Statistical Methods for Minimax Estimation in Linear Models with Unknown Design over Finite Alphabets
SIAM journal on mathematics of data science(2022)
摘要
We provide a minimax optimal estimation procedure for F and 1 in matrix valued linear models Y = F 1 + Z, where the parameter matrix 1 and the design matrix F are unknown but the latter takes values in a known finite set. The proposed finite alphabet linear model is justified in a variety of applications, ranging from signal processing to cancer genetics. We show that this allows one to separate F and 1 uniquely under weak identifiability conditions, a task which is not doable, in general. To this end we quantify in the noiseless case, that is, Z = 0, the perturbation range of Y in order to obtain stable recovery of F and 1. Based on this, we derive an iterative Lloyd's type estimation procedure that attains minimax estimation rates for 1 and F for Gaussian error matrix Z. In contrast to the least squares solution the estimation procedure can be computed efficiently and scales linearly with the total number of observations. We confirm our theoretical results in a simulation study and illustrate it with a genetic sequencing data example.
更多查看译文
关键词
Lloyd?s algorithm,combinatorial linear model,minimax theory,exact recovery,blind source sepa-ration,dictionary learning
AI 理解论文
溯源树
样例
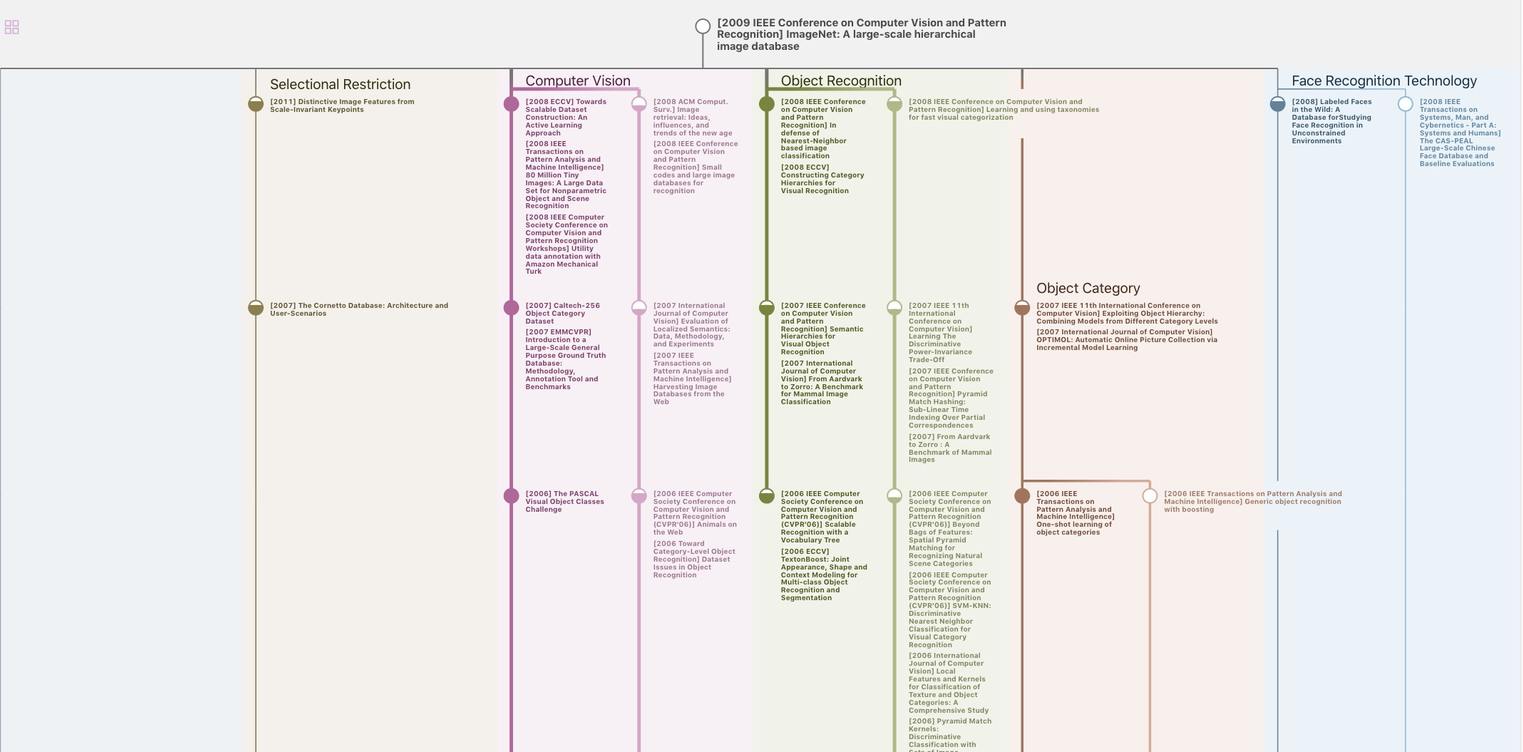
生成溯源树,研究论文发展脉络
Chat Paper
正在生成论文摘要