Privid: Practical, Privacy-Preserving Video Analytics Queries
PROCEEDINGS OF THE 19TH USENIX SYMPOSIUM ON NETWORKED SYSTEMS DESIGN AND IMPLEMENTATION (NSDI '22)(2022)
摘要
Analytics on video recorded by cameras in public areas have the potential to fuel many exciting applications, but also pose the risk of intruding on individuals' privacy. Unfortunately, existing solutions fail to practically resolve this tension between utility and privacy, relying on perfect detection of all private information in each video frame-an elusive requirement. This paper presents: (1) a new notion of differential privacy (DP) for video analytics, (rho, K, epsilon)-event-duration privacy, which protects all private information visible for less than a particular duration, rather than relying on perfect detections of that information, and (2) a practical system called PRIVID that enforces duration-based privacy even with the (untrusted) analyst-provided deep neural networks that are commonplace for video analytics today. Across a variety of videos and queries, we show that PRIVID increases error by 1-21% relative to a non-private system.
更多查看译文
关键词
video,privacy-preserving
AI 理解论文
溯源树
样例
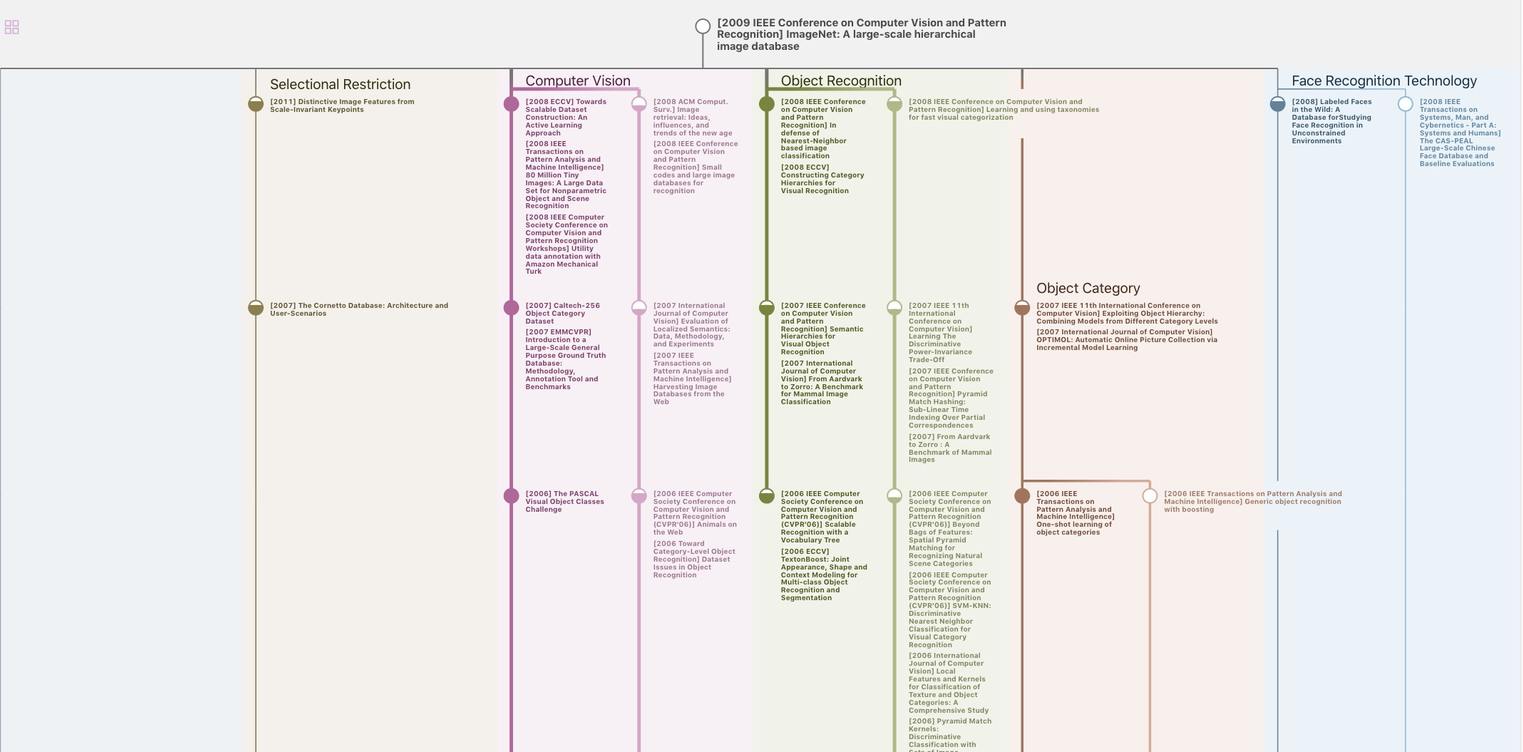
生成溯源树,研究论文发展脉络
Chat Paper
正在生成论文摘要