Analysis of Relaxation Methods for Feature Selection in Mixed Effects Models
arxiv(2022)
摘要
Linear Mixed-Effects (LME) models are a fundamental tool for modeling clustered data, including cohort studies, longitudinal data analysis, and meta-analysis. The design and analysis of variable selection methods for LMEs is considerably more difficult than for linear regression because LME models are nonlinear. The approach considered here is motivated by a recent method for sparse relaxed regularized regression (SR3) for variable selection in the context of linear regression. The theoretical underpinnings for the proposed extension to LMEs are developed, including consistency results, variational properties, implementability of optimization methods, and convergence results. In particular we provide convergence analyses for a basic implementation of SR3 for LME (called MSR3) and an accelerated hybrid algorithm (called MSR3-fast). Numerical results show the utility and speed of these algorithms on realistic simulated datasets. The numerical implementations are available in an open source python package pysr3.
更多查看译文
AI 理解论文
溯源树
样例
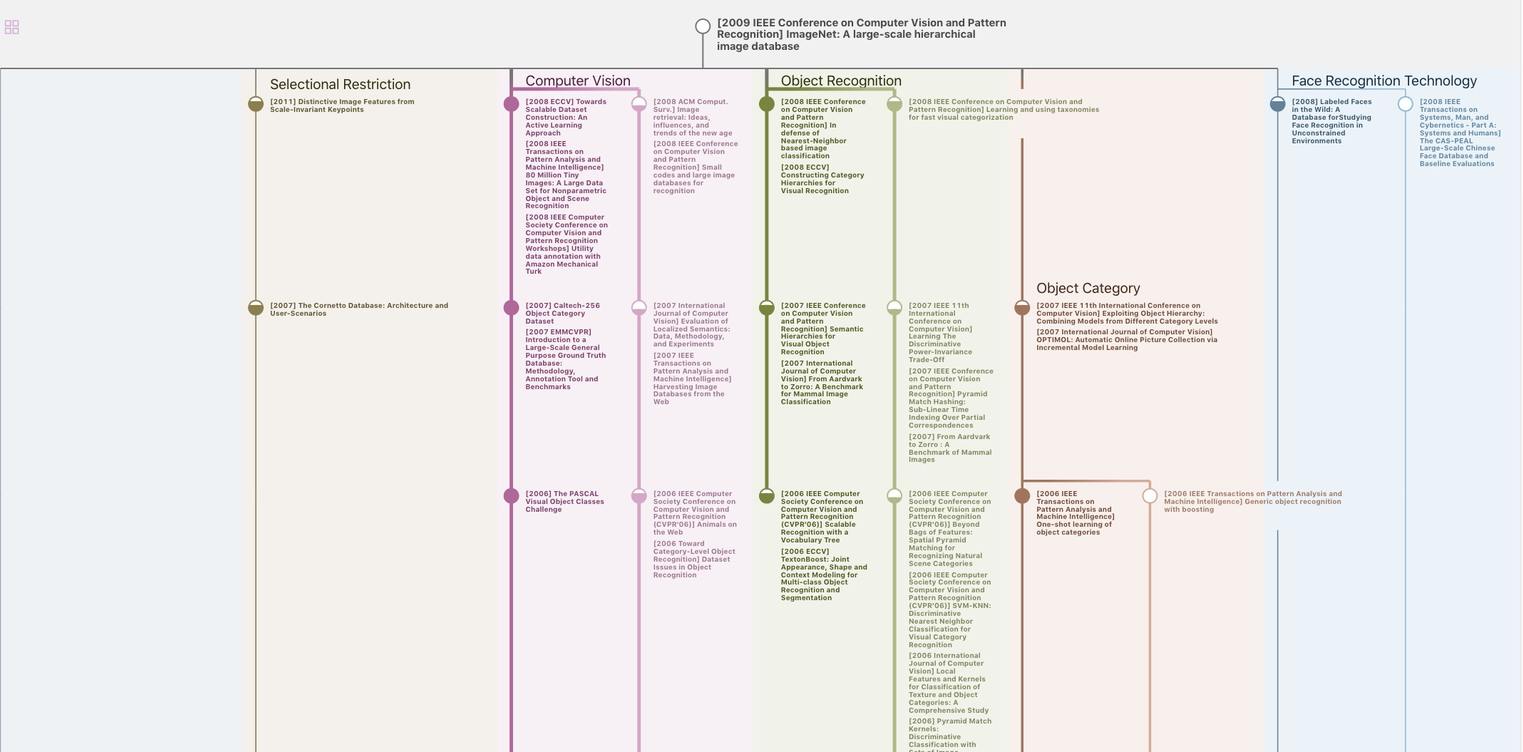
生成溯源树,研究论文发展脉络
Chat Paper
正在生成论文摘要