Longitudinal Monitoring of Donor Derived Cell Free DNA in Lung Transplant Recipients
The Journal of Heart and Lung Transplantation(2022)
关键词
Delayed Graft Function
AI 理解论文
溯源树
样例
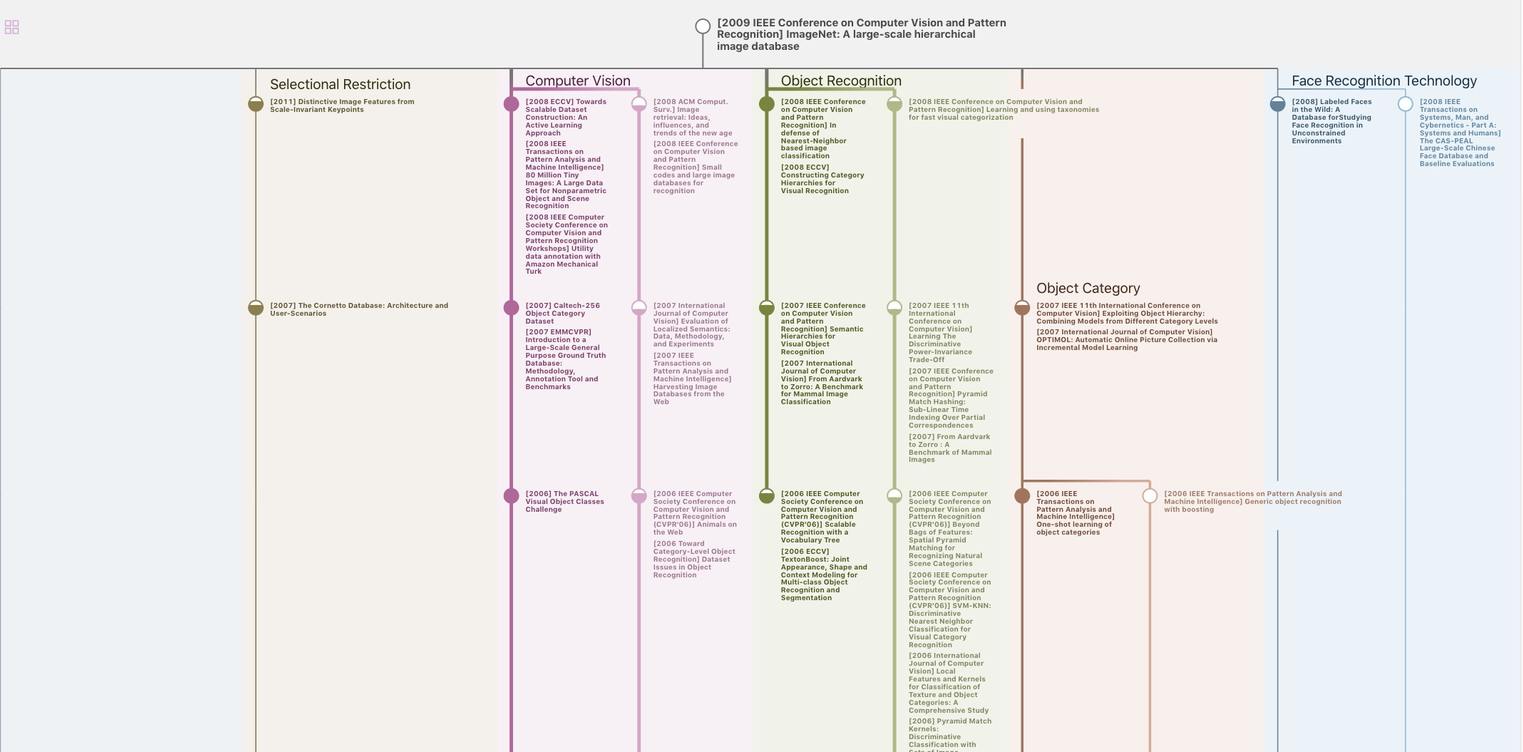
生成溯源树,研究论文发展脉络
Chat Paper
正在生成论文摘要
The Journal of Heart and Lung Transplantation(2022)