Assessing Scale‐dependent Effects on Forest Biomass Productivity Based on Machine Learning
Ecology and evolution(2022)
摘要
AbstractEstimating forest above‐ground biomass (AGB) productivity constitutes one of the most fundamental topics in forest ecological research. Based on a 30‐ha permanent field plot in Northeastern China, we modeled AGB productivity as output, and topography, species diversity, stand structure, and a stand density variable as input across a series of area scales using the Random Forest (RF) algorithm. As the grain size increased from 10 to 200 m, we found that the relative importance of explanatory variables that drove the variation of biomass productivity varied a lot, and the model accuracy was gradually improved. The minimum sampling area for biomass productivity modeling in this region was 140 × 140 m. Our study shows that the relationship of topography, species diversity, stand structure, and stand density variables with biomass productivity modeled using the RF algorithm changes when moving from scales typical of forest surveys (10 m) to larger scales (200 m) within a controlled methodology. These results should be of considerable interest to scientists concerned with forest assessment.
更多查看译文
关键词
above-ground biomass,productivity,random Forest algorithm,random spatial sampling,scale dependence
AI 理解论文
溯源树
样例
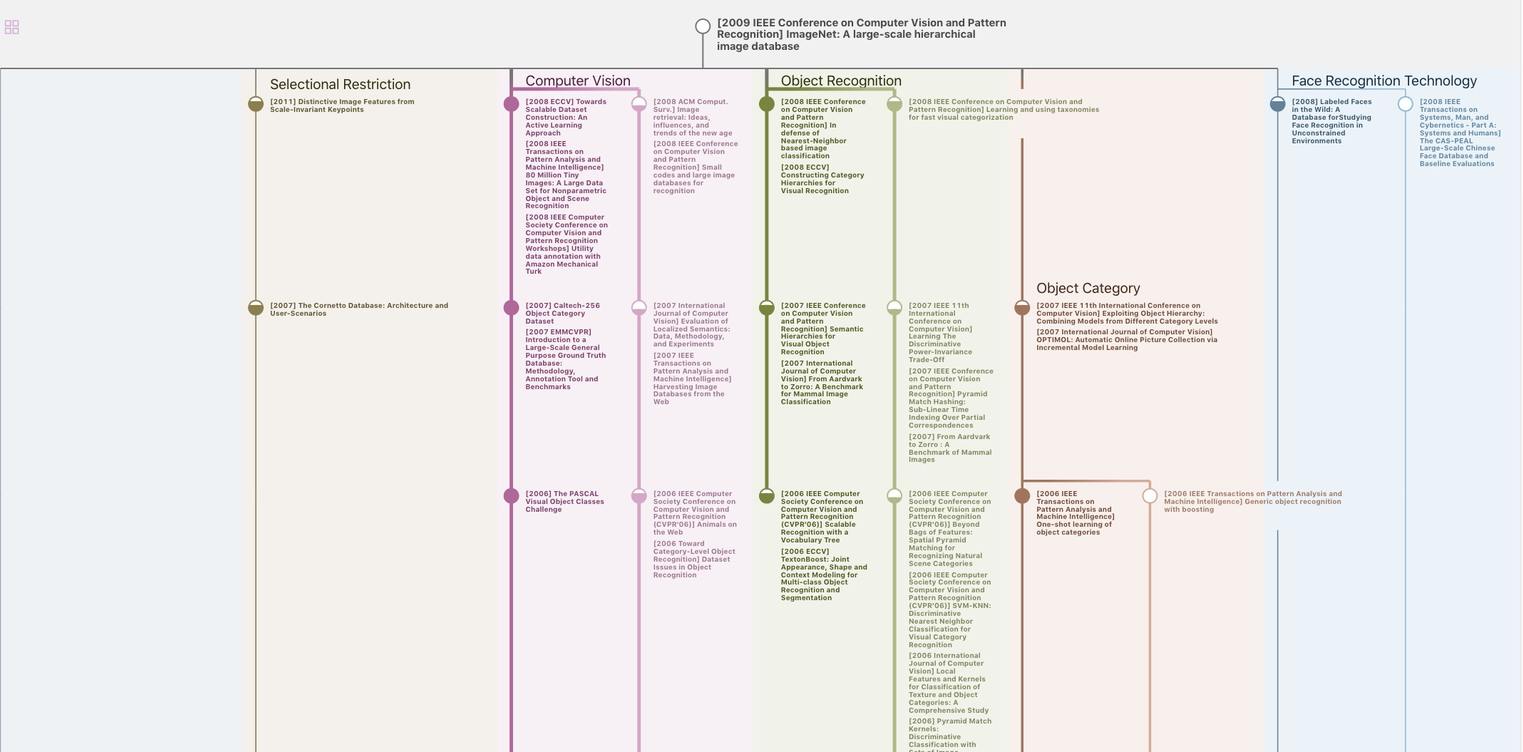
生成溯源树,研究论文发展脉络
Chat Paper
正在生成论文摘要