Denoising Particle Beam Micrographs With Plug-and-Play Methods
IEEE Transactions on Computational Imaging(2023)
摘要
In a particle beam microscope, a raster-scanned focused beam of particles interacts with a sample to generate a secondary electron (SE) signal pixel by pixel. Conventionally formed micrographs are noisy because of limitations on acquisition time and dose. Recent work has shown that estimation methods applicable to a time-resolved measurement paradigm can greatly reduce noise, but these methods apply pixel by pixel without exploiting image structure. Raw SE count data can be modeled with a compound Poisson (Neyman Type A) likelihood, which implies data variance that is signal-dependent and greater than the variation in the underlying particle-sample interaction. These statistical properties make methods that assume additive white Gaussian noise ineffective. This article introduces methods for particle beam micrograph denoising that use the plug-and-play framework to exploit image structure while being applicable to the unusual data likelihoods of this modality. Approximations of the data likelihood that vary in accuracy and computational complexity are combined with denoising by total variation regularization, BM3D, and DnCNN. Methods are provided for both conventional and time-resolved measurements, assuming SE counts are available. In simulations representative of helium ion microscopy and scanning electron microscopy, significant improvements in root mean-squared error (RMSE), structural similarity index measure (SSIM), and qualitative appearance are obtained. Average reductions in RMSE are by factors ranging from 2.24 to 4.11.
更多查看译文
关键词
Electron microscopy,focused ion beam,helium ion microscopy,Neyman Type A distribution,Poisson processes
AI 理解论文
溯源树
样例
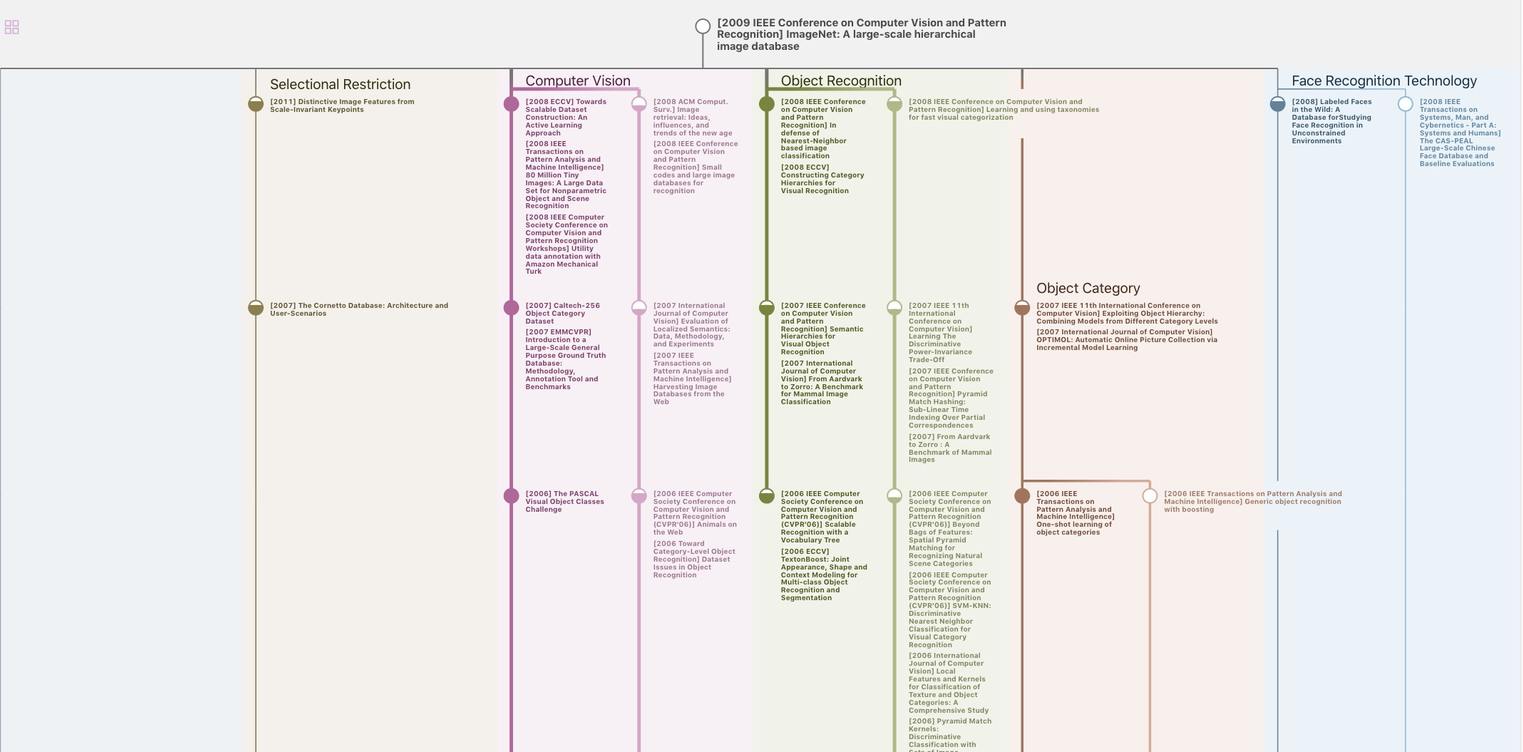
生成溯源树,研究论文发展脉络
Chat Paper
正在生成论文摘要