Marine Oil Pollution Monitoring Based on a Morphological Attention U-Net Using SAR Images
Sensors (Basel, Switzerland)(2024)
摘要
This study proposed an improved full-scale aggregated MobileUNet (FA-MobileUNet) model to achieve more complete detection results of oil spill areas using synthetic aperture radar (SAR) images. The convolutional block attention module (CBAM) in the FA-MobileUNet was modified based on morphological concepts. By introducing the morphological attention module (MAM), the improved FA-MobileUNet model can reduce the fragments and holes in the detection results, providing complete oil spill areas which were more suitable for describing the location and scope of oil pollution incidents. In addition, to overcome the inherent category imbalance of the dataset, label smoothing was applied in model training to reduce the model’s overconfidence in majority class samples while improving the model’s generalization ability. The detection performance of the improved FA-MobileUNet model reached an mIoU (mean intersection over union) of 84.55%, which was 17.15% higher than that of the original U-Net model. The effectiveness of the proposed model was then verified using the oil pollution incidents that significantly impacted Taiwan’s marine environment. Experimental results showed that the extent of the detected oil spill was consistent with the oil pollution area recorded in the incident reports.
更多查看译文
关键词
oil spills,U-Net model,synthetic aperture radar (SAR),convolutional block attention module (CBAM),label smoothing
AI 理解论文
溯源树
样例
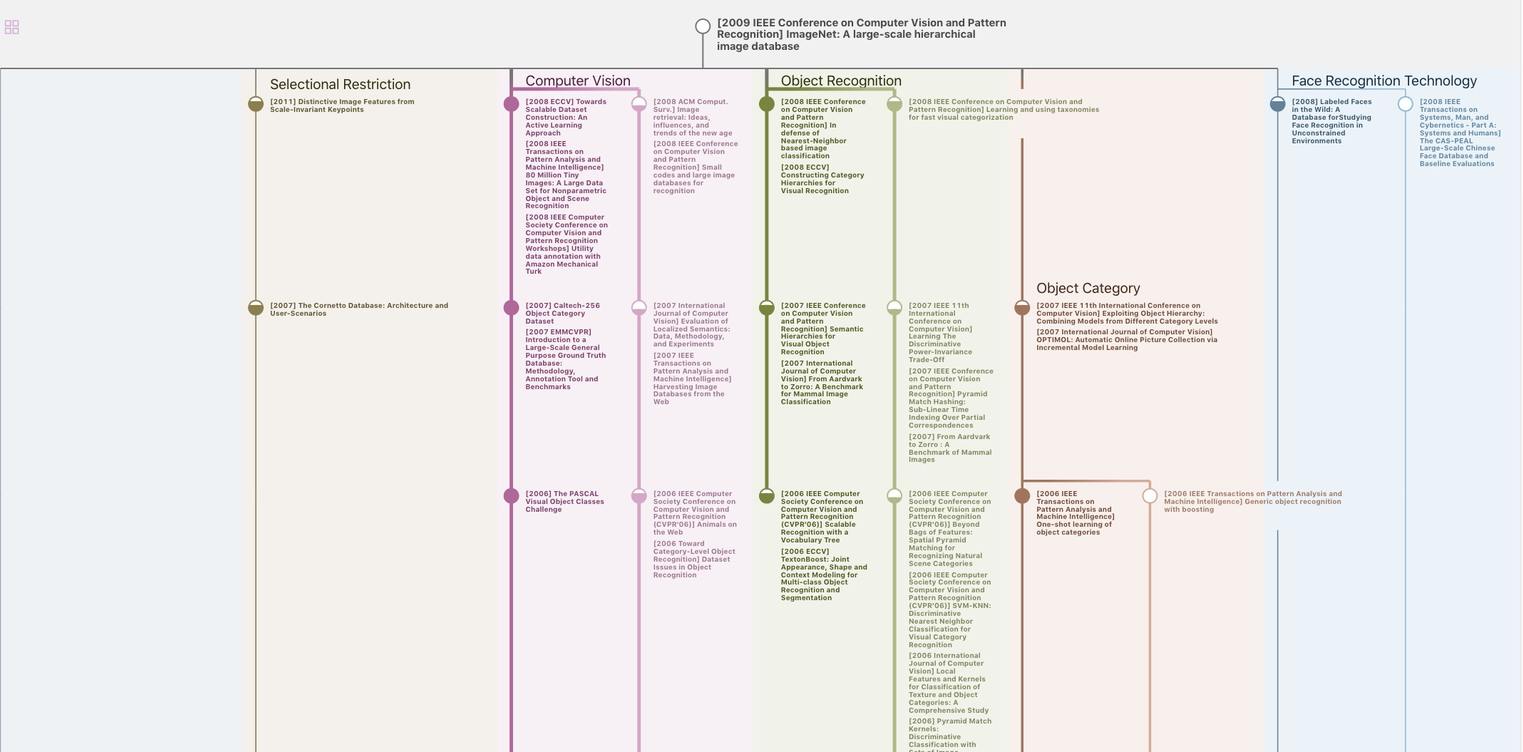
生成溯源树,研究论文发展脉络
Chat Paper
正在生成论文摘要