Predicting in-hospital mortality by combining clinical notes with time-series data.
ACL/IJCNLP(2021)
摘要
In intensive care units (ICUs), patient health is monitored through (1) continuous vital signals from various medical devices, and (2) clinical notes consisting of opinions and summaries from doctors which are recorded in electronic health records (EHR). It is difficult to jointly model these two sources of information because clinical notes, unlike vital signals, are collected at irregular intervals and their contents are relatively unstructured. In this paper, we present a model that combines both sources of information about ICU patients to make accurate in-hospital mortality predictions. We apply a fine-tuned BERT model to each of the patient’s clinical notes. The resulting embeddings are then combined to obtain the overall embedding for the entire text part of the data. This is then combined with the output of an LSTM model that encodes patients’ vital signals. Our model improves upon the state of the art for mortality prediction, attaining an AUC score of 0.9, compared to the previous 0.87, setting a new standard for mortality prediction on the MIMIC III benchmark.1
更多查看译文
关键词
clinical notes,mortality,in-hospital,time-series
AI 理解论文
溯源树
样例
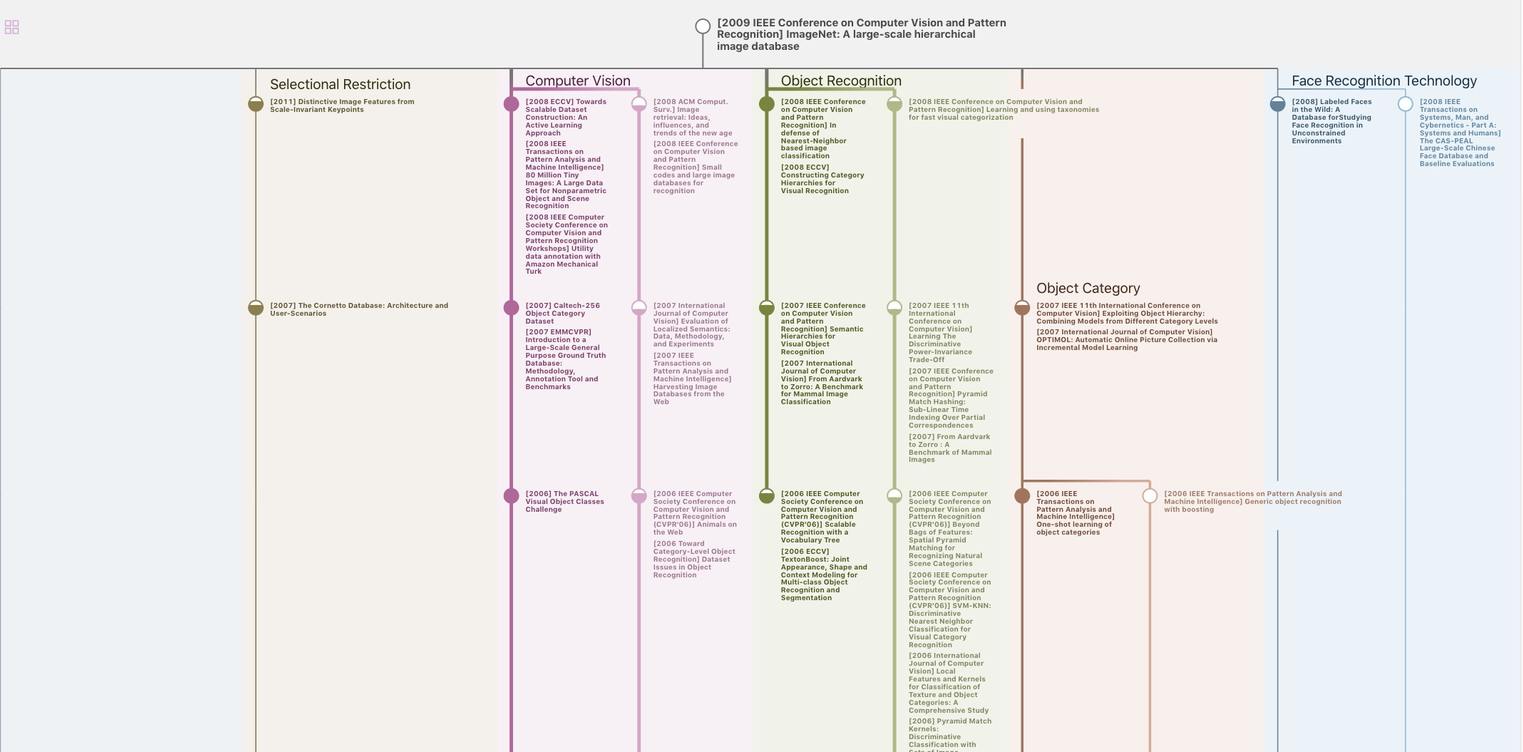
生成溯源树,研究论文发展脉络
Chat Paper
正在生成论文摘要