Deep Learning For Automatic Segmentation Of The Nuclear Envelope In Electron Microscopy Data, Trained With Volunteer Segmentations
TRAFFIC(2021)
摘要
Advancements in volume electron microscopy mean it is now possible to generate thousands of serial images at nanometre resolution overnight, yet the gold standard approach for data analysis remains manual segmentation by an expert microscopist, resulting in a critical research bottleneck. Although some machine learning approaches exist in this domain, we remain far from realizing the aspiration of a highly accurate, yet generic, automated analysis approach, with a major obstacle being lack of sufficient high-quality ground-truth data. To address this, we developed a novel citizen science project, Etch a Cell, to enable volunteers to manually segment the nuclear envelope (NE) of HeLa cells imaged with serial blockface scanning electron microscopy. We present our approach for aggregating multiple volunteer annotations to generate a high-quality consensus segmentation and demonstrate that data produced exclusively by volunteers can be used to train a highly accurate machine learning algorithm for automatic segmentation of the NE, which we share here, in addition to our archived benchmark data.
更多查看译文
关键词
cell biology, cellular imaging, citizen science, image processing, machine learning, segmentation, volume electron microscopy
AI 理解论文
溯源树
样例
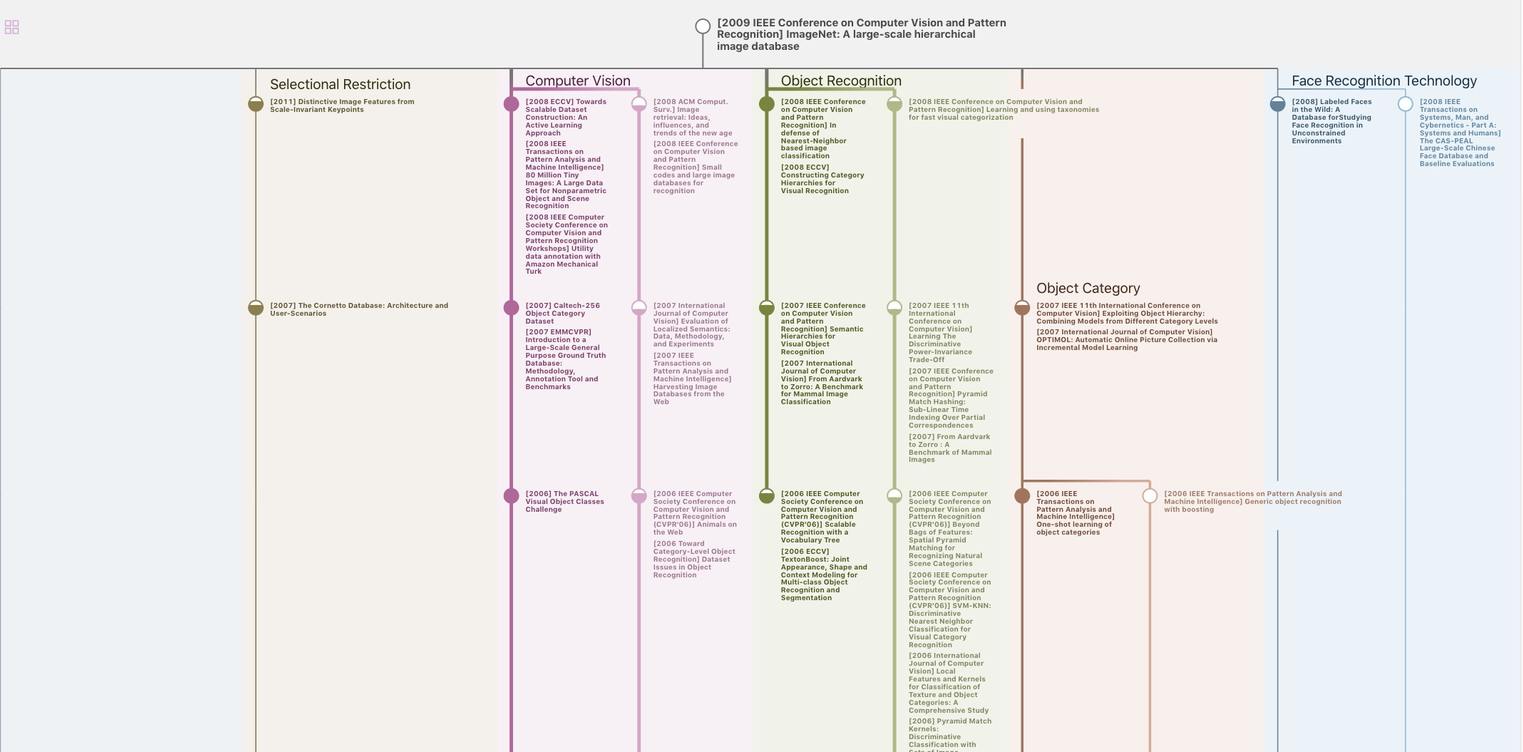
生成溯源树,研究论文发展脉络
Chat Paper
正在生成论文摘要