Domain Knowledge-Based Automated Analog Circuit Design with Deep Reinforcement Learning
arXiv (Cornell University)(2022)
摘要
The design automation of analog circuits is a longstanding challenge in the integrated circuit field. This paper presents a deep reinforcement learning method to expedite the design of analog circuits at the pre-layout stage, where the goal is to find device parameters to fulfill desired circuit specifications. Our approach is inspired by experienced human designers who rely on domain knowledge of analog circuit design (e.g., circuit topology and couplings between circuit specifications) to tackle the problem. Unlike all prior methods, our method originally incorporates such key domain knowledge into policy learning with a graph-based policy network, thereby best modeling the relations between circuit parameters and design targets. Experimental results on exemplary circuits show it achieves human-level design accuracy (~99%) with 1.5x efficiency of existing best-performing methods. Our method also shows better generalization ability to unseen specifications and optimality in circuit performance optimization. Moreover, it applies to designing diverse analog circuits across different semiconductor technologies, breaking the limitations of prior ad-hoc methods in designing one particular type of analog circuits with conventional semiconductor technology.
更多查看译文
关键词
automated analog circuit design,learning,knowledge-based
AI 理解论文
溯源树
样例
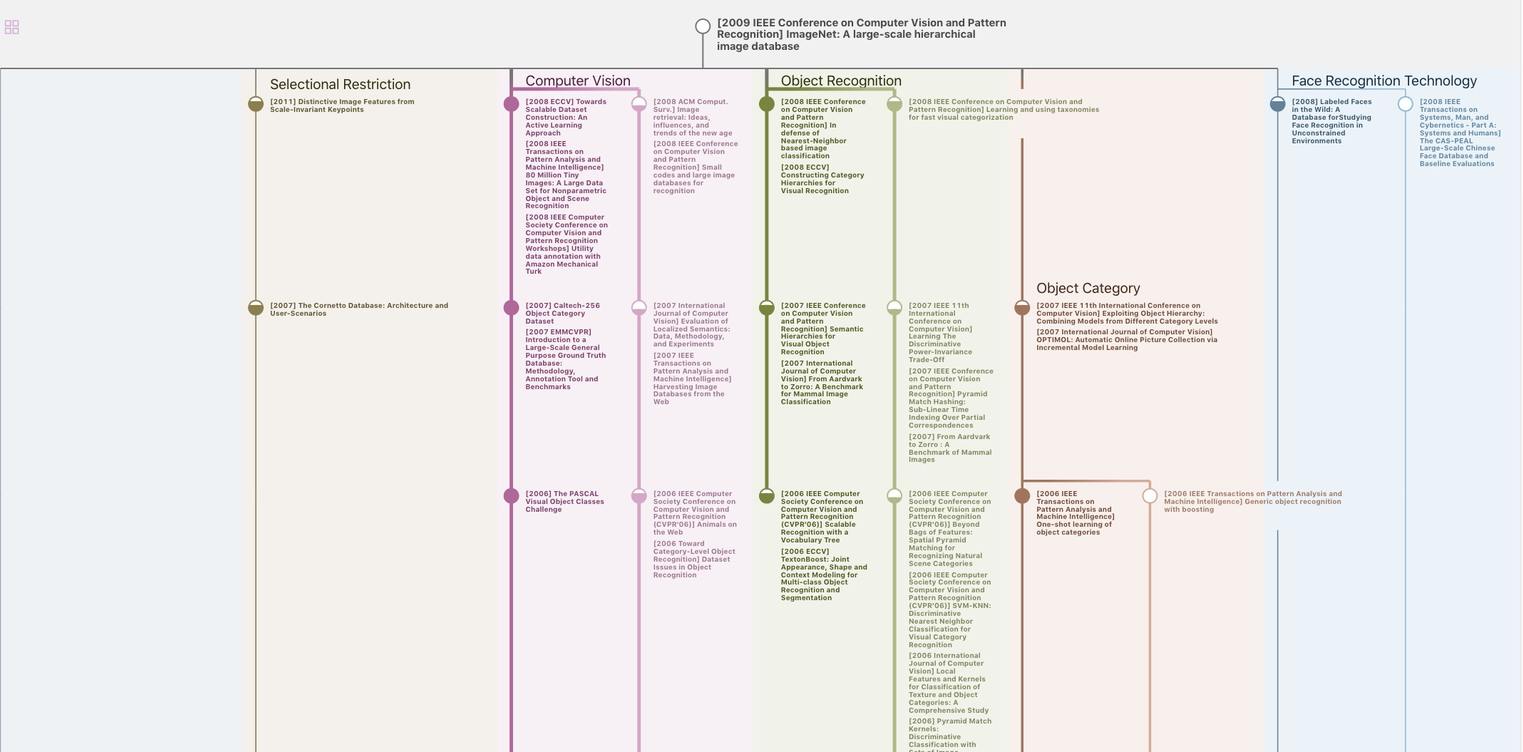
生成溯源树,研究论文发展脉络
Chat Paper
正在生成论文摘要