Backdoor Learning Curves: Explaining Backdoor Poisoning Beyond Influence Functions
INTERNATIONAL JOURNAL OF MACHINE LEARNING AND CYBERNETICS(2024)
摘要
Backdoor attacks inject poisoning samples during training, with the goal of forcing a machine learning model to output an attacker-chosen class when presented a specific trigger at test time. Although backdoor attacks have been demonstrated in a variety of settings and against different models, the factors affecting their effectiveness are still not well understood. In this work, we provide a unifying framework to study the process of backdoor learning under the lens of incremental learning and influence functions. We show that the effectiveness of backdoor attacks depends on: (i) the complexity of the learning algorithm, controlled by its hyperparameters; (ii) the fraction of backdoor samples injected into the training set; and (iii) the size and visibility of the backdoor trigger. These factors affect how fast a model learns to correlate the presence of the backdoor trigger with the target class. Our analysis unveils the intriguing existence of a region in the hyperparameter space in which the accuracy on clean test samples is still high while backdoor attacks are ineffective, thereby suggesting novel criteria to improve existing defenses.
更多查看译文
关键词
Backdoor poisoning,Influence functions,Poisoning,Machine learning,Adversarial machine learning,Security
AI 理解论文
溯源树
样例
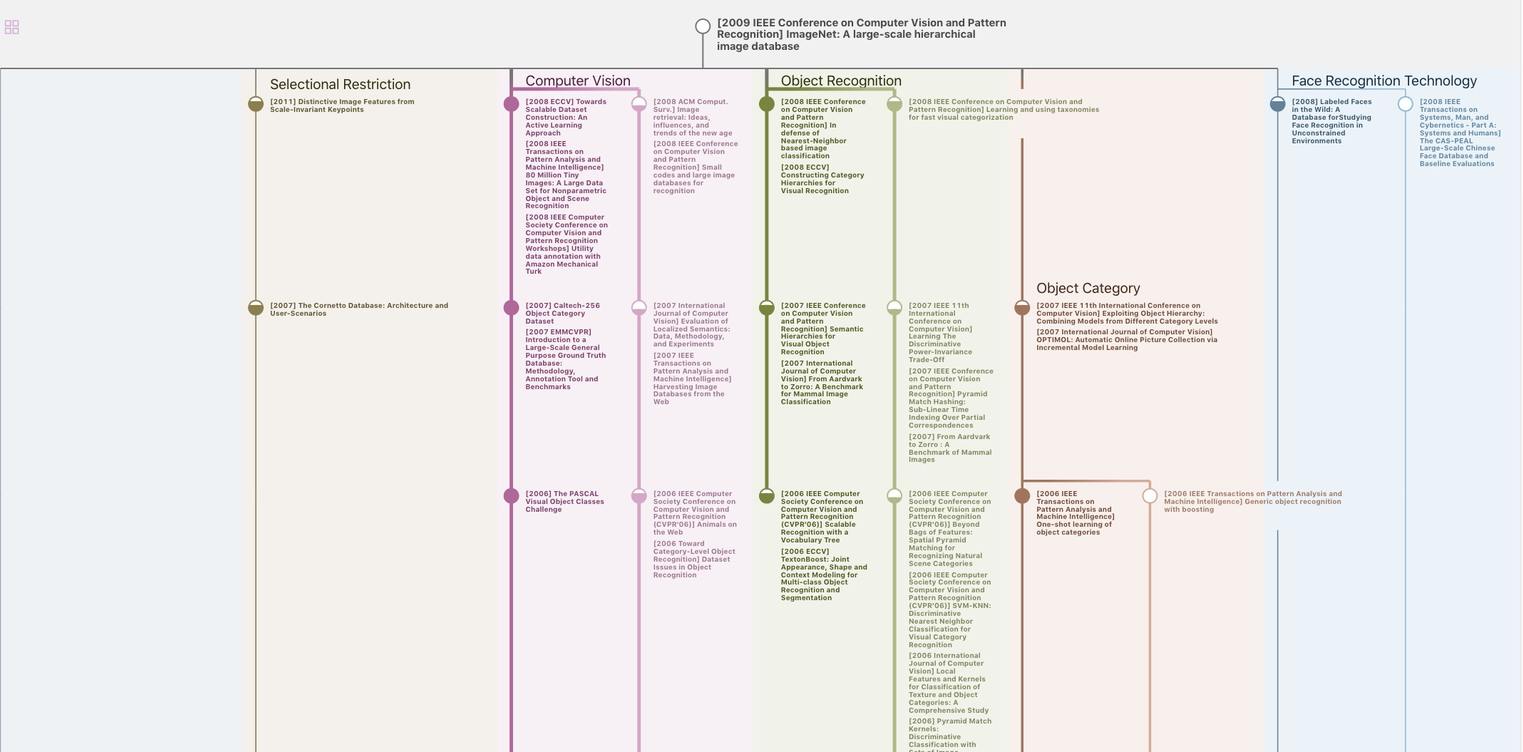
生成溯源树,研究论文发展脉络
Chat Paper
正在生成论文摘要