Enhanced Discriminative Fine-Tuning of Large Language Models for Chinese Text Classification
International Conference on Asian Language Processing(2024)
摘要
In the field of natural language processing, the era of Large Language Models (LLMs) has arrived. Text classification, being a classic and widely applied task, can greatly benefit from the advancements brought by LLMs to enhance its performance. We introduce an Enhanced Discriminative Fine-Tuning (ED-FT) approach aimed at enhancing discriminative text classification with LLMs, which we experimentally validate on the open-source Chinese LLM, Yi series. ED-FT utilizes a prompt encoder to search for the soft prompts in a continuous parameter space for downstream text classification tasks, combined with efficient fine-tuning techniques for LLMs. Additionally, we modify the attention mask of the Yi-6B model to incorporate bidirectional attention, enabling it to generate bidirectional representations of the input text sequences, which are then fed into an additional classification layer for non-generative text classification. We evaluate our method on three publicly available Chinese datasets: Tnews, ChnSentiCorp, and SMP2020-EWECT-Usual, achieving accuracy rates of 64.88%, 96.83%, and 83.35%, respectively. These results significantly surpass those of Bert-like models, achieving state-of-the-art performance. Additionally, it markedly improved inference speed. Furthermore, we explore the effectiveness of utilizing LLM intermediate layer hidden states for text classification, and the impact of LLM sizes.
更多查看译文
关键词
Large Language Models,Text Classification,Natural Language Processing
AI 理解论文
溯源树
样例
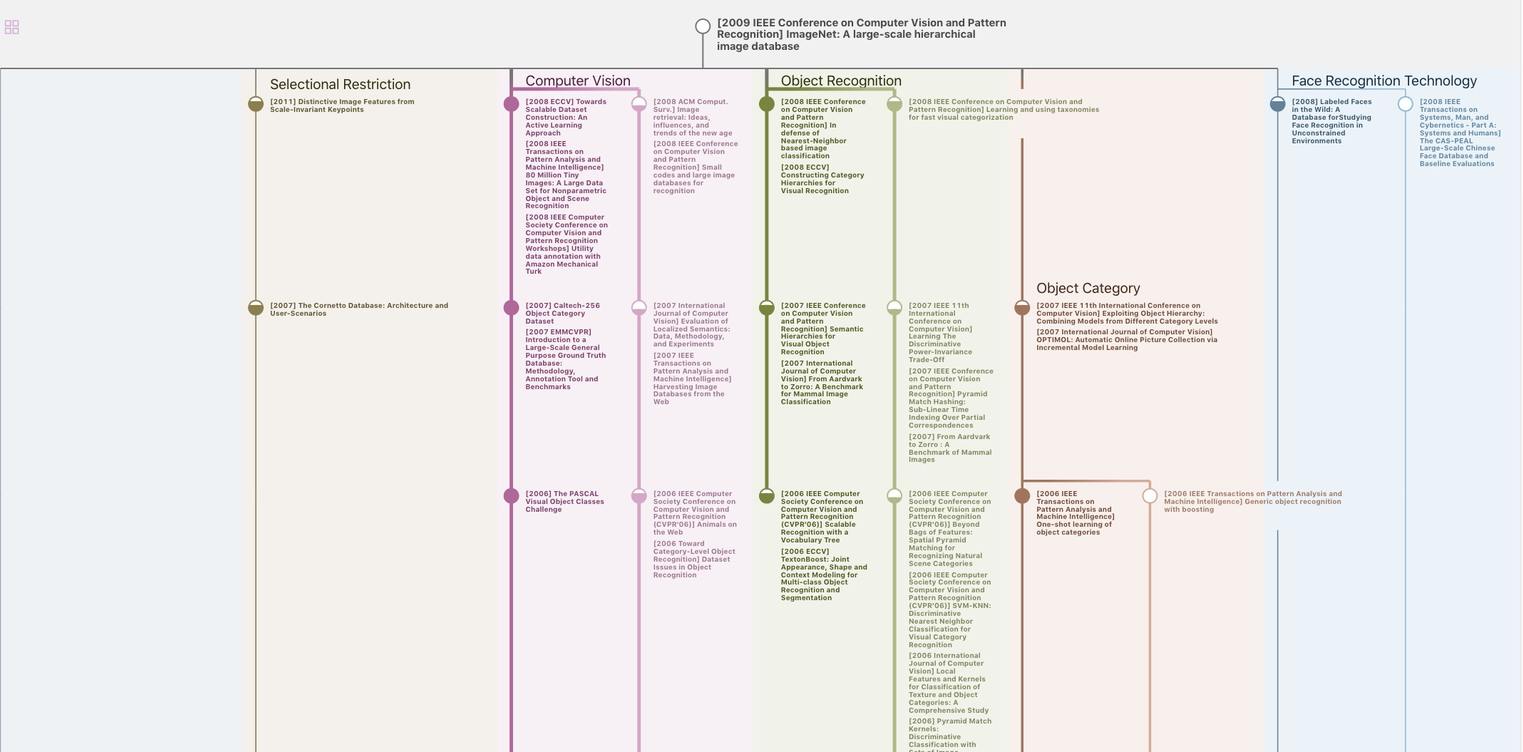
生成溯源树,研究论文发展脉络
Chat Paper
正在生成论文摘要