Overestimation of Syntactic Representationin Neural Language Models.
58TH ANNUAL MEETING OF THE ASSOCIATION FOR COMPUTATIONAL LINGUISTICS (ACL 2020)(2020)
摘要
With the advent of powerful neural language models over the last few years, research attention has increasingly focused on what aspects of language they represent that make them so successful. Several testing methodologies have been developed to probe models’ syntactic representations. One popular method for determining a model’s ability to induce syntactic structure trains a model on strings generated according to a template then tests the model’s ability to distinguish such strings from superficially similar ones with different syntax. We illustrate a fundamental problem with this approach by reproducing positive results from a recent paper with two non-syntactic baseline language models: an n-gram model and an LSTM model trained on scrambled inputs.
更多查看译文
关键词
Language Modeling,Syntax-based Translation Models,Language Understanding,Topic Modeling,Neural Machine Translation
AI 理解论文
溯源树
样例
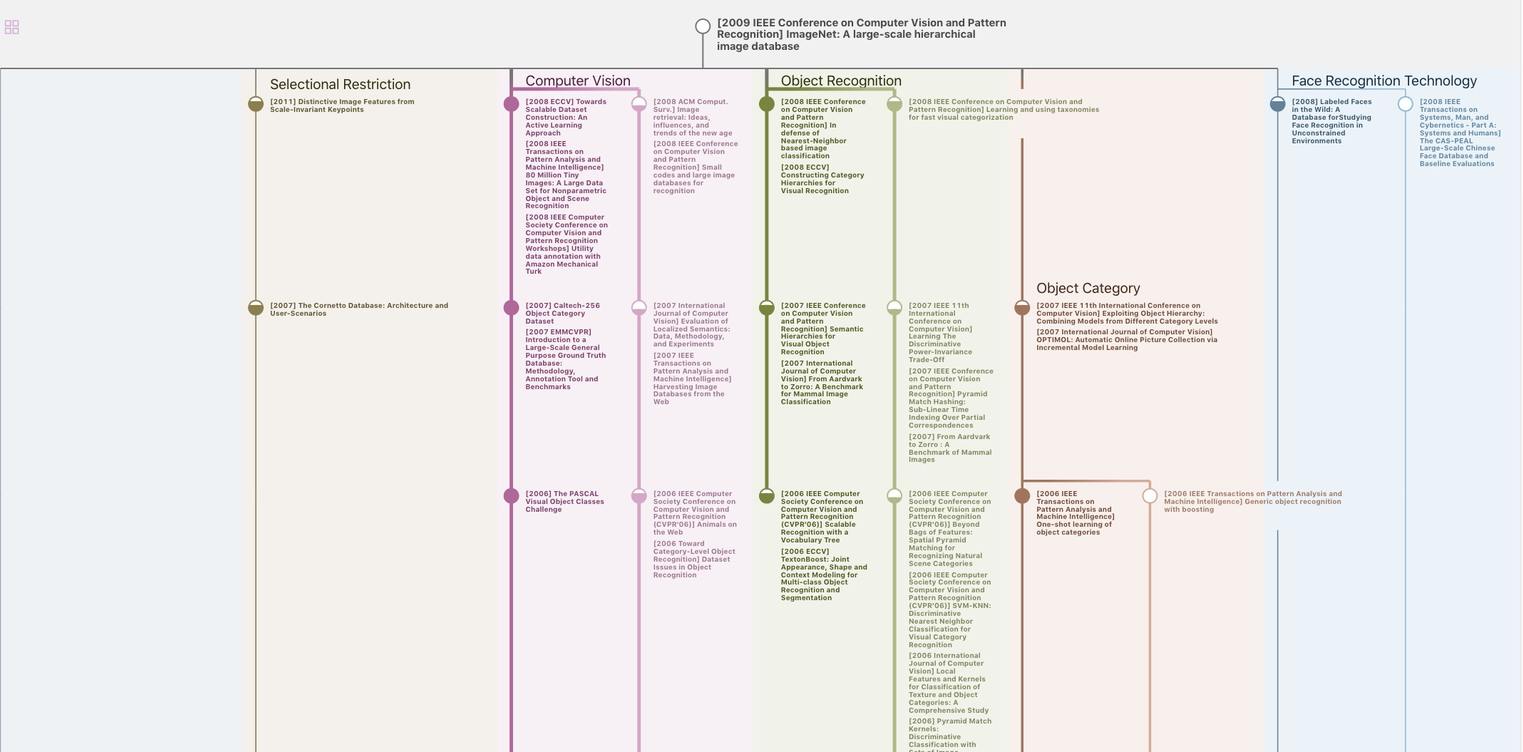
生成溯源树,研究论文发展脉络
Chat Paper
正在生成论文摘要