Knowledge Embedding Based Graph Convolutional Network
PROCEEDINGS OF THE WORLD WIDE WEB CONFERENCE 2021 (WWW 2021)(2021)
摘要
Recently, a considerable literature has grown up around the theme of Graph Convolutional Network (GCN). How to effectively leverage the rich structural information in complex graphs, such as knowledge graphs with heterogeneous types of entities and relations, is a primary open challenge in the field. Most GCN methods are either restricted to graphs with a homogeneous type of edges (e.g., citation links only), or focusing on representation learning for nodes only instead of jointly propagating and updating the embeddings of both nodes and edges for target-driven objectives. This paper addresses these limitations by proposing a novel framework, namely the Knowledge Embedding based Graph Convolutional Network (KE-GCN), which combines the power of GCNs in graph-based belief propagation and the strengths of advanced knowledge embedding (a.k.a. knowledge graph embedding) methods, and goes beyond. Our theoretical analysis shows that KE-GCN offers an elegant unification of several well-known GCN methods as specific cases, with a new perspective of graph convolution. Experimental results on benchmark datasets show the advantageous performance of KE-GCN over strong baseline methods in the tasks of knowledge graph alignment and entity classification(1).
更多查看译文
关键词
graph convolutional network,knowledge graph,knowledge embedding
AI 理解论文
溯源树
样例
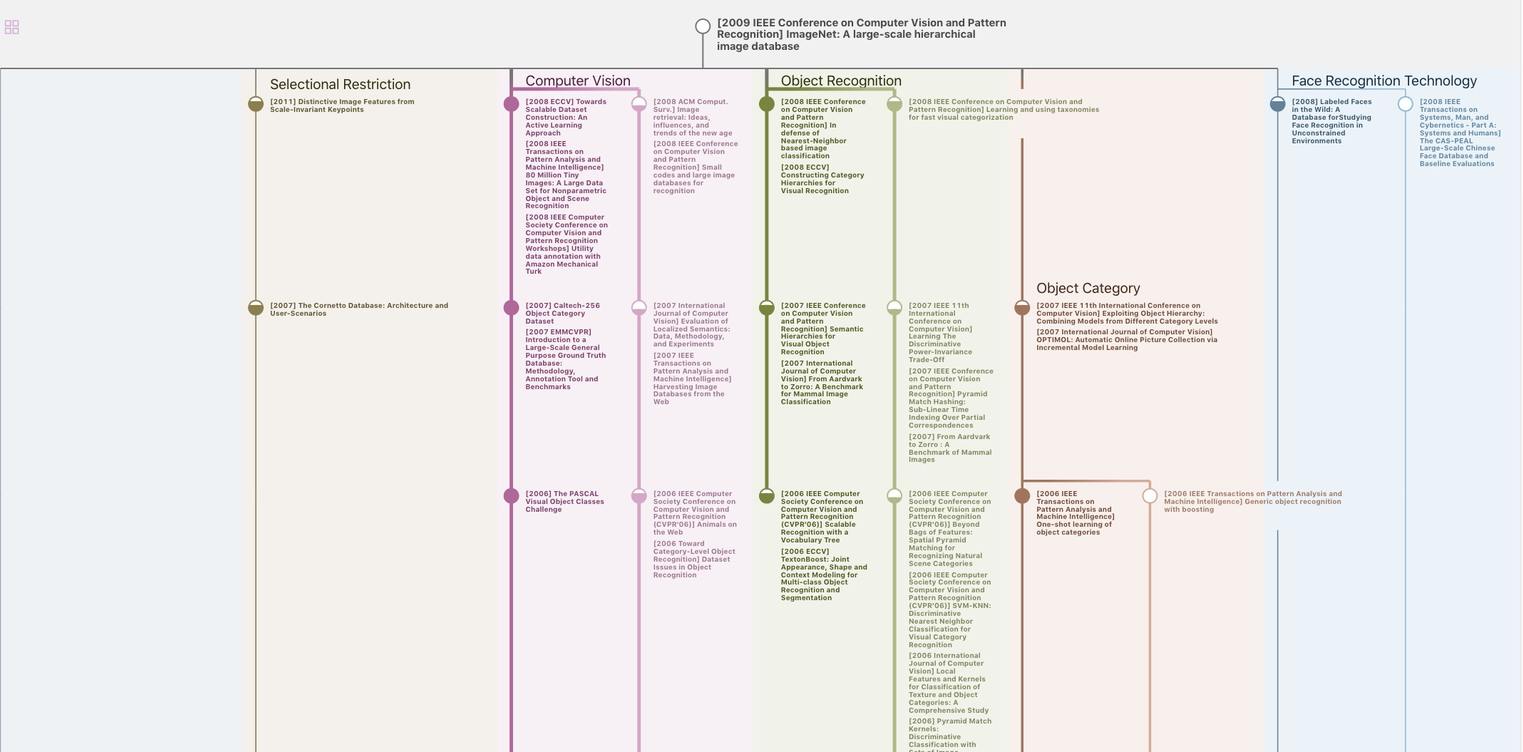
生成溯源树,研究论文发展脉络
Chat Paper
正在生成论文摘要