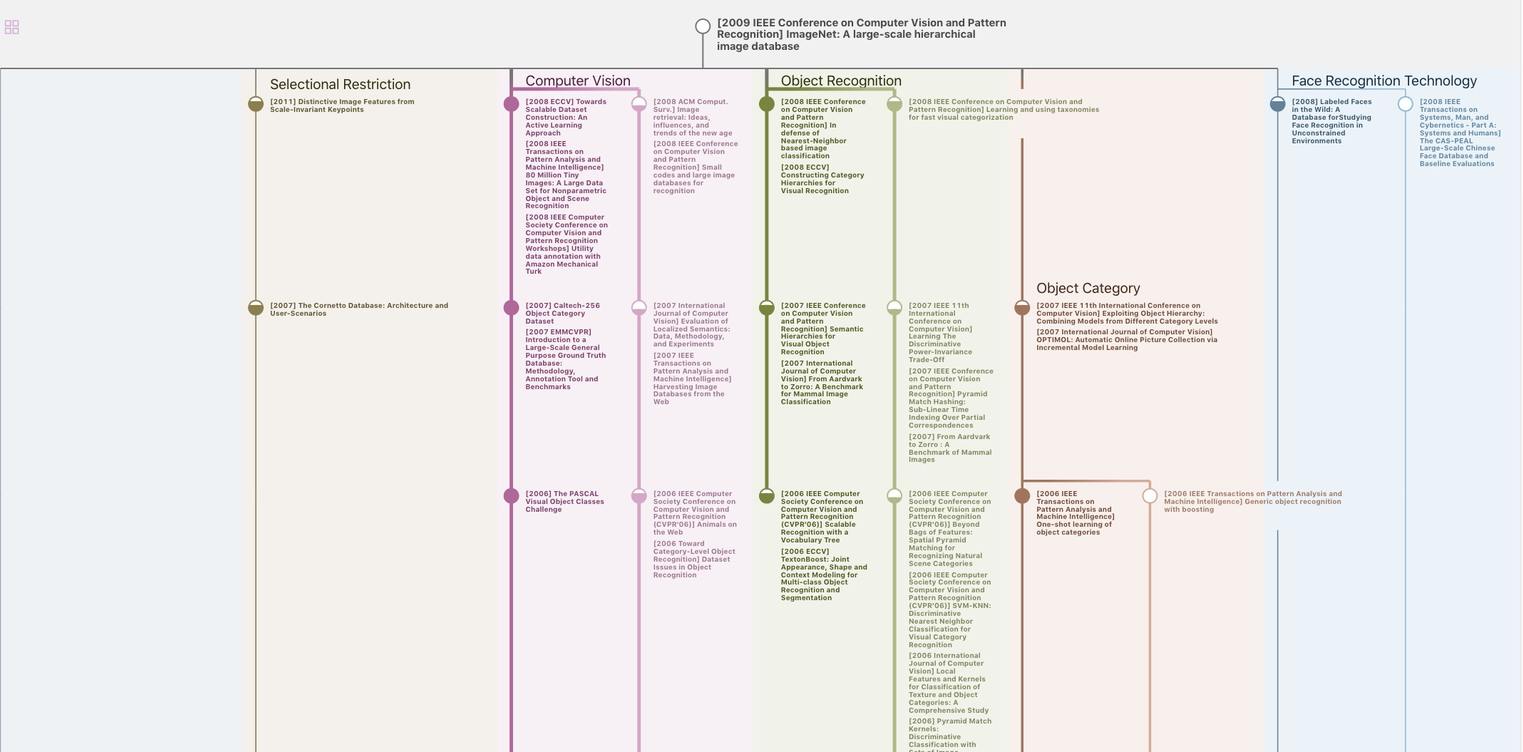
生成溯源树,研究论文发展脉络
Chat Paper
正在生成论文摘要