Effect of Polishing on the Morphology of Zircaloy-4 Nanostructure: Formation of a Novel Hexagonal Nanoscale Pattern.
RSC ADVANCES(2025)
PINSTECH | Islamia Coll Univ | King Saud Univ | Abdul Wali Khan Univ Mardan | Univ Poonch Rawalakot
Abstract
Zircaloy-4 (Zr-4) is widely used as the cladding material in nuclear power plants (NPPs) due to its excellent corrosion resistance and low neutron absorption cross-section. Under Loss of Coolant Accident (LOCA) conditions, oxidation of Zr-4 can compromise the safety of the NPPs by accelerating hydrogen production. Therefore, enhancing the oxidation resistance of Zr-4 is a critical research focus. Surface modification through a facile method offers a promising approach to address this issue. This study explores the impact of chemical, mechanical, and electropolishing (EP) pre-treatments on Zr-4 morphology before and after anodization. EP conducted in ethylene glycol monobutyl ether-perchloric acid electrolyte produced a mirror-like surface finish with hexagonal nanopattern formation under optimized conditions, as revealed by SEM studies. Anodization of the patterned surface in a glycerol-based electrolyte resulted in a hybrid (nanoporous and nanotubular) structure, unlike the nanotubular-only morphology observed in the chemically or mechanically polished samples. EDAX and XRD analyses confirmed the formation of ZrO2. The hexagonal nanopattern generated by EP suppressed the dissolution of the nanotube during anodization, resulting in a hybrid nanostructure. Additionally, the chemical polishing of EP-treated Zr-4 in HF : HNO3 : H2O generated a porous particulate structure due to selective etching. This work demonstrates that the surface pre-treatment significantly influences the morphology of anodized Zr-4 nanostructures and suggests the potential use of EP in other materials for hexagonal nanopattern generation.
MoreTranslated text
求助PDF
上传PDF
View via Publisher
AI Read Science
AI Summary
AI Summary is the key point extracted automatically understanding the full text of the paper, including the background, methods, results, conclusions, icons and other key content, so that you can get the outline of the paper at a glance.
Example
Background
Key content
Introduction
Methods
Results
Related work
Fund
Key content
- Pretraining has recently greatly promoted the development of natural language processing (NLP)
- We show that M6 outperforms the baselines in multimodal downstream tasks, and the large M6 with 10 parameters can reach a better performance
- We propose a method called M6 that is able to process information of multiple modalities and perform both single-modal and cross-modal understanding and generation
- The model is scaled to large model with 10 billion parameters with sophisticated deployment, and the 10 -parameter M6-large is the largest pretrained model in Chinese
- Experimental results show that our proposed M6 outperforms the baseline in a number of downstream tasks concerning both single modality and multiple modalities We will continue the pretraining of extremely large models by increasing data to explore the limit of its performance
Upload PDF to Generate Summary
Must-Reading Tree
Example
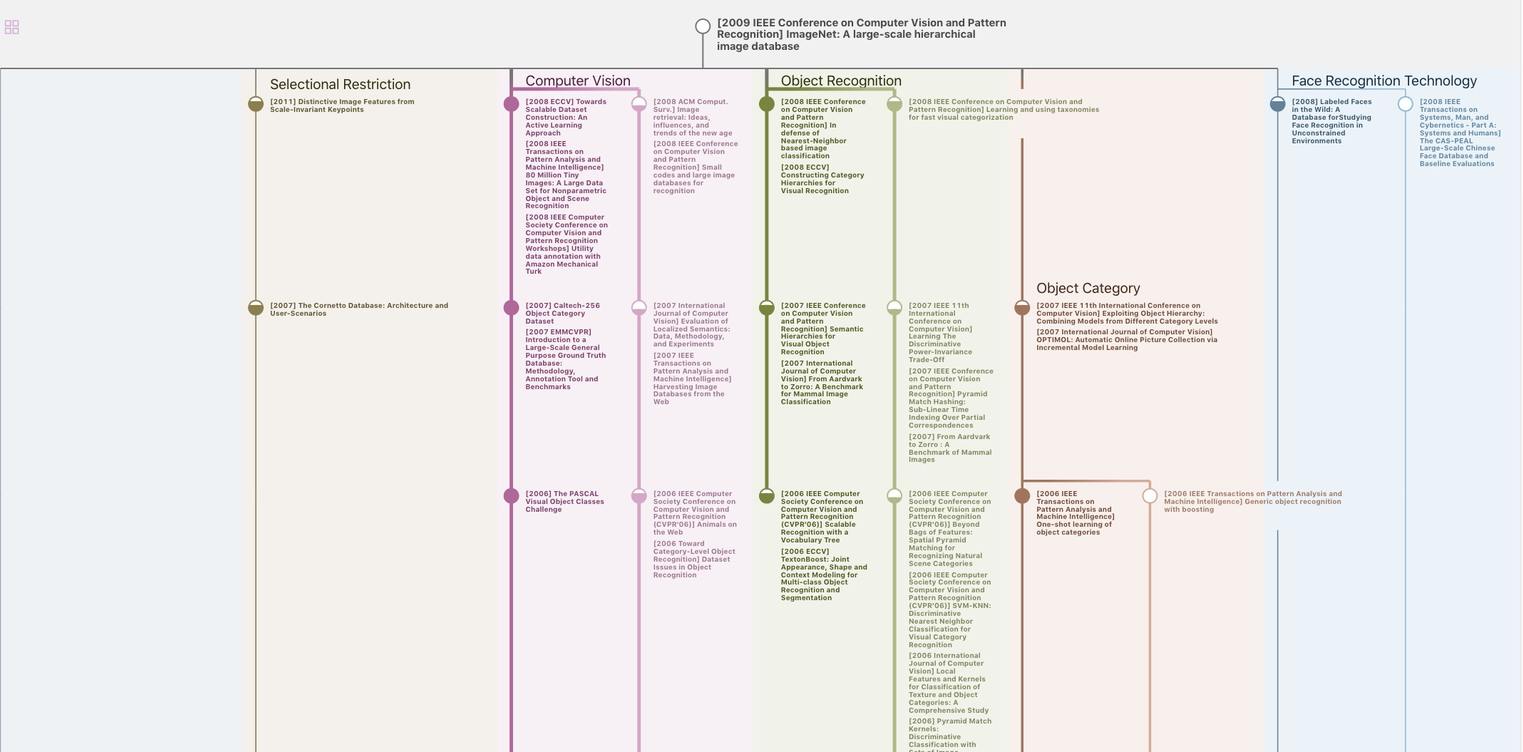
Generate MRT to find the research sequence of this paper
Data Disclaimer
The page data are from open Internet sources, cooperative publishers and automatic analysis results through AI technology. We do not make any commitments and guarantees for the validity, accuracy, correctness, reliability, completeness and timeliness of the page data. If you have any questions, please contact us by email: report@aminer.cn
Chat Paper