From Server-Based to Client-Based Machine Learning: A Comprehensive Survey
ACM COMPUTING SURVEYS(2021)
摘要
In recent years, mobile devices have gained increasing development with stronger computation capability and larger storage space. Some of the computation-intensive machine learning tasks can now be run on mobile devices. To exploit the resources available on mobile devices and preserve personal privacy, the concept of client-based machine learning has been proposed. It leverages the users' local hardware and local data to solve machine learning sub-problems on mobile devices and only uploads computation results rather than the original data for the optimization of the global model. Such an architecture can not only relieve computation and storage burdens on servers but also protect the users' sensitive information. Another benefit is the bandwidth reduction because various kinds of local data can be involved in the training process without being uploaded. In this article, we provide a literature review on the progressive development of machine learning from server based to client based. We revisit a number of widely used server-based and client-based machine learning methods and applications. We also extensively discuss the challenges and future directions in this area. We believe that this survey will give a clear overview of client-based machine learning and provide guidelines on applying client-based machine learning to practice.
更多查看译文
关键词
Mobile intelligence,machine learning,distributed system,decentralized training,federated learning
AI 理解论文
溯源树
样例
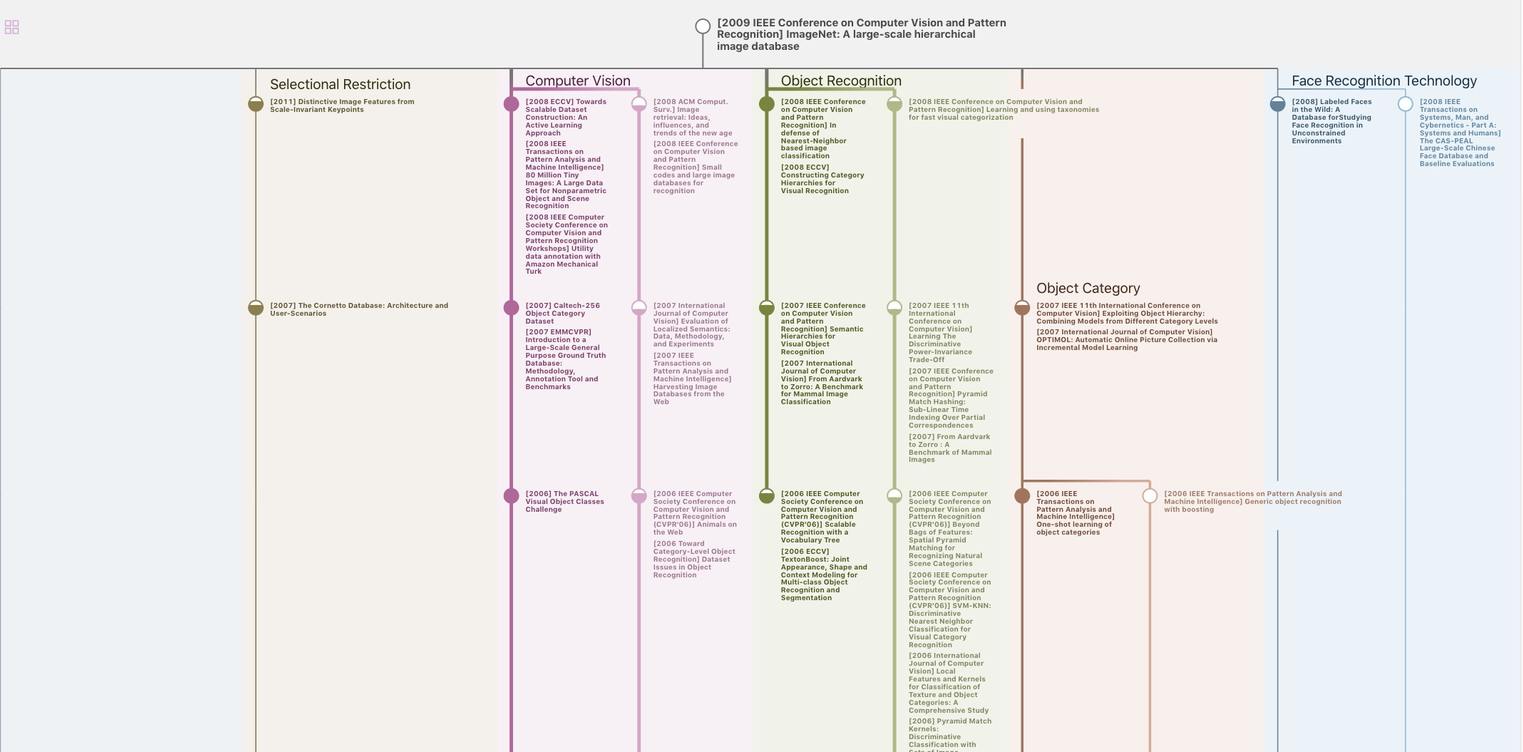
生成溯源树,研究论文发展脉络
Chat Paper
正在生成论文摘要