A Comprehensive Analysis of the Diverse Aspects Inherent to Image Data Stream Classification
Knowledge and information systems(2022)
摘要
Image data stream classification presents several challenges, for example, the evolution of concepts of known classes (concept drift) and the emergence of new classes (open set). Many studies conducted on image data stream classification investigate the classifier, but do not explore other important issues, such as specific evaluation methods for data stream scenarios, evolution of the image feature descriptor and the updating of the decision model, while considering characteristics of real application environments. This article thus aims at making contributions that aid in closing these gaps through the incorporation of an experimental study, which considers a new evaluation method for the classification of image streams, while deliberating on important issues connected to this task. To this end, algorithms from the literature were considered, in order to identify how such algorithms lose performance when evaluated in real-world scenarios. Experiments were carried out exploring the refinement of the feature descriptor, updating the model in the presence of concept drift and open set, in addition to the use of latency and active learning strategies. The results obtained show that the greater the reality considered in the experiments, the greater the degradation of the results.
更多查看译文
关键词
Feature evolution,Image classification,Image data stream,Image data stream evaluation
AI 理解论文
溯源树
样例
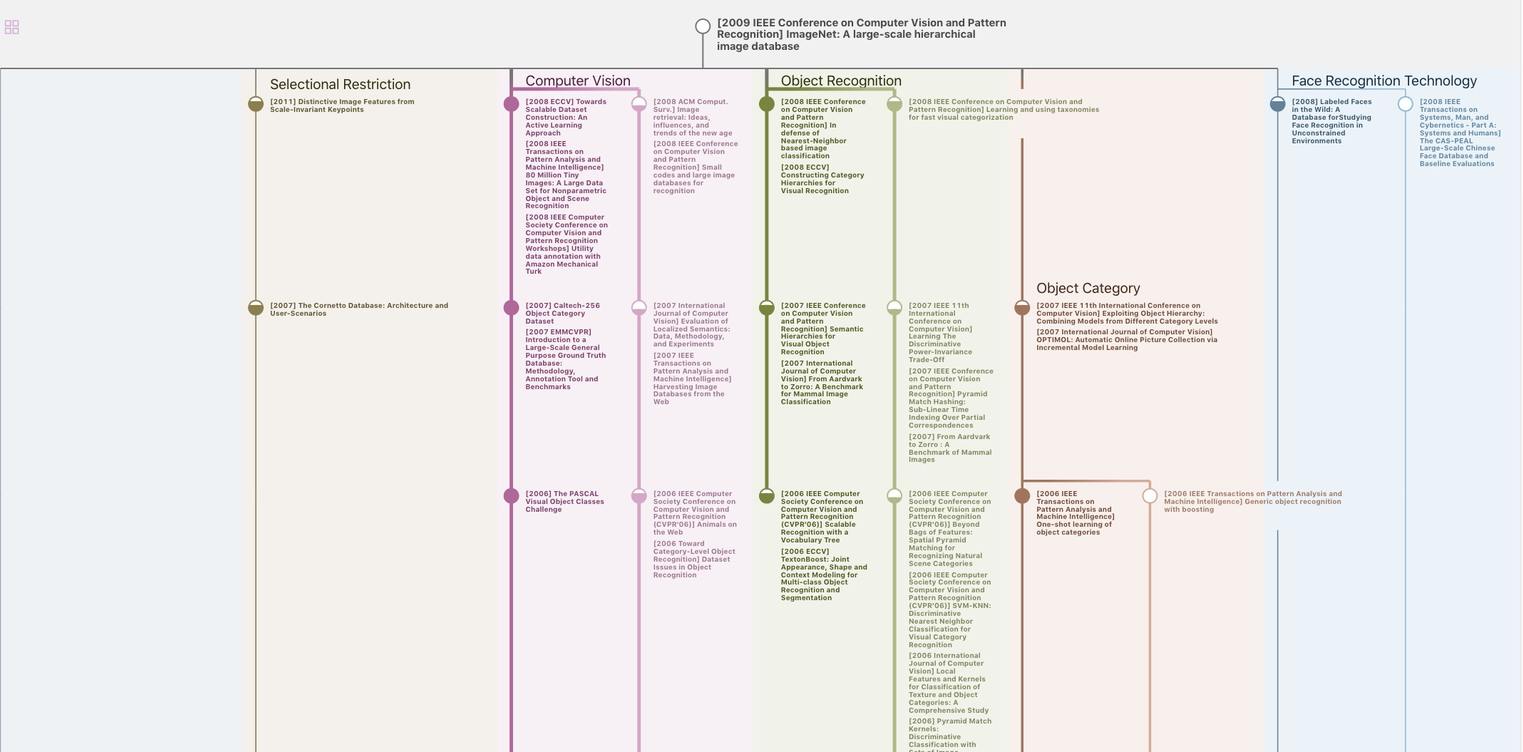
生成溯源树,研究论文发展脉络
Chat Paper
正在生成论文摘要