Multi-output TCN Autoencoder for Long-Term Pollution Forecasting for Multiple Sites
Urban climate(2021)
摘要
Air pollution is one of the major environmental issues attracting massive attention from researchers and policymakers. Both the developed and developing countries are undergoing a high concentration of pollution levels. Fine particulate matter PM2.5 (particles having a diameter less than 2.5μm) and PM10 (particles having a diameter less than 10μm) can easily penetrate the lungs and respiratory system and causes adverse health issues like heart attacks, cardiovascular diseases, lung function reduction. Real-time pollutant information is of great importance to providing prompt and complete information on air quality. Air pollution forecasting is another significant step of air pollution management, which can help policymakers and citizens make proper decisions to prevent air pollution-related diseases. This research study explores a novel pollutant forecasting model named as Multi-output temporal convolutional network autoencoder (MO-TCNA). The model accumulates each step's predicted values to perform multi-step ahead long-term forecasting for multiple pollutants and multiple sites in a single training model. The MO-TCNA network serves both the PM2.5 and PM10 pollutants forecasting for various locations instead of performing single output and site-specific pollutant forecasting. Consequently, the experimental results show that the MO-TCNA network is time-saving and has better performance than the traditional site-specific forecasting models.
更多查看译文
关键词
Temporal convolutional network,Spatio-temporal prediction,Autoencoder,Deep learning,Pollution
AI 理解论文
溯源树
样例
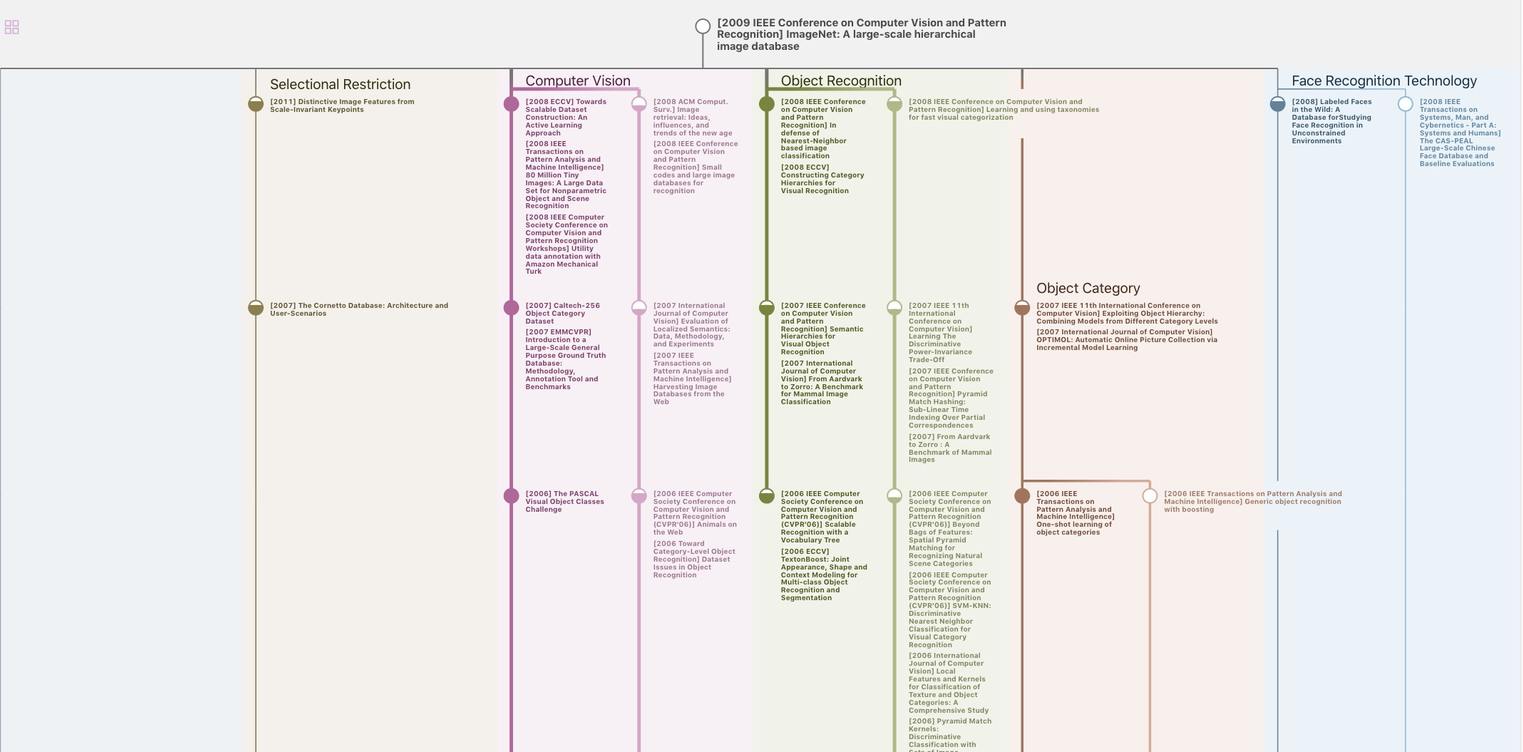
生成溯源树,研究论文发展脉络
Chat Paper
正在生成论文摘要