STPNet: A Spatial-Temporal Propagation Network for Background Subtraction
IEEE transactions on circuits and systems for video technology(2022)
摘要
In background subtraction tasks, spatial and temporal contexts are beneficial in detecting moving objects. The methods based on Deep Neural Networks in this task has explored different topologies, which are composed of the conventional operations of convolutional neural networks, such as Convolutional Long-short Term Memory layer (ConvLSTM), 2D convolutional layer, or 3D convolutional layer, to capture these contexts. In this work, we propose a new background subtraction algorithm named spatial–temporal propagation network. An end-to-end network with novel layers, whose process of operation is equivalent to that the feature maps multiply with affinity matrices, is proposed to capture the spatial–temporal correlation in video sequences and aggregate the deep features from the consecutive frames. Experimental results on CDnet-2014 and LASIESTA datasets show that this novel layer provides an alternative way for our network to aggregate multiscale spatial–temporal features. Meanwhile, the proposed network achieves state-of-the-art performance and is generalizable to unseen videos.
更多查看译文
关键词
Videos,Feature extraction,Training,Three-dimensional displays,Task analysis,Correlation,Adaptation models,Background subtraction,affinity matrices,convolutional neural network,computer vision
AI 理解论文
溯源树
样例
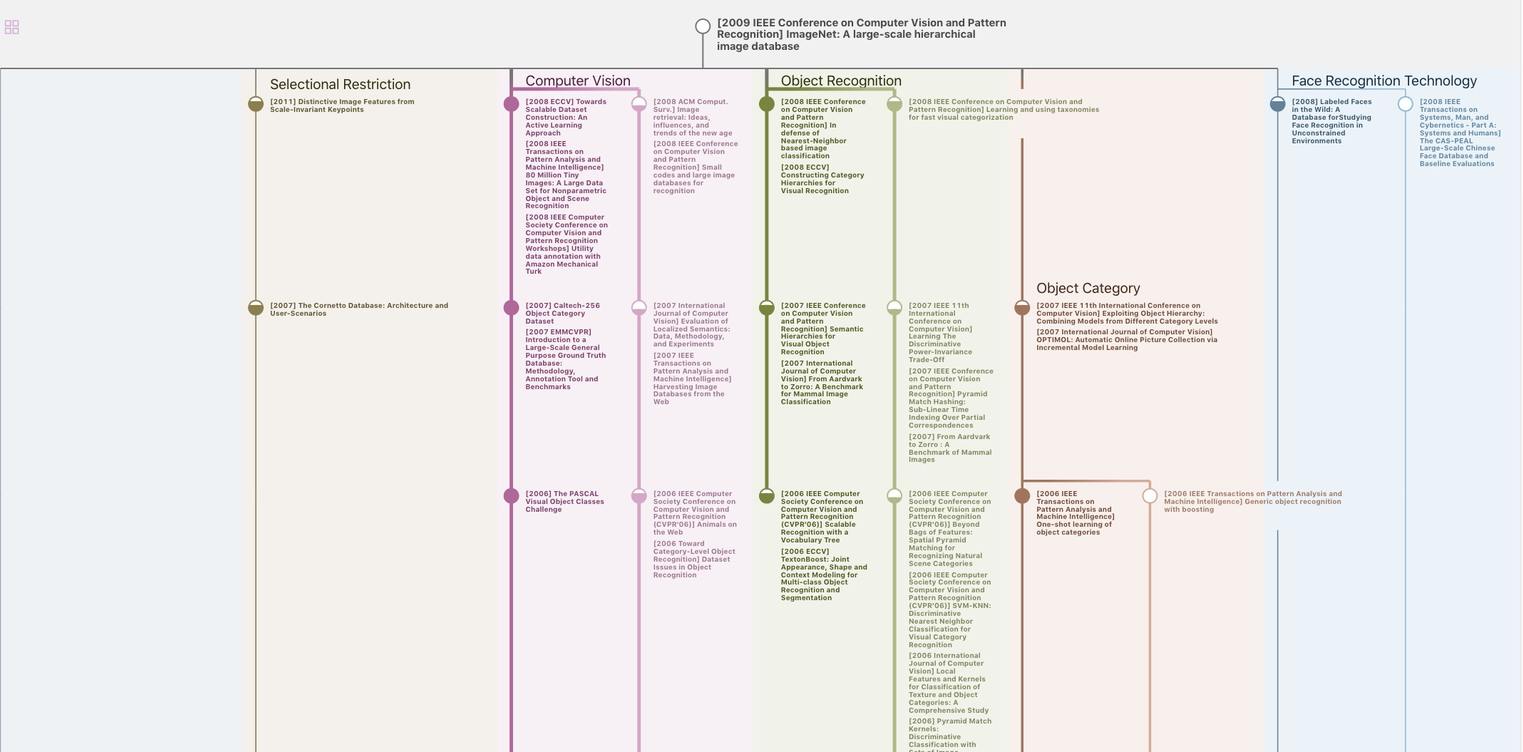
生成溯源树,研究论文发展脉络
Chat Paper
正在生成论文摘要