Using Multiple Scales of Movement to Highlight Risk–reward Strategies of Coyotes (canis Latrans) in Mixed‐use Landscapes
Ecosphere(2024)
摘要
AbstractMany wildlife species vary habitat selection across space, time, and behavior to maximize rewards and minimize risk. Multi‐scale research approaches that identify variation in wildlife habitat selection can highlight not only habitat preferences and risk tolerance but also movement strategies that afford coexistence or cause conflict with humans. Here, we examined how anthropogenic and natural features influenced coyote (Canis latrans) habitat selection in a mixed‐use, agricultural landscape in Mendocino County, California, USA. We used resource selection functions and hidden Markov models to test whether coyote selection for anthropogenic and natural features varied by time of day or by behavioral state (resting, foraging, and traveling). We found that coyotes avoided development, but, contrary to our expectations, coyotes selected for roads, agriculture, and areas with risk of human encounter and rifle use regardless of diel period or behavioral state. While traveling, coyotes increased selection for roads and avoided ruggedness, indicating that unpaved roads may enhance connectivity for coyotes in mixed‐use landscapes. Finally, we found that coyotes selected for mountain lion habitat when resting and at night, signifying that risk from natural predators was not a factor in habitat selection at coarse scales. Coyote habitat selection for places and times associated with human activity, without variation across scales, signals a potential for conflict if coyotes are perceived by people as a nuisance.
更多查看译文
关键词
behavioral partitioning,Canis latrans,habitat selection,hidden Markov model,humans,reward,risk,temporal partitioning
AI 理解论文
溯源树
样例
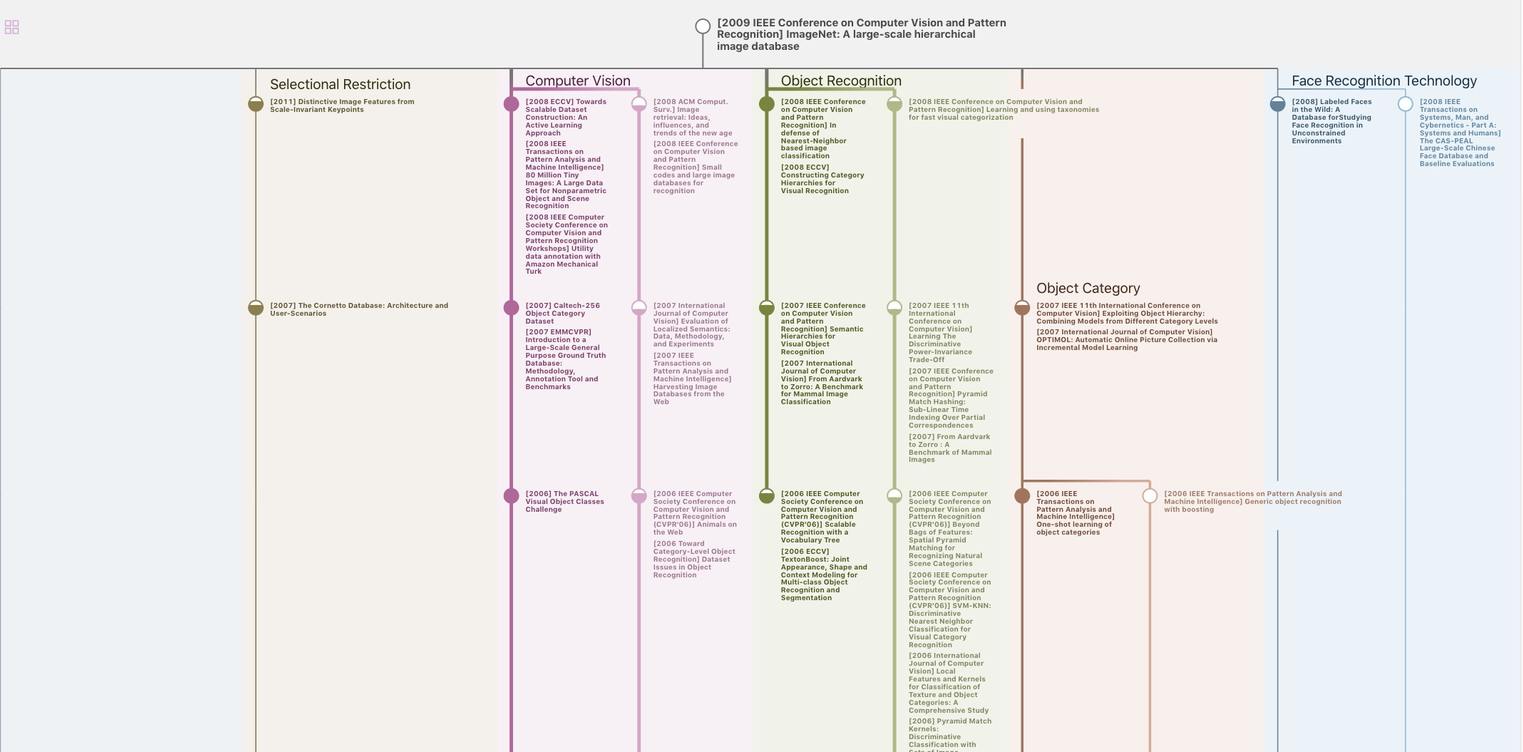
生成溯源树,研究论文发展脉络
Chat Paper
正在生成论文摘要