Error-Driven Fixed-Budget ASR Personalization for Accented Speakers
arXiv (Cornell University)(2021)
摘要
We consider the task of personalizing ASR models while being constrained by a fixed budget on recording speaker specific utterances. Given a speaker and an ASR model, we propose a method of identifying sentences for which the speaker’s utterances are likely to be harder for the given ASR model to recognize. We assume a tiny amount of speaker-specific data to learn phoneme-level error models which help us select such sentences. We show that speaker’s utterances on the sentences selected using our error model indeed have larger error rates when compared to speaker’s utterances on randomly selected sentences. We find that fine-tuning the ASR model on the sentence utterances selected with the help of error models yield higher WER improvements in comparison to fine-tuning on an equal number of randomly selected sentence utterances. Thus, our method provides an efficient way of collecting speaker utterances under budget constraints for personalizing ASR models.
更多查看译文
关键词
Data selection,Personalization,Accent-adaptation,Error detection,Speaker-adaptation
AI 理解论文
溯源树
样例
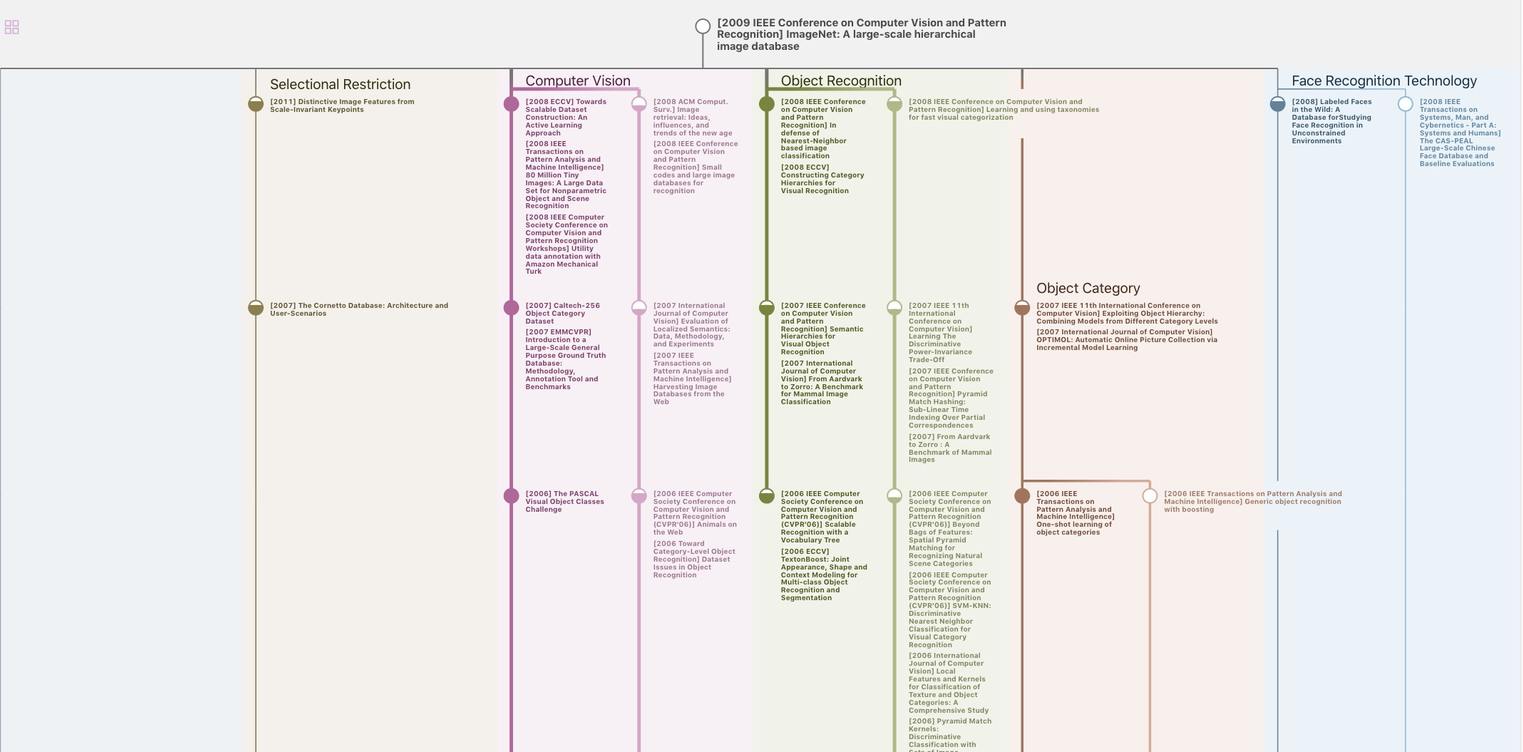
生成溯源树,研究论文发展脉络
Chat Paper
正在生成论文摘要