ESMFL: Efficient and Secure Models for Federated Learning
CoRR(2020)
摘要
Deep Neural Networks are widely applied to various domains. The successful deployment of these applications is everywhere and it depends on the availability of big data. However, massive data collection required for deep neural network reveals the potential privacy issues and also consumes large mounts of communication bandwidth. To address this problem, we propose a privacy-preserving method for the federated learning distributed system, operated on Intel Software Guard Extensions, a set of instructions that increases the security of application code and data. Meanwhile, the encrypted models make the transmission overhead larger. Hence, we reduce the commutation cost by sparsification and achieve reasonable accuracy with different model architectures. Experimental results under our privacy-preserving framework show that, for LeNet-5, we obtain 98.78% accuracy on IID data and 97.60% accuracy on Non-IID data with 34.85% communication saving, and 1.8X total elapsed time acceleration. For MobileNetV2, we obtain 85.40% accuracy on IID data and 81.66% accuracy on Non-IID data with 15.85% communication saving, and 1.2X total elapsed time acceleration.
更多查看译文
关键词
secure models,learning
AI 理解论文
溯源树
样例
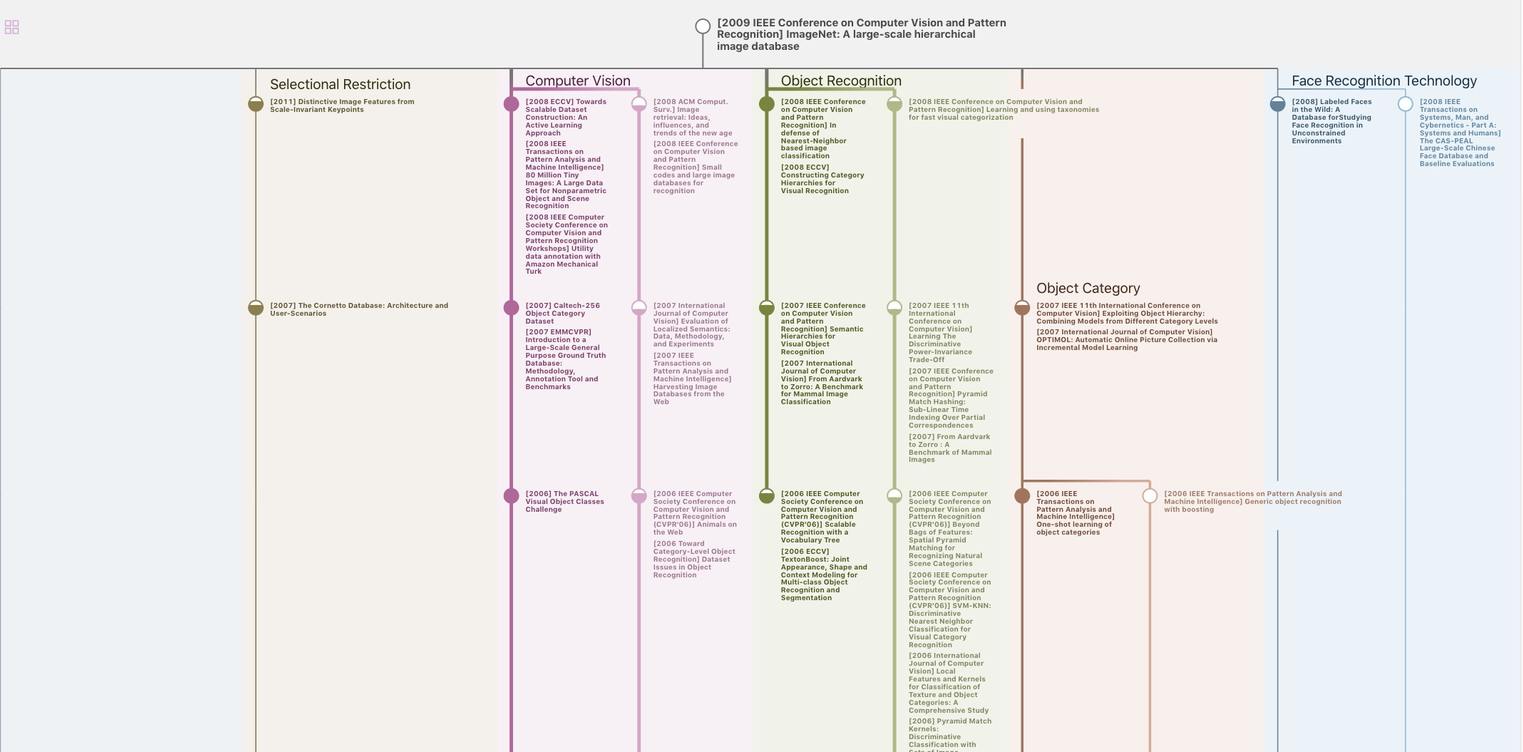
生成溯源树,研究论文发展脉络
Chat Paper
正在生成论文摘要