Unsupervised and Semi-Supervised Few-Shot Acoustic Event Classification
2021 IEEE INTERNATIONAL CONFERENCE ON ACOUSTICS, SPEECH AND SIGNAL PROCESSING (ICASSP 2021)(2021)
摘要
Few-shot Acoustic Event Classification (AEC) aims to learn a model to recognize novel acoustic events using very limited labeled data. Previous works utilize supervised pre-training as well as meta-learning approaches, which heavily rely on labeled data. Here, we study unsupervised and semi-supervised learning approaches for few-shot AEC. Our work builds upon recent advances in unsupervised representation learning introduced for speech recognition and language modeling. We learn audio representations from a large amount of unlabeled data, and use the resulting representations for few-shot AEC. We further extend our model in a semi-supervised fashion. Our unsupervised representation learning approach outperforms supervised pre-training methods, and our semi-supervised learning approach outperforms meta-learning methods for few-shot AEC. We also show that our work is more robust under domain mismatch.
更多查看译文
关键词
acoustic event classification,few-shot learning,representation learning,semi-supervised learning
AI 理解论文
溯源树
样例
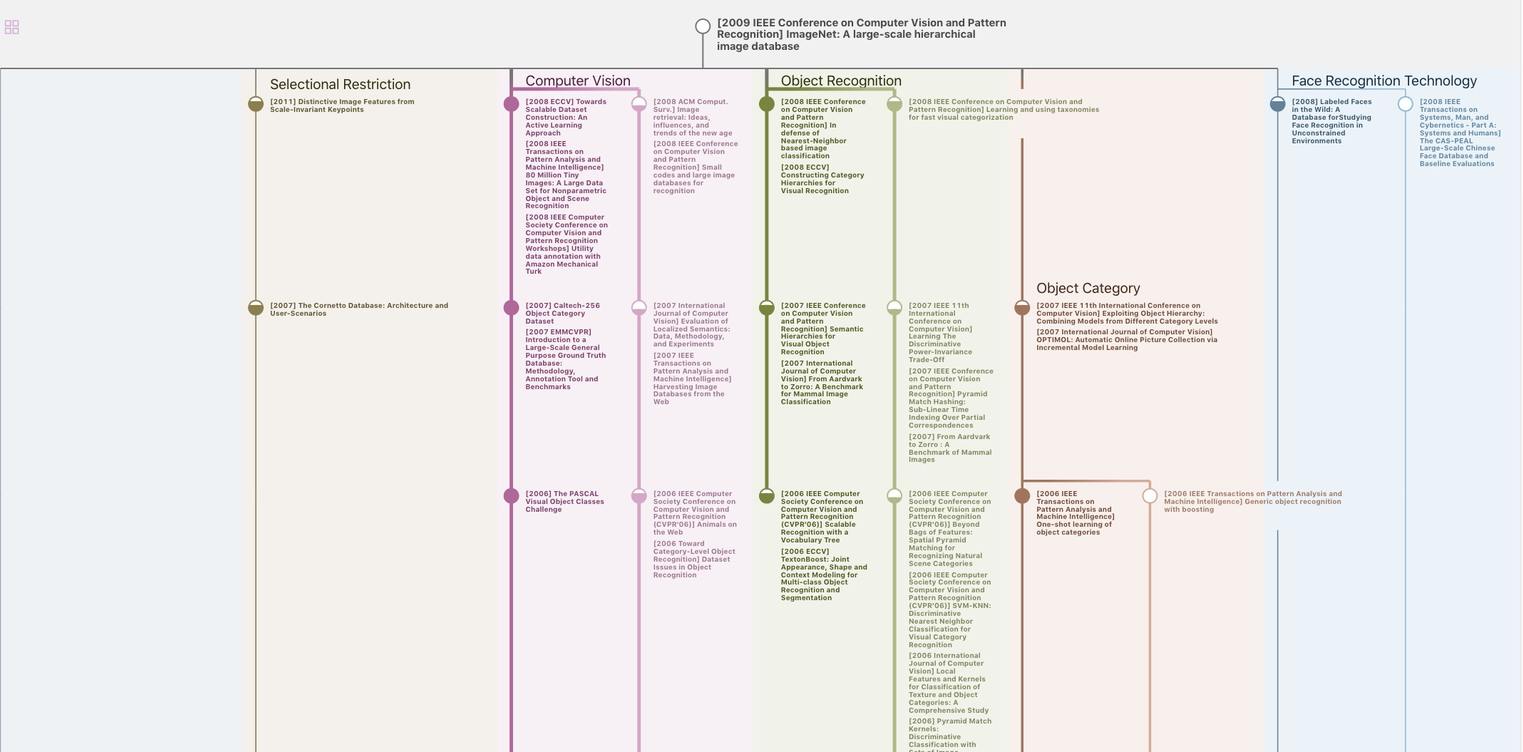
生成溯源树,研究论文发展脉络
Chat Paper
正在生成论文摘要