Wind Turbine Fault Detection: A Semi-Supervised Learning Approach With Automatic Evolutionary Feature Selection
2020 International Conference on Systems, Signals and Image Processing (IWSSIP)(2020)
摘要
Wind energy is promoting the disruption of the current energy model, as the world begins to reduce the use of non-renewable energy sources. At the core of this energetic revolution, the wind turbines are designed to capture the wind's kinetic energy to produce electricity. Wind turbines are complicated and expensive systems whose operating signals are converted into high dimensional data for health management. This signal-to-data conversion involves a variety of constraints that produces an imperfect dataset as an output. In recent years, many semi-supervised learning approaches were developed to detect faults in a wind turbine preemptively. In this paper, we propose a workflow to detect faults based on two hot research topics in data science: (a) automatic feature selection; and (b) semi-supervised classification for fault detection. The multi-objective optimization framework Non-dominated Sorting Genetic Algorithm II (NSGA-II) was adopted to select a subset of features and hyperparameters to perform the semi-supervised fault detection using both a Soft-Label and a Binary support vector machine (SVM). Our experiments were carried out using a dataset containing unlabelled records of 5 2.0MW wind turbines. We discuss the results from NSGA-II based on the trade-off between the number of selected features and the detection performance. We also select the algorithm configuration that achieved the best savings by predicting a faulty system before its collapse.
更多查看译文
关键词
Wind Turbine,Fault detection,Semi-supervised classification
AI 理解论文
溯源树
样例
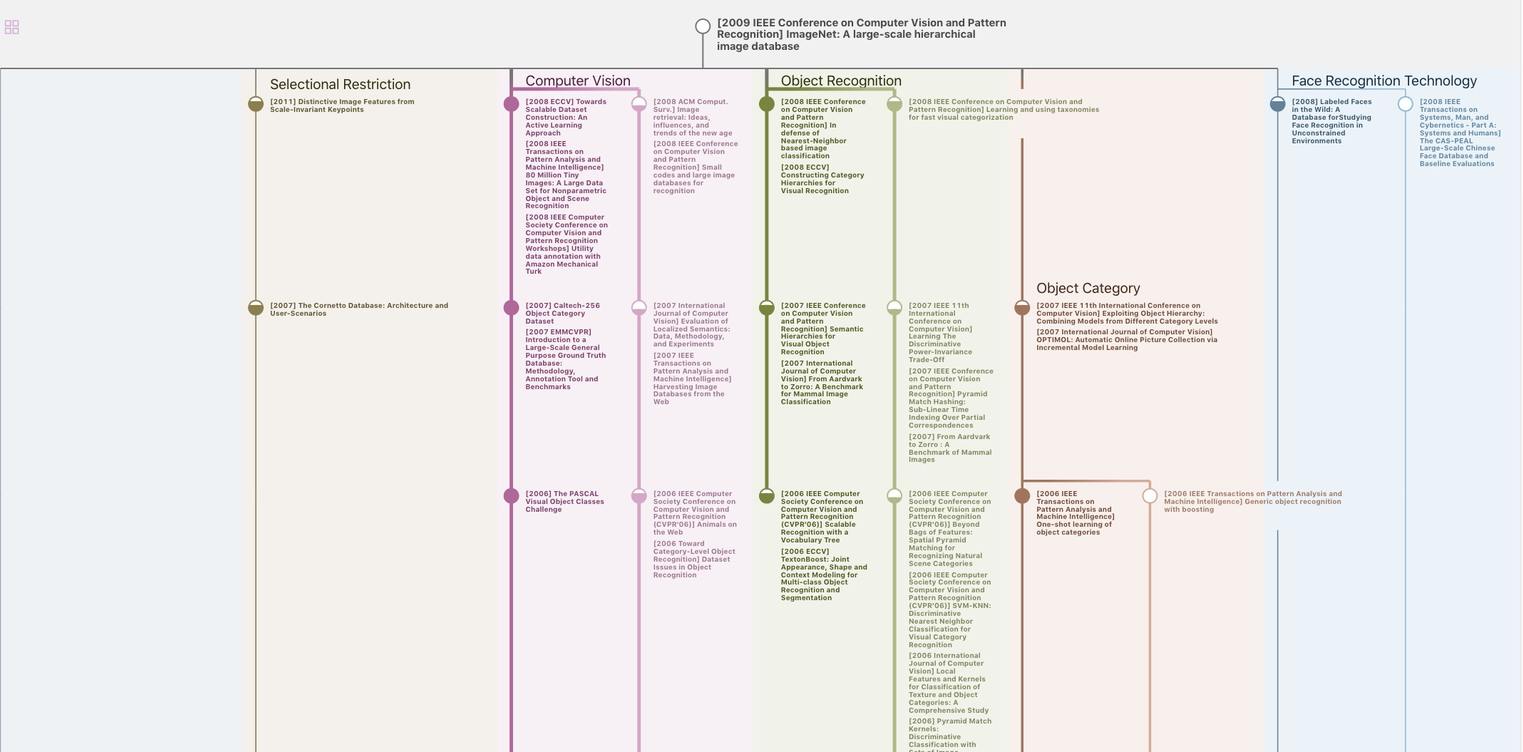
生成溯源树,研究论文发展脉络
Chat Paper
正在生成论文摘要